Settling the Bias and Variance of Meta-Gradient Estimation for Meta-Reinforcement Learning
arXiv (Cornell University)(2021)
摘要
In recent years, gradient based Meta-RL (GMRL) methods have achieved remarkable successes in either discovering effective online hyperparameter for one single task (Xu et al., 2018) or learning good initialisation for multi-task transfer learning (Finn et al., 2017). Despite the empirical successes, it is often neglected that computing meta gradients via vanilla backpropagation is ill-defined. In this paper, we argue that the stochastic meta-gradient estimation adopted by many existing MGRL methods are in fact biased; the bias comes from two sources: 1) the compositional bias that is inborn in the structure of compositional optimisation problems and 2) the bias of multi-step Hessian estimation caused by direct automatic differentiation. To better understand the meta gradient biases, we perform the first of its kind study to quantify the amount for each of them. We start by providing a unifying derivation for existing GMRL algorithms, and then theoretically analyse both the bias and the variance of existing gradient estimation methods. On understanding the underlying principles of bias, we propose two mitigation solutions based on off-policy correction and multi-step Hessian estimation techniques. Comprehensive ablation studies have been conducted and results reveals: (1) The existence of these two biases and how they influence the meta-gradient estimation when combined with different estimator/sample size/step and learning rate. (2) The effectiveness of these mitigation approaches for meta-gradient estimation and thereby the final return on two practical Meta-RL algorithms: LOLA-DiCE and Meta-gradient Reinforcement Learning.
更多查看译文
关键词
bias,learning,meta-gradient,meta-reinforcement
AI 理解论文
溯源树
样例
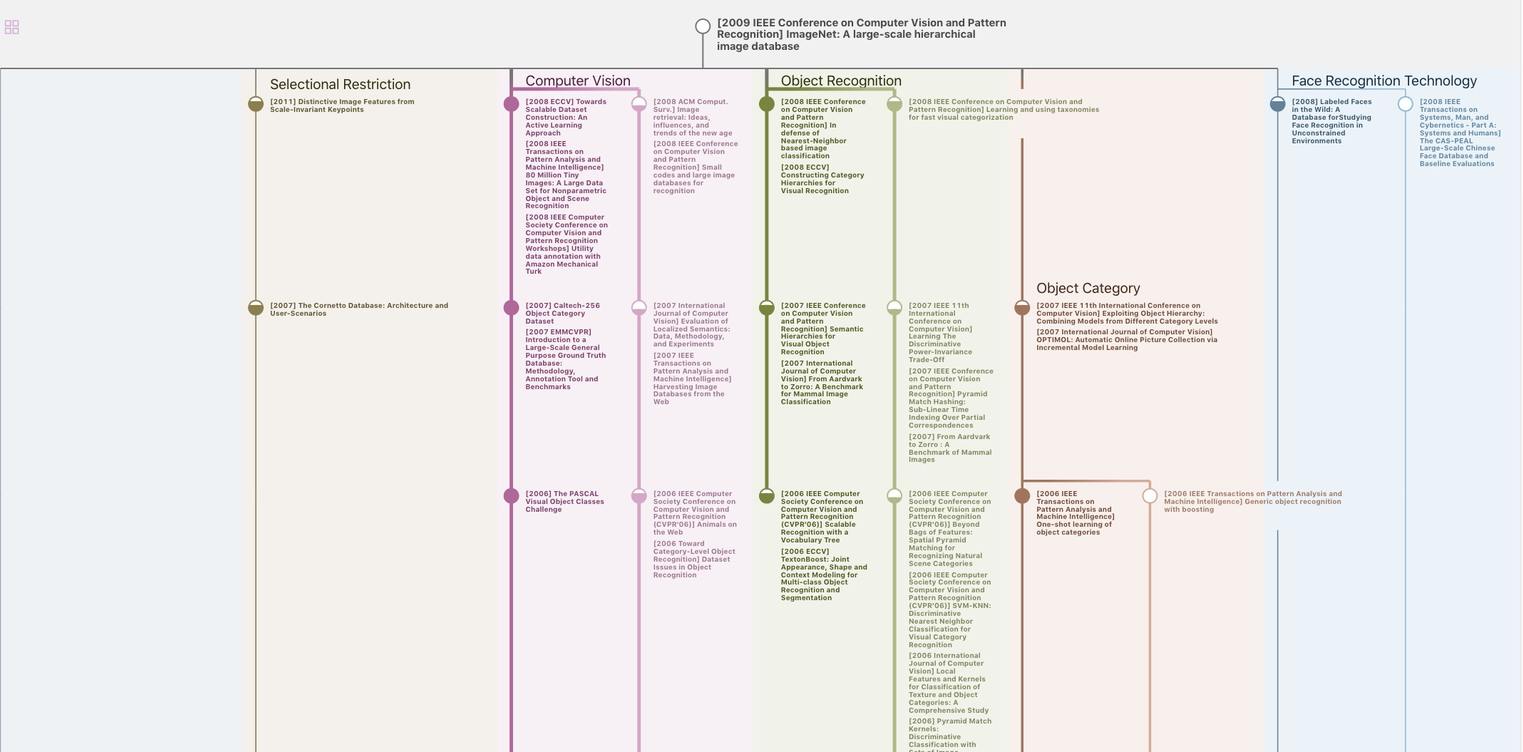
生成溯源树,研究论文发展脉络
Chat Paper
正在生成论文摘要