Group K-SVD for the classification of gene expression data
Computers & Electrical Engineering(2019)
摘要
Abstract In this paper, we propose a new sparse learning model for the classification of gene expression data, named Group K-Singular Value Decomposition (Group K-SVD). It learns the optimal dictionary and sparse representation from the training data, and then assigns the out-of-sample data to the class with the nearest centroid. Group K-SVD reduces the redundancy of over-complete dictionary by using a group update strategy during the dictionary update stage. To solve the optimization problem, we also develop a Multivariate Orthogonal Matching Pursuit (MOMP) algorithm for the sparse coefficient update stage. In the evaluation of the performance of Group K-SVD model, we implement three Group K-SVD algorithms by incorporating MOMP as well as another two popular optimization algorithms into the sparse coefficient update stage. Sufficient experimental results demonstrate that our Group K-SVD algorithms are both effective and efficient in comparison with the state-of-the-methods on real-world gene expression datasets.
更多查看译文
关键词
gene expression data,gene expression,classification,k-svd
AI 理解论文
溯源树
样例
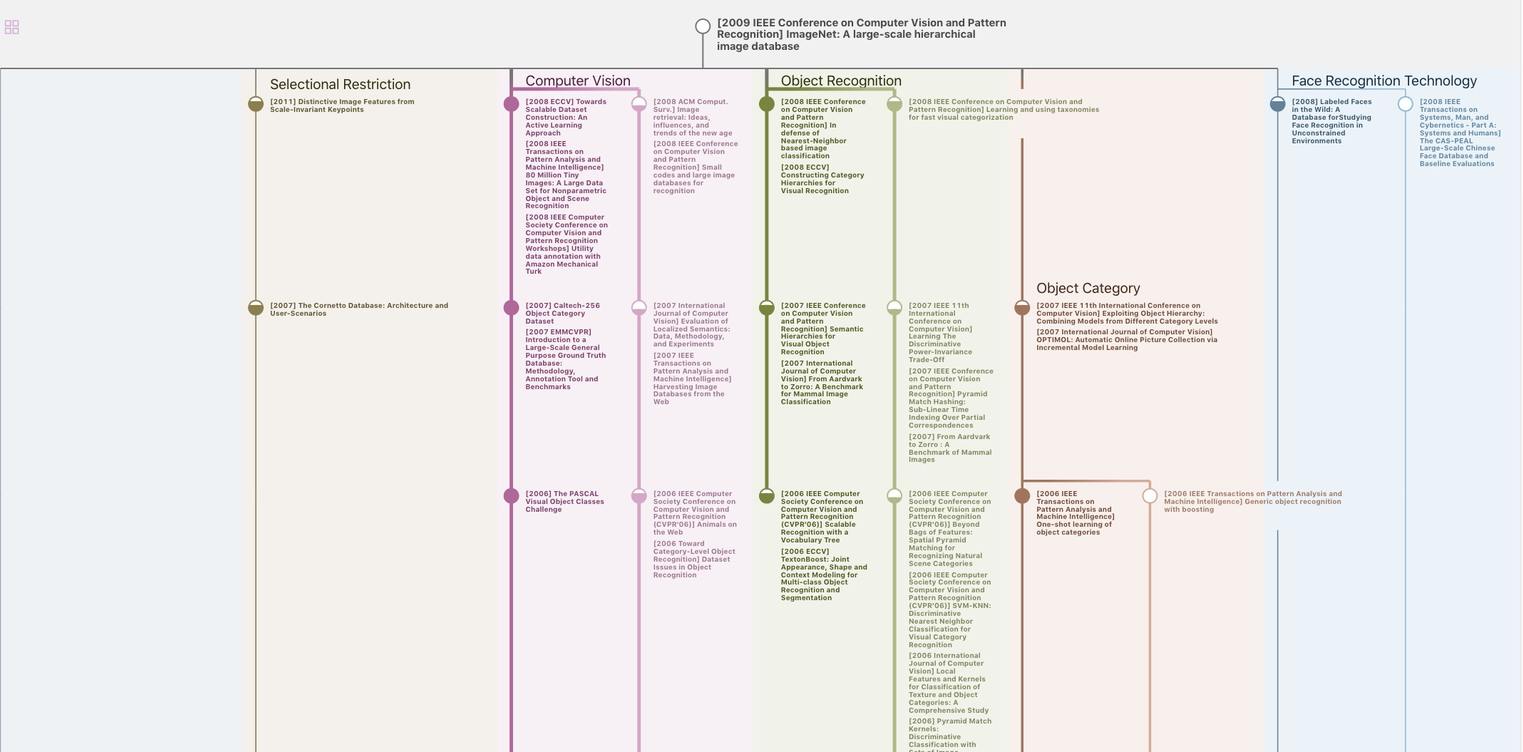
生成溯源树,研究论文发展脉络
Chat Paper
正在生成论文摘要