Two-Stage Asymmetric Similarity Preserving Hashing for Cross-Modal Retrieval
IEEE TRANSACTIONS ON KNOWLEDGE AND DATA ENGINEERING(2024)
摘要
Hashing-based techniques present appealing solutions for cross-modal retrieval due to its low storage requirements and excellent query efficiency. The majority of cross-modal hashing methods typically adopt equal-length encoding scheme to represent multimodal data and achieve cross-modal similarity search. However, such scheme can be regarded as a relatively strict limitation, because it sacrifices the flexible representation of multimodal data in reality and cannot always guarantee the optimal retrieval performance. To address the challenge, this paper focuses on encoding heterogeneous data with varying hash lengths. To achieve this purpose, we propose a flexible cross-modal hashing approach, named Two-stage Asymmetric Similarity Preserving Hashing, TASPH for short, which can be applied to both unequal-length and equal-length retrieval scenarios. Specifically, in the first stage, TASPH designs a novel discrete asymmetric strategy to learn the modality-specific hash codes with varying lengths, enabling a flexible representation of heterogeneous data. Simultaneously, TASPH utilizes two semantic transformation matrices to establish the semantic correlations between varying hash codes. Different from most of the existing approaches that employ relaxation solutions, TASPH satisfies the discrete constraints without any relaxation. In the second stage, the learned semantic transformation matrices are employed to alleviate cross-modal heterogeneity, which guarantees that TASPH can learn more powerful hash functions to improve the discriminative ability of hash codes. Abundant experiments conducted on three benchmark datasets demonstrate encouraging results compared with the state-of-the-art approaches under different retrieval scenarios.
更多查看译文
关键词
Cross-modal retrieval,Hashing,Discrete optimization,Unequal-length encoding,Semantic transformation
AI 理解论文
溯源树
样例
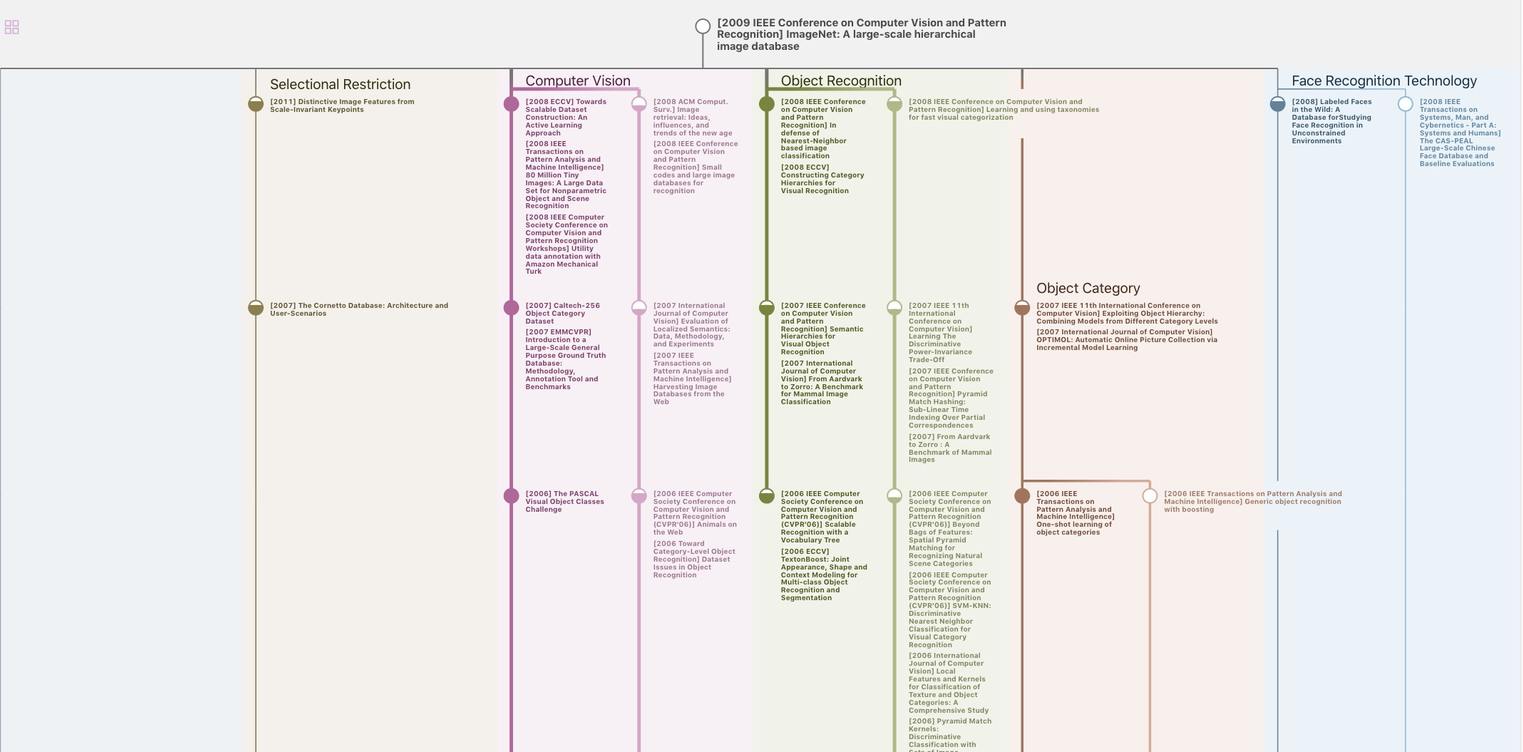
生成溯源树,研究论文发展脉络
Chat Paper
正在生成论文摘要