Implicit Autoencoder for Point-Cloud Self-Supervised Representation Learning
arXiv (Cornell University)(2022)
摘要
This paper advocates the use of implicit surface representation in autoencoder-based self-supervised 3D representation learning. The most popular and accessible 3D representation, i.e., point clouds, involves discrete samples of the underlying continuous 3D surface. This discretization process introduces sampling variations on the 3D shape, making it challenging to develop transferable knowledge of the true 3D geometry. In the standard autoencoding paradigm, the encoder is compelled to encode not only the 3D geometry but also information on the specific discrete sampling of the 3D shape into the latent code. This is because the point cloud reconstructed by the decoder is considered unacceptable unless there is a perfect mapping between the original and the reconstructed point clouds. This paper introduces the Implicit AutoEncoder (IAE), a simple yet effective method that addresses the sampling variation issue by replacing the commonly-used point-cloud decoder with an implicit decoder. The implicit decoder reconstructs a continuous representation of the 3D shape, independent of the imperfections in the discrete samples. Extensive experiments demonstrate that the proposed IAE achieves state-of-the-art performance across various self-supervised learning benchmarks.
更多查看译文
关键词
autoencoder,learning,representation
AI 理解论文
溯源树
样例
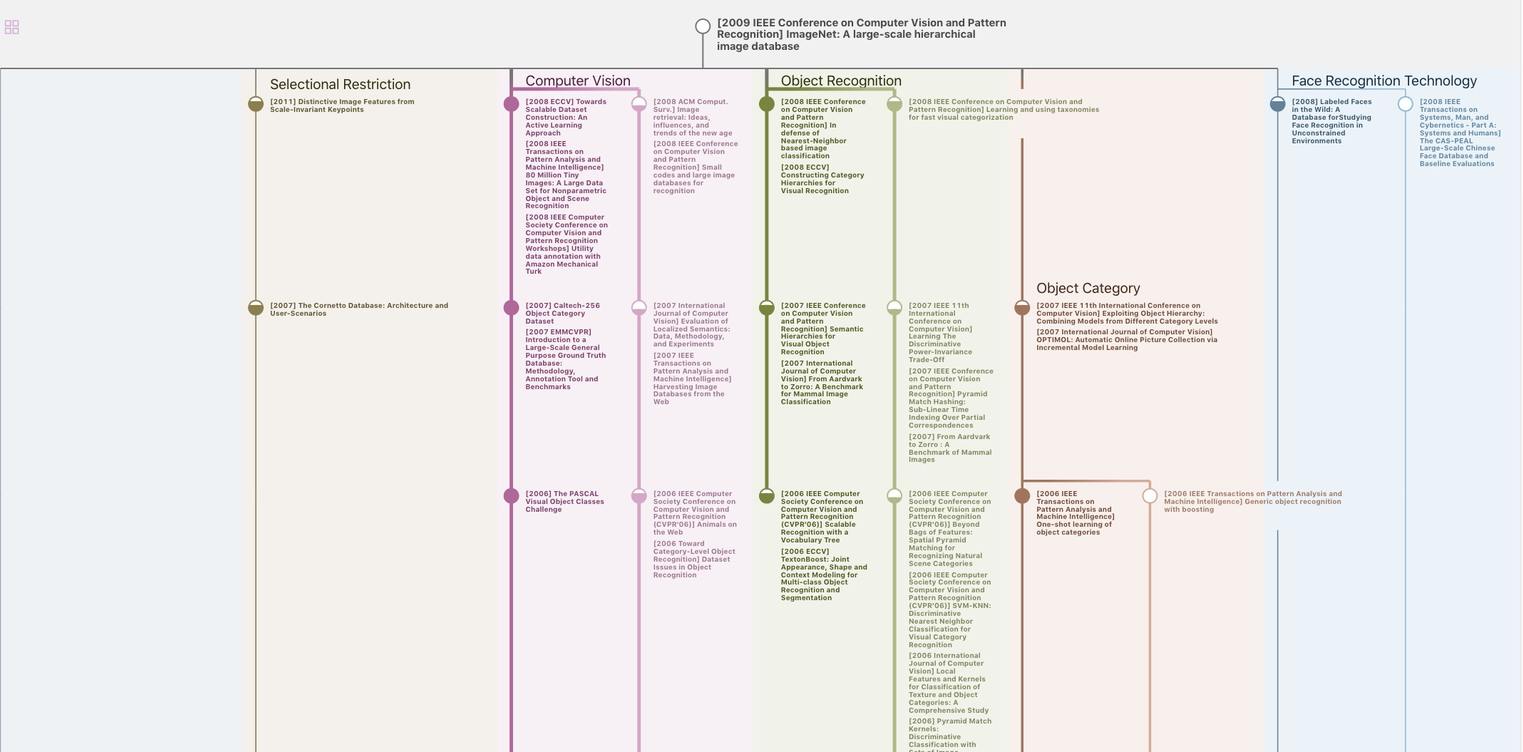
生成溯源树,研究论文发展脉络
Chat Paper
正在生成论文摘要