Chimp
Proceedings of the VLDB Endowment(2022)
摘要
Applications in diverse domains such as astronomy, economics and industrial monitoring, increasingly press the need for analyzing massive collections of time series data. The sheer size of the latter hinders our ability to efficiently store them and also yields significant storage costs. Applying general purpose compression algorithms would effectively reduce the size of the data, at the expense of introducing significant computational overhead. Time Series Management Systems that have emerged to address the challenge of handling this overwhelming amount of information, cannot suffer the ingestion rate restrictions that such compression algorithms would cause. Data points are usually encoded using faster, streaming compression approaches. However, the techniques that contemporary systems use do not fully utilize the compression potential of time series data, with implications in both storage requirements and access times. In this work, we propose a novel streaming compression algorithm, suitable for floating point time series data. We empirically establish properties exhibited by a diverse set of time series and harness these features in our proposed encodings. Our experimental evaluation demonstrates that our approach readily outperforms competing techniques, attaining compression ratios that are competitive with slower general purpose algorithms, and on average around 50% of the space required by state-of-the-art streaming approaches. Moreover, our algorithm outperforms all earlier techniques with regards to both compression and access time , offering a significantly improved trade-off between space and speed. The aforementioned benefits of our approach - in terms of all space requirements, compression time and read access - significantly improve the efficiency in which we can store and analyze time series data.
更多查看译文
关键词
chimp
AI 理解论文
溯源树
样例
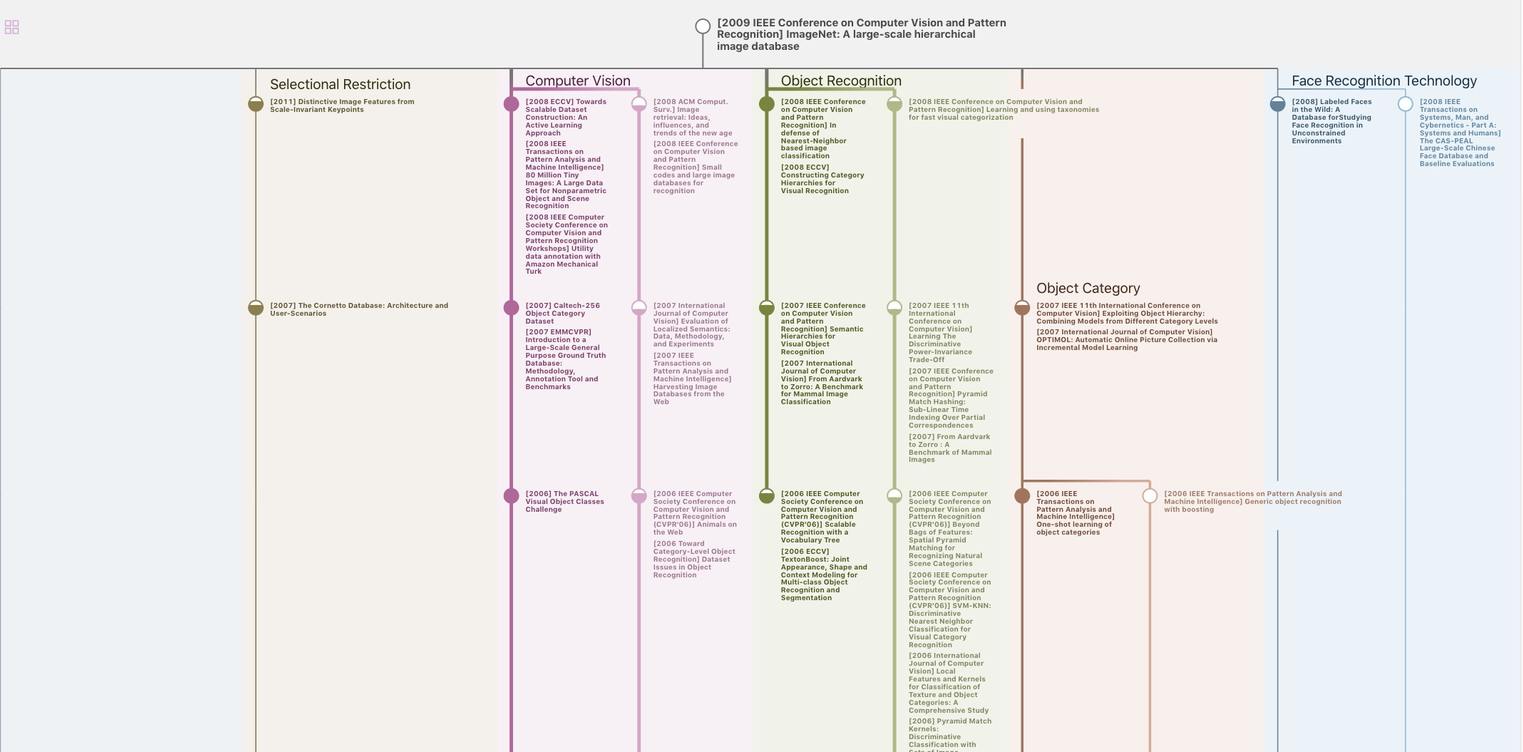
生成溯源树,研究论文发展脉络
Chat Paper
正在生成论文摘要