Multi-modal deep-fusion network for meningioma presurgical grading with integrative imaging and clinical data
The Visual Computer(2023)
摘要
Predicting meningioma grade before surgery is crucial to making decisions on therapy planning and prognosis prediction. Prior works mainly investigate traditional classification techniques with hand-crafted features or rely on image data only, thus having limited accuracy. In this study, we propose a novel multi-modal classification method, i.e., multi-modal deep-fusion network (MMDF), integrating high-dimensional 3D MRI imaging information and low-dimensional tabular clinical data to classify low-grade and high-grade meningiomas. Specifically, the MMDF adopts two modality-specific branches to extract image and clinical features, respectively, and leverages an image-clinical integration module in the shared branch to fuse cross-model features, while specifically considering the impact of low-dimensional clinical data. Besides, we propose a multi-modal image feature aggregation module to integrate three image modalities in the image-specific branch, which can compensate for the feature distribution gaps among the contrast-enhanced T1, contrast-enhanced T2-FLAIR and ADC modalities. Comprehensive experiments show that our approach significantly outperforms the SOTA methods using imaging data only and those combining image and tabular data both, with an AUC of 0.958, sensitivity of 0.877, specificity of 0.926, and accuracy of 0.921. Our approach holds high potential to aid radiologists in doing the presurgical evaluation for clinical decision making.
更多查看译文
关键词
meningioma presurgical grading,integrative imaging,multi-modal,deep-fusion
AI 理解论文
溯源树
样例
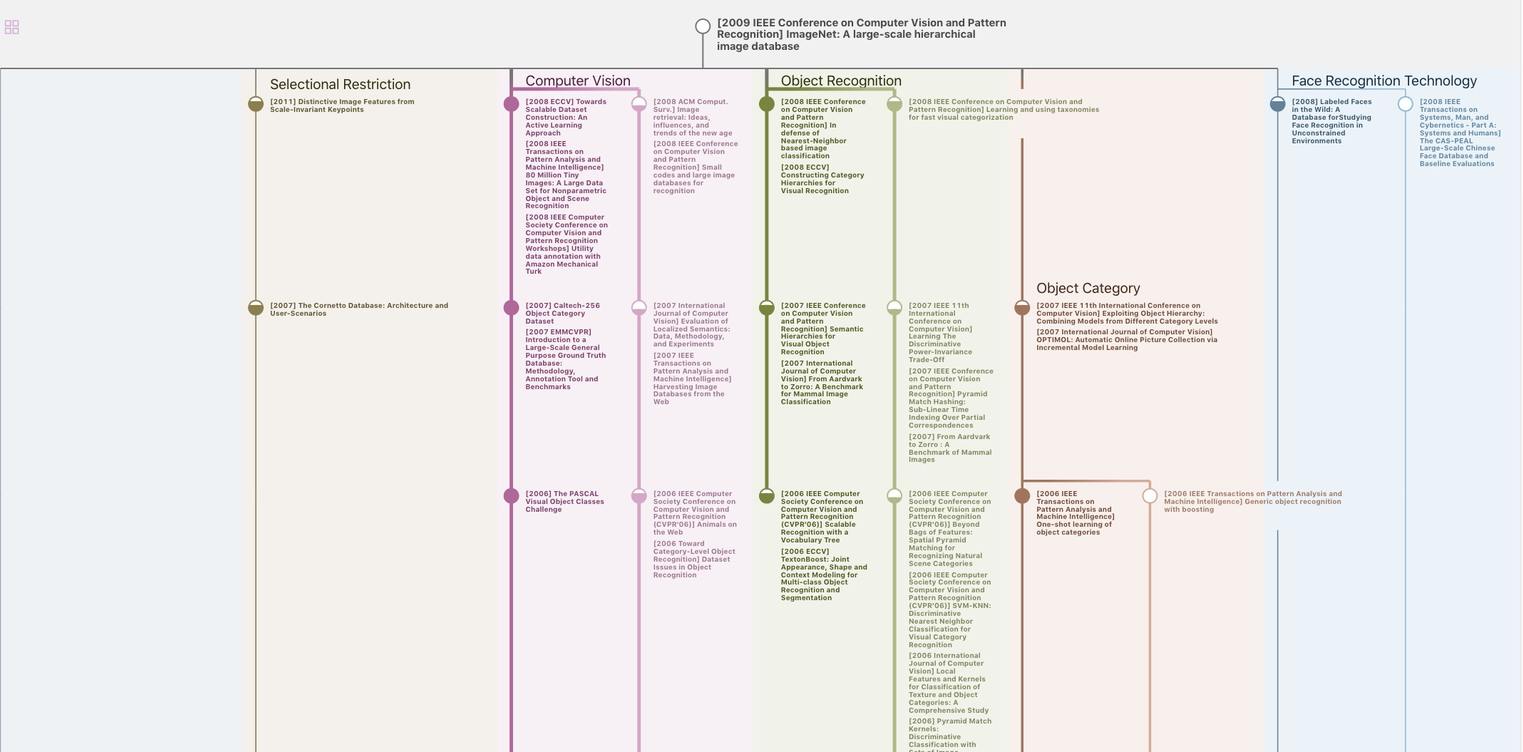
生成溯源树,研究论文发展脉络
Chat Paper
正在生成论文摘要