Self-Prior Guided Pixel Adversarial Networks for Blind Image Inpainting
IEEE Transactions on Pattern Analysis and Machine Intelligence(2023)
摘要
Blind image inpainting involves two critical aspects, i.e. , “where to inpaint” and “how to inpaint”. Knowing “where to inpaint” can eliminate the interference arising from corrupted pixel values; a good “how to inpaint” strategy yields high-quality inpainted results robust to various corruptions. In existing methods, these two aspects usually lack explicit and separate consideration. This paper fully explores these two aspects and proposes a self-prior guided inpainting network (SIN). The self-priors are obtained by detecting semantic-discontinuous regions and by predicting global semantic structures of the input image. On the one hand, the self-priors are incorporated into the SIN, which enables the SIN to perceive valid context information from uncorrupted regions and to synthesize semantic-aware textures for corrupted regions. On the other hand, the self-priors are reformulated to provide a pixel-wise adversarial feedback and a high-level semantic structure feedback, which can promote the semantic continuity of inpainted images. Experimental results demonstrate that our method achieves state-of-the-art performance in metric scores and in visual quality. It has an advantage over many existing methods that assume “where to inpaint” is known in advance. Extensive experiments on a series of related image restoration tasks validate the effectiveness of our method in obtaining high-quality inpainting.
更多查看译文
关键词
pixel adversarial networks,blind image,self-prior
AI 理解论文
溯源树
样例
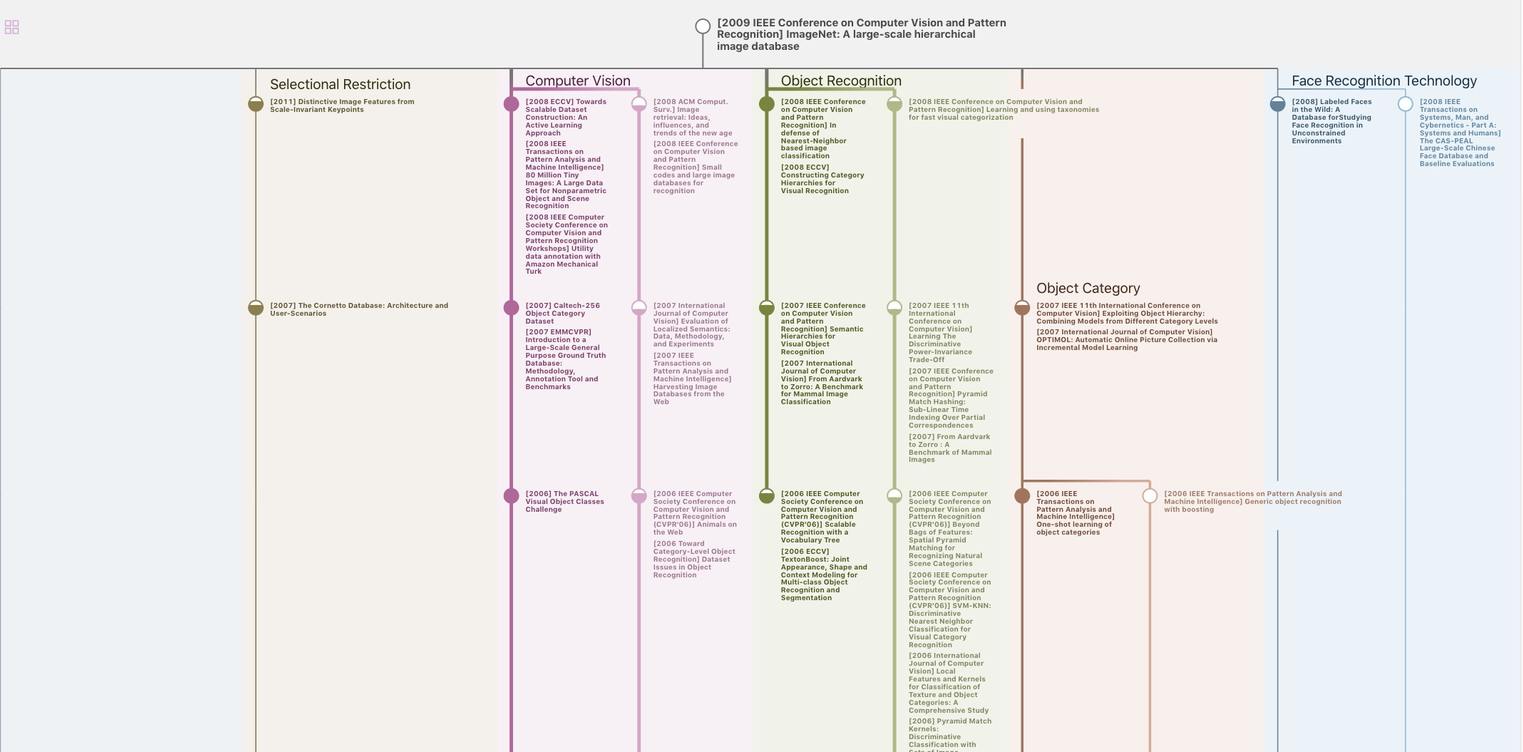
生成溯源树,研究论文发展脉络
Chat Paper
正在生成论文摘要