Dual Consistency-Enhanced Semi-Supervised Sentiment Analysis Towards COVID-19 Tweets
IEEE TRANSACTIONS ON KNOWLEDGE AND DATA ENGINEERING(2023)
摘要
In the context of COVID-19, numerous people present their opinions through social networks. It is thus highly desired to conduct sentiment analysis towards COVID-19 tweets to learn the public's attitudes, and facilitate the government to make proper guidelines for avoiding the social unrest. Although many efforts have studied the text-based sentiment classification from various domains (e.g., delivery and shopping reviews), it is hard to directly use these classifiers for the sentiment analysis towards COVID-19 tweets due to the domain gap. In fact, developing the sentiment classifier for COVID-19 tweets is mainly challenged by the limited annotated training dataset, as well as the diverse and informal expressions of user-generated posts. To address these challenges, we construct a large-scale COVID-19 dataset from Weibo and propose a dual COnsistency-enhanced semi-superVIseD network for Sentiment Anlaysis (COVID-SA). In particular, we first introduce a knowledge-based augmentation method to augment data and enhance the model's robustness. We then employ BERT as the text encoder backbone for both labeled data, unlabeled data, and augmented data. Moreover, we propose a dual consistency (i.e., label-oriented consistency and instance-oriented consistency) regularization to promote the model performance. Extensive experiments on our self-constructed dataset and three public datasets show the superiority of COVID-SA over state-of-the-art baselines on various applications.
更多查看译文
关键词
Index Terms-Semi-supervised text classification,sentiment analysis,social media dataset on COVID-19
AI 理解论文
溯源树
样例
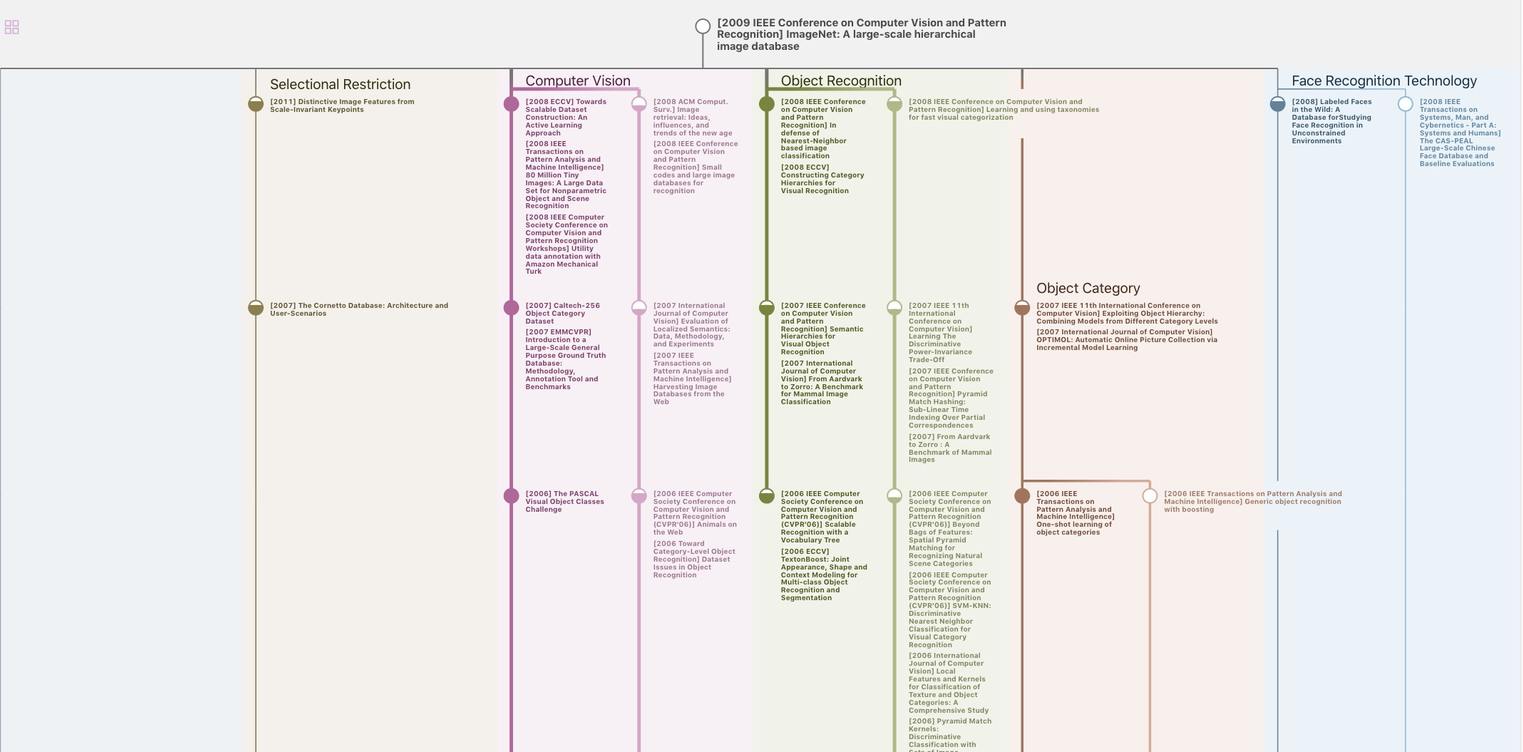
生成溯源树,研究论文发展脉络
Chat Paper
正在生成论文摘要