Filler Word Detection with Hard Category Mining and Inter-Category Focal Loss
arXiv (Cornell University)(2023)
摘要
Filler words like ``um" or ``uh" are common in spontaneous speech. It is desirable to automatically detect and remove them in recordings, as they affect the fluency, confidence, and professionalism of speech. Previous studies and our preliminary experiments reveal that the biggest challenge in filler word detection is that fillers can be easily confused with other hard categories like ``a" or ``I". In this paper, we propose a novel filler word detection method that effectively addresses this challenge by adding auxiliary categories dynamically and applying an additional inter-category focal loss. The auxiliary categories force the model to explicitly model the confusing words by mining hard categories. In addition, inter-category focal loss adaptively adjusts the penalty weight between ``filler" and ``non-filler" categories to deal with other confusing words left in the ``non-filler" category. Our system achieves the best results, with a huge improvement compared to other methods on the PodcastFillers dataset.
更多查看译文
关键词
hard category mining,filler,detection,inter-category
AI 理解论文
溯源树
样例
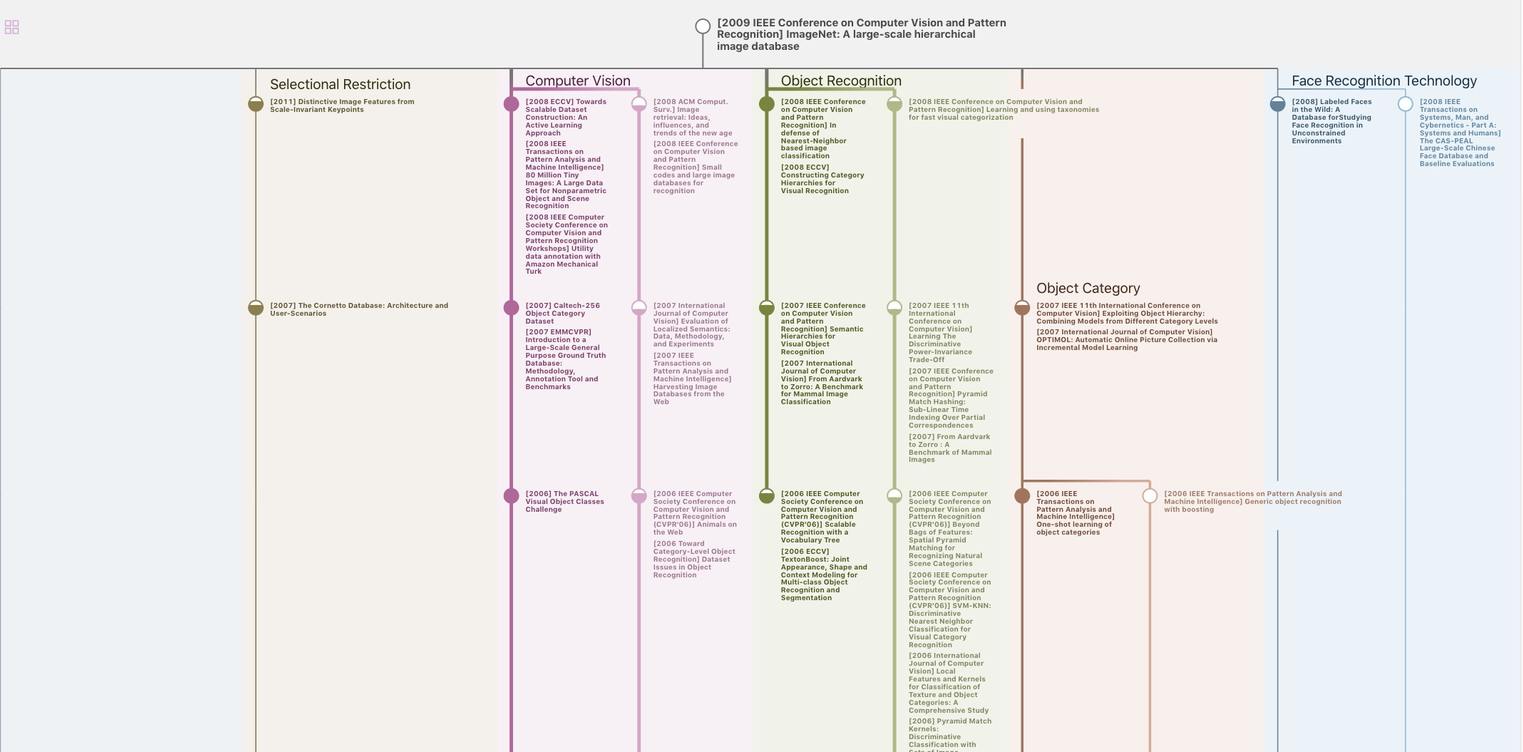
生成溯源树,研究论文发展脉络
Chat Paper
正在生成论文摘要