An intelligent timestamp data manipulation methodology for customer level resource efficient short-term electric load forecasting.
International Journal of Data Analysis Techniques and Strategies(2023)
摘要
Electricity supply companies need to know the expected load consumption to perform better scheduling and planning. Prediction of electric-load usage thus becomes a time-series forecasting problem. Traditionally used statistical, knowledge-based and hybrid techniques for forecasting do not ensure a high level of accuracy, while more accurate techniques like deep learning are computationally expensive and require additional non-temporal data. We propose a significantly accurate but computationally efficient methodology using 'stack ensembling' with two different data manipulations and compare their results with selected baseline predictors as well as existing literature. We only use timestamp information for feature extraction to keep this study independent of non-time features. We achieve a maximum improvement of 34.98% in terms of MAPE over chosen base predictor ARIMA. Limitations of our work include a low degree of accuracy for outliers' estimation in the electric-load consumption on which we plan to improve upon in future work.
更多查看译文
关键词
customer level resource efficient,short-term
AI 理解论文
溯源树
样例
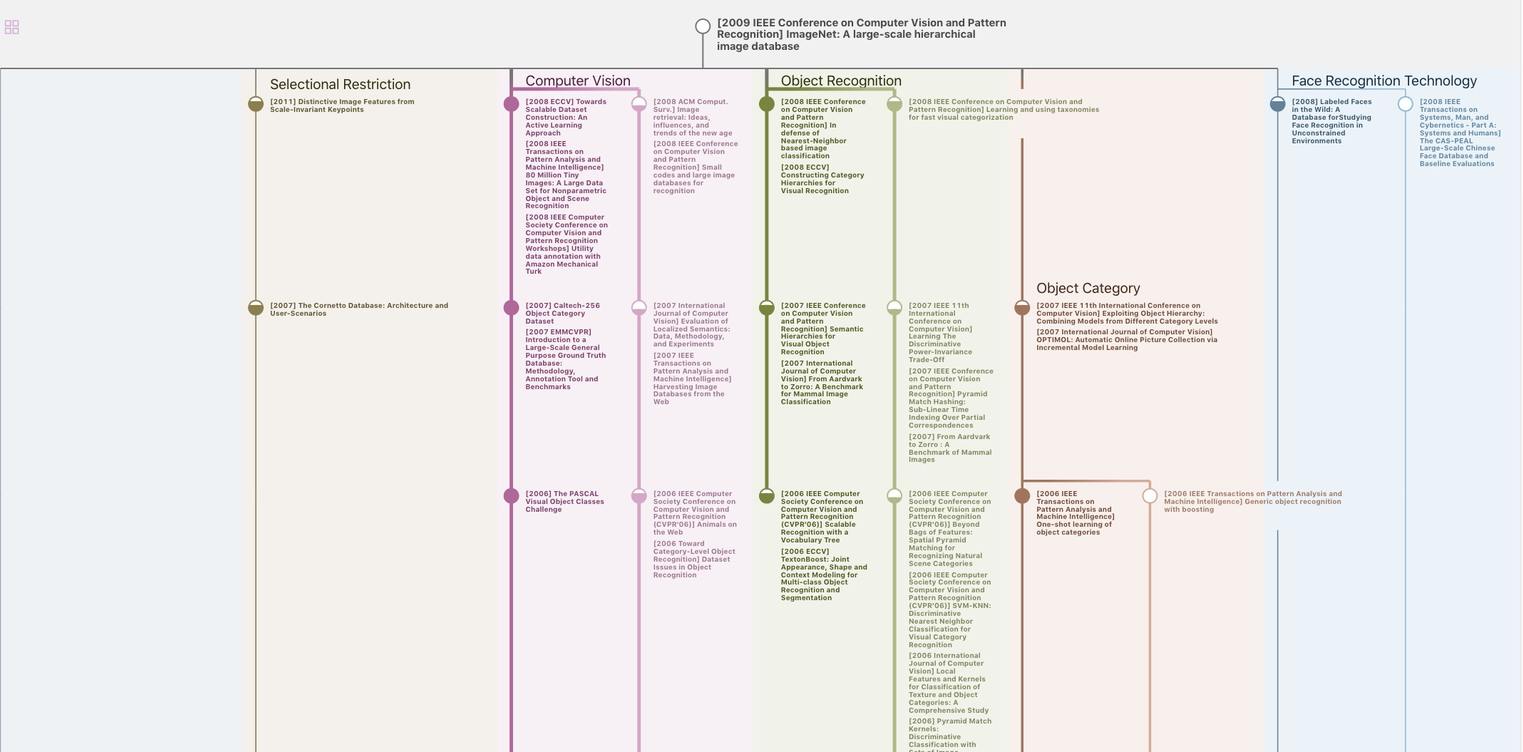
生成溯源树,研究论文发展脉络
Chat Paper
正在生成论文摘要