Comparison of imputation methods for discriminant analysis with strategically hidden data
European Journal of Operational Research(2016)
摘要
Abstract In many situations, data may be selectively presented by data providers to achieve desirable but undeserved decision outcomes from decision makers. Decisions taken without considering strategic information revelation might be biased. We revisit and study the properties of two methods handling strategically missing data in a classification context. The asymptotic analysis suggests that when the training sets are sufficiently large these methods outperform the conventional methods handling missing data that do not consider strategic motivations of agents (e.g., Average method and Similarity method). Scale-up experiments support the theoretical findings and show that as the training size increases the misclassification rates of those methods decrease. We show that sampling can be used to efficiently identify sufficient information for the imputation methods to treat strategically missing data.
更多查看译文
关键词
imputation methods,discriminant analysis,data
AI 理解论文
溯源树
样例
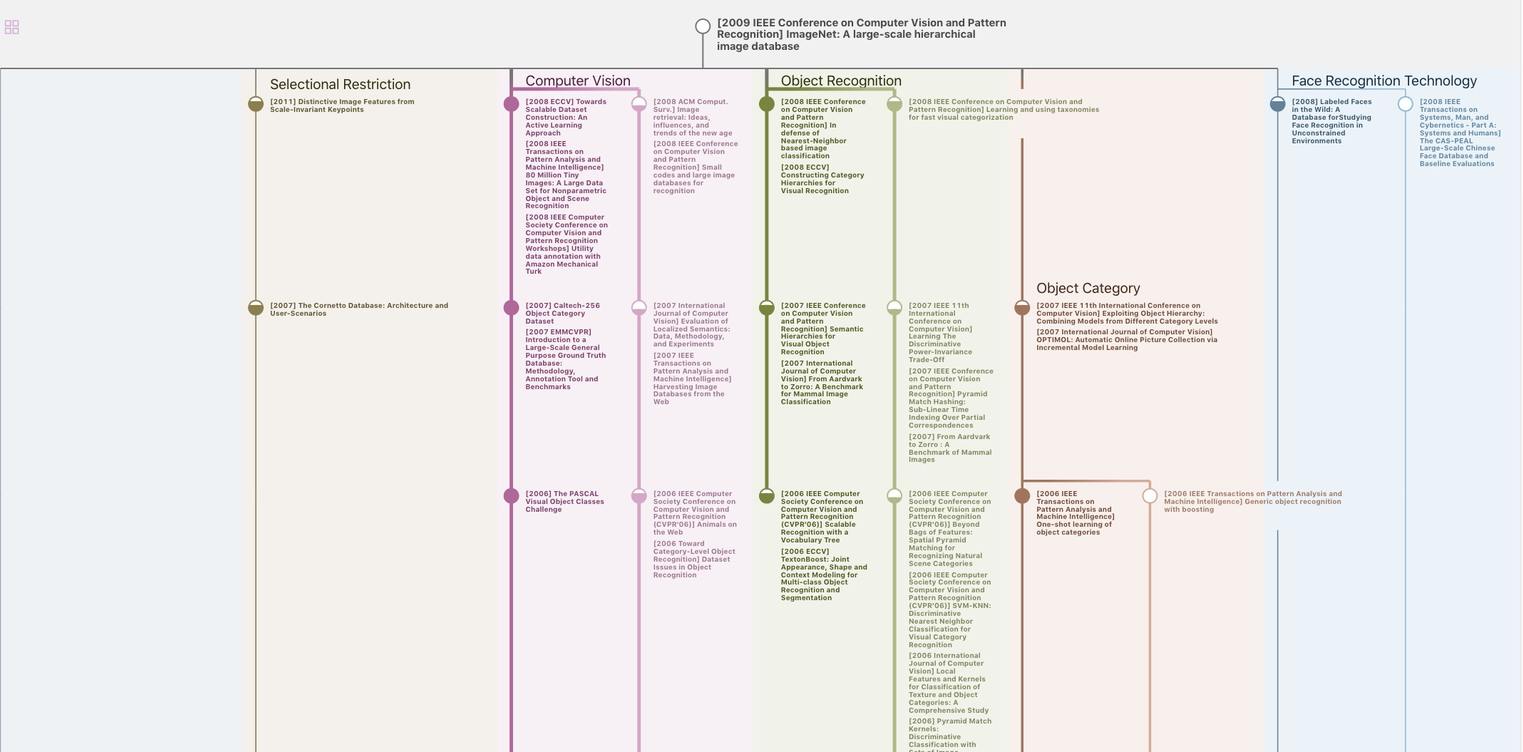
生成溯源树,研究论文发展脉络
Chat Paper
正在生成论文摘要