Identifying predictors of survival in leukemia using single-cell mass cytometry and machine learning
bioRxiv (Cold Spring Harbor Laboratory)(2022)
摘要
Mass cytometry by time-of-flight (CyTOF) allows for in-depth characterisation of cellular heterogeneity in cancer and other diseases. However, computational identification of cell populations from CyTOF, and utilisation of single-cell data for biomarker discoveries is challenging. Here, we deployed a bioinformatics framework that enables automated cell population annotation, and systematic exploration of interactions between signalling proteins in a CyTOF antibody panel. As a case study, we applied the developed framework to analyse a cohort of 45 leukemia patients. We investigated associations between the cellular composition and clinicopathological and genetic features and reported salient signalling interactions that were sufficient to predict short-term survival. We also demonstrate that targeting cell type-specific signalling interactions in leukemia might improve existing patient risk stratification methods. Our work introduces a more general analysis framework for CyTOF data with many applications in future personalised medicine projects.
更多查看译文
关键词
leukemia,cytometry,machine learning,single-cell
AI 理解论文
溯源树
样例
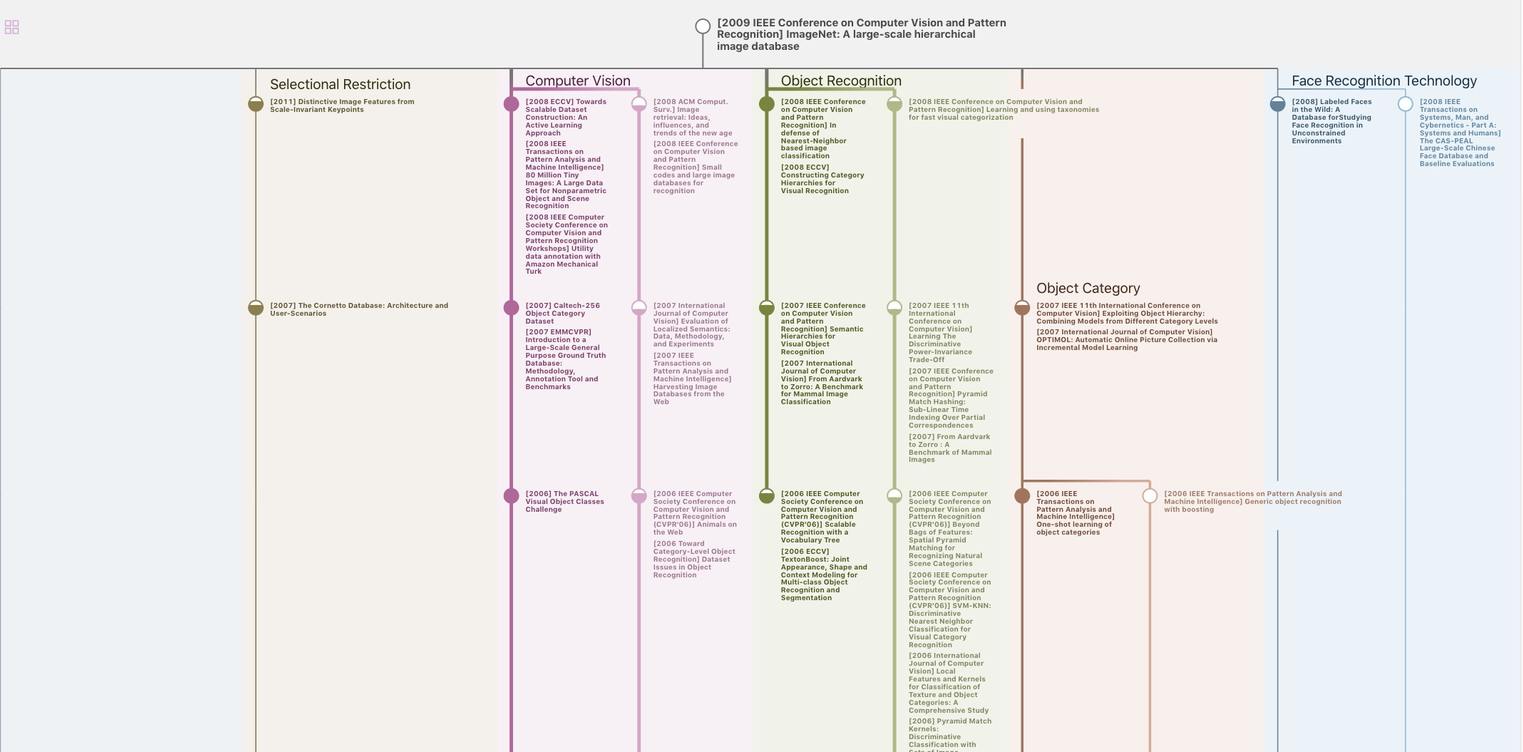
生成溯源树,研究论文发展脉络
Chat Paper
正在生成论文摘要