Active Learning of Piecewise Gaussian Process Surrogates
arXiv (Cornell University)(2023)
摘要
Active learning of Gaussian process (GP) surrogates has been useful for optimizing experimental designs for physical/computer simulation experiments, and for steering data acquisition schemes in machine learning. In this paper, we develop a method for active learning of piecewise, Jump GP surrogates. Jump GPs are continuous within, but discontinuous across, regions of a design space, as required for applications spanning autonomous materials design, configuration of smart factory systems, and many others. Although our active learning heuristics are appropriated from strategies originally designed for ordinary GPs, we demonstrate that additionally accounting for model bias, as opposed to the usual model uncertainty, is essential in the Jump GP context. Toward that end, we develop an estimator for bias and variance of Jump GP models. Illustrations, and evidence of the advantage of our proposed methods, are provided on a suite of synthetic benchmarks, and real-simulation experiments of varying complexity.
更多查看译文
关键词
active learning,process
AI 理解论文
溯源树
样例
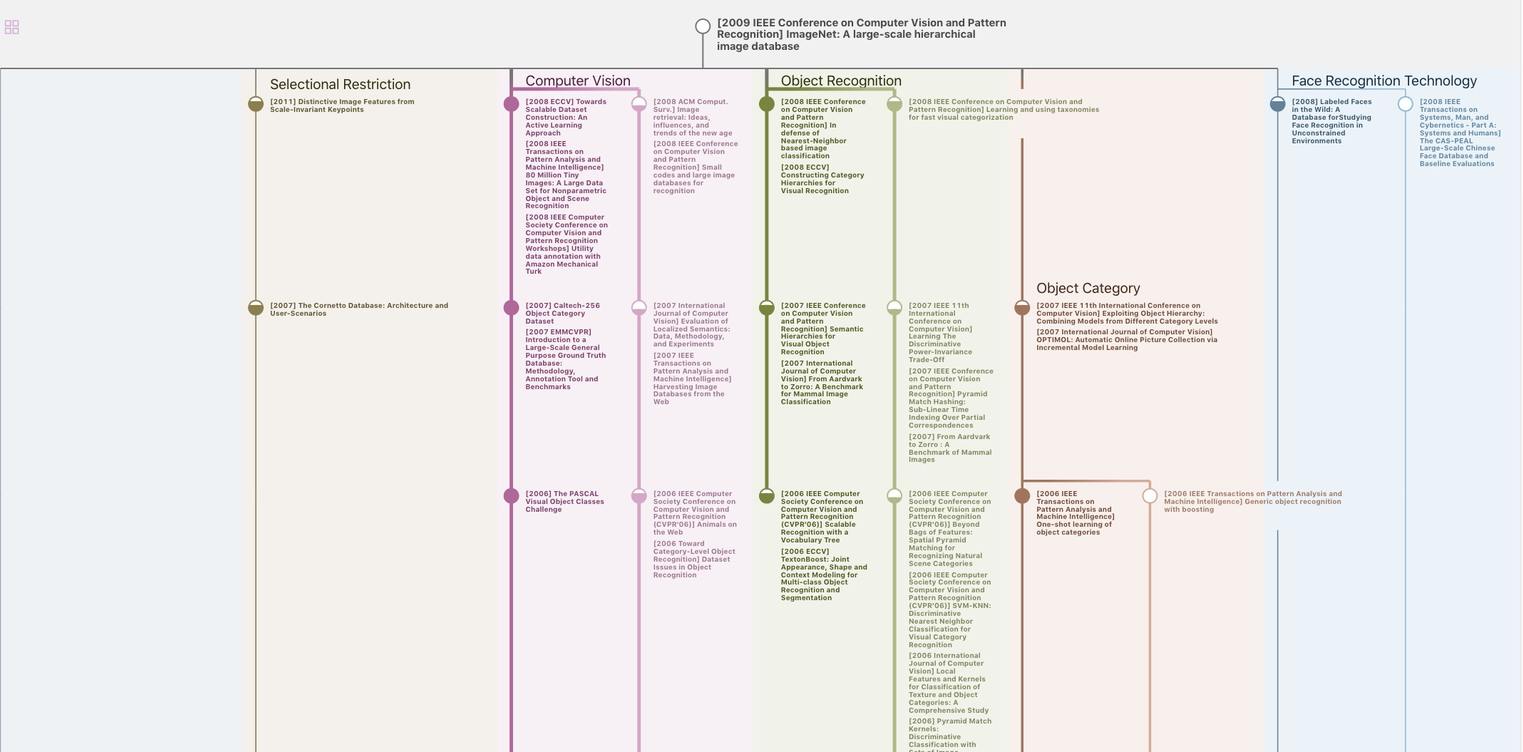
生成溯源树,研究论文发展脉络
Chat Paper
正在生成论文摘要