Vocabulary Matters: An Annotation Pipeline and Two Deep Learning Algorithms for Enzyme Named Entity Recognition
bioRxiv (Cold Spring Harbor Laboratory)(2023)
摘要
Abstract Enzymes are indispensable substances in many biological processes. With biomedical literature growing exponentially, it becomes more difficult to review the literature effectively. Hence, text-mining techniques are needed to facilitate and speed up literature review. The aims of this study are to create a corpus with annotated enzymes to train and evaluate enzyme named-entity recognition (NER) models. A novel pipeline was built using a combination of dictionary matching and rulebased keyword searching to automatically annotate enzyme entities in over 4,800 biomedical full texts. Two Bidirectional Long Short-Term Memory (BiLSTM) networks using BioBERT and SciBERT as tokeniser and word embedding layers were trained on this corpus and evaluated on a manually annotated test set of 526 full-text publications. The dictionary- and rule-based annotation pipeline achieved an F1-score of 0.863 (precision 0.996, recall 0.762). The SciBERT-BiLSTM model (F1-score 0.965, precision 0.981, recall 0.954) largely out-performed the BioBERT-BiLSTM model (F1-score 0.955, precision 0.981, recall 0.937). This study contributed a novel dictionary- and rule-based automatic pipeline with almost perfect precision which runs in a matter of seconds on a standard laptop. Both deep learning (DL) models achieved state-of-the-art performance (F1 > 0.95) for enzyme NER, with the SciBERT-based model outperforming the BioBERT-based model in terms of recall, demonstrating the vocabulary used by models matters. The proposed pipeline with the DL models can facilitate more effective enzyme text-mining and information extraction research for literature review and are the first algorithms specifically for enzyme NER. Availability All codes are available for automatic annotation and model training (including data), with instructions on how to deploy the model on new text, from https://github.com/omicsNLP/enzymeNER .
更多查看译文
关键词
annotation pipeline,deep learning,deep learning algorithms,enzyme
AI 理解论文
溯源树
样例
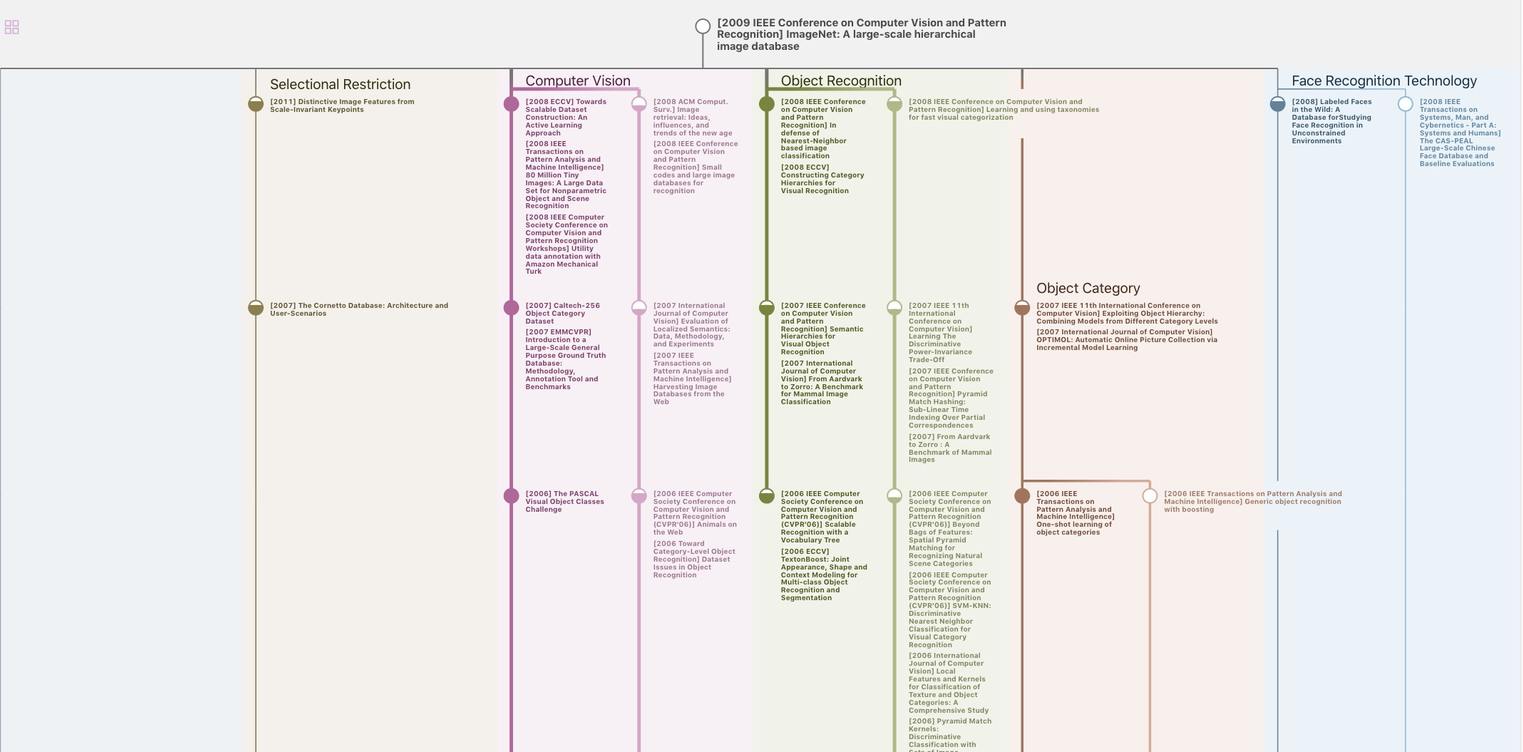
生成溯源树,研究论文发展脉络
Chat Paper
正在生成论文摘要