Federated learning with ℓ 1 regularization
Pattern Recognition Letters(2023)
摘要
Federated Learning (FL) is a widely adopted deep learning method that does not require the collection of raw training data and solves specific learning tasks by federating distributed devices. Due to the heterogeneous distribution of data across clients, the clients will drift toward the local optimal solutions during local training and result in different local models. The global model after aggregating these different local models may keep away from the global optimal solution. This phenomenon is known as client drift, which often hinders the performance of FL. Parameter regularization methods address this challenge of client drift by controlling the update direction of each client. They consider the global model as both the starting point and a reference for the induction bias in the penalty. However, the existing regularization approaches produce dense solutions so that all parameters need to be updated during local model training. At the same time, we note that some studies on deep learning have found that it is unnecessary to update all parameters at each round. Therefore, in this work, we design a novel FL training approach called Fedℓ1 which can alleviate the performance degradation of FL by updating only part of the parameters at each round. ℓ1 regularization is utilized to control the update direction of each client and avoid unnecessary parameter updates at the same time. To our knowledge, our study is the first to introduce sparse regularization term to correct the local training of individual clients in FL. We design a stochastic subgradient descent algorithm to train the ℓ1-regularized nonsmooth model. The comparison experiments with state-of-the-art baselines verify the superiority of the proposed approach.
更多查看译文
关键词
regularization,<mmlmath xmlnsmml=http//wwww3org/1998/math/mathml,learning
AI 理解论文
溯源树
样例
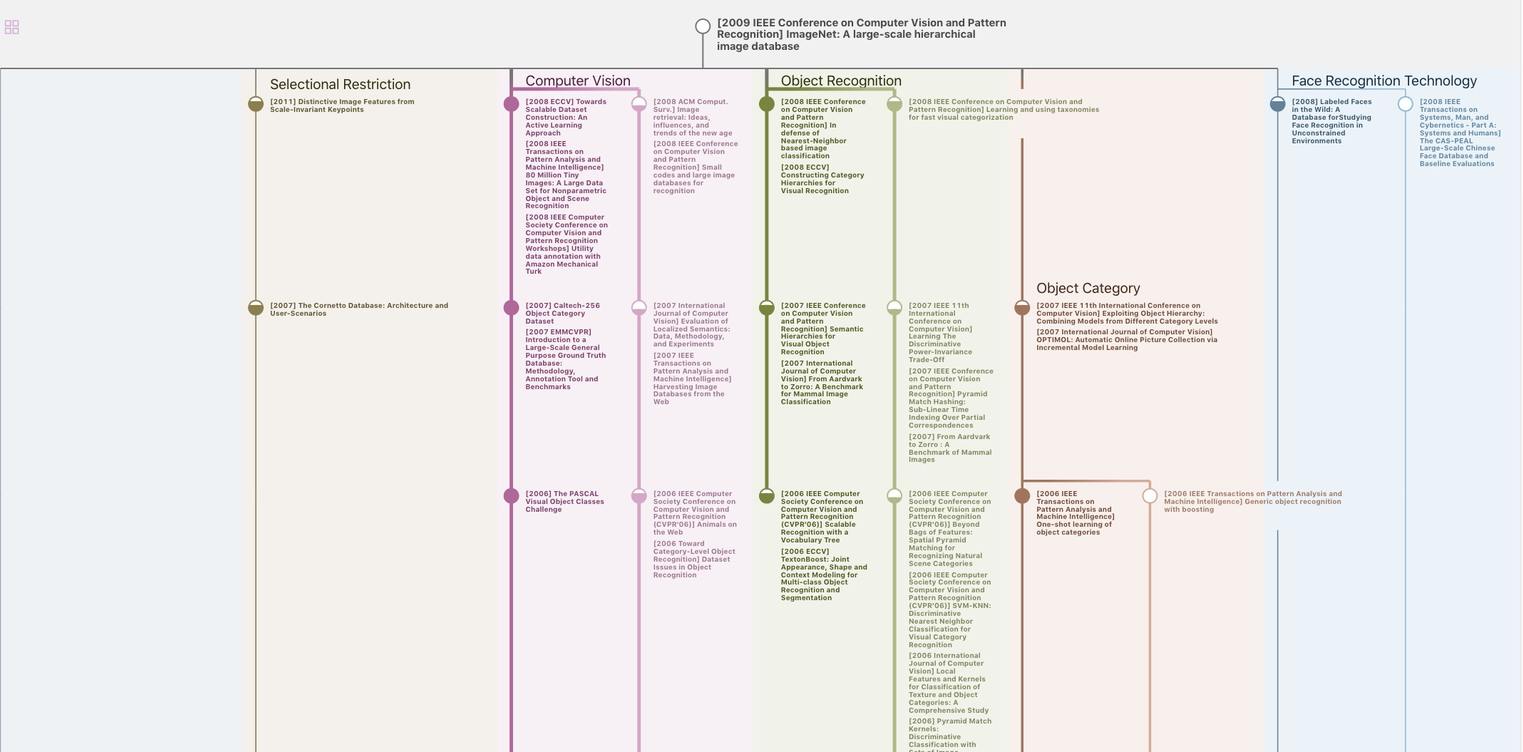
生成溯源树,研究论文发展脉络
Chat Paper
正在生成论文摘要