Are Multimodal Transformers Robust to Missing Modality?
arXiv (Cornell University)(2022)
摘要
Multimodal data collected from the real world are often imperfect due to missing modalities. Therefore multimodal models that are robust against modal-incomplete data are highly preferred. Recently, Transformer models have shown great success in processing multimodal data. However, existing work has been limited to either architecture designs or pre-training strategies; whether Transformer models are naturally robust against missing-modal data has rarely been investigated. In this paper, we present the first-of-its-kind work to comprehensively investigate the behavior of Transformers in the presence of modal-incomplete data. Unsurprising, we find Transformer models are sensitive to missing modalities while different modal fusion strategies will significantly affect the robustness. What surprised us is that the optimal fusion strategy is dataset dependent even for the same Transformer model; there does not exist a universal strategy that works in general cases. Based on these findings, we propose a principle method to improve the robustness of Transformer models by automatically searching for an optimal fusion strategy regarding input data. Experimental validations on three benchmarks support the superior performance of the proposed method.
更多查看译文
关键词
multimodal transformers,modality
AI 理解论文
溯源树
样例
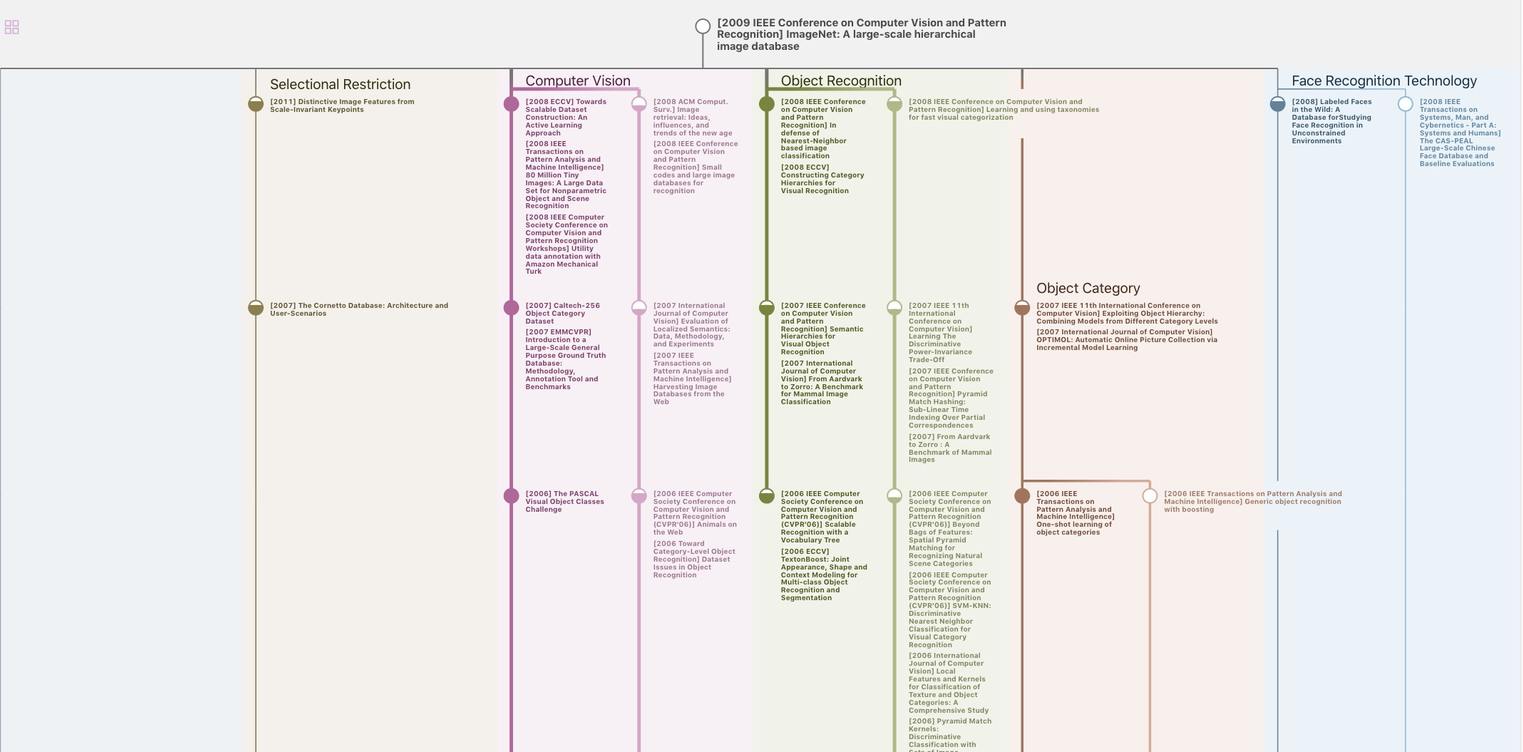
生成溯源树,研究论文发展脉络
Chat Paper
正在生成论文摘要