On the Importance of Sampling in Training GCNs: Tighter Analysis and Variance Reduction
arXiv (Cornell University)(2021)
摘要
Graph Convolutional Networks (GCNs) have achieved impressive empirical advancement across a wide variety of semi-supervised node classification tasks. Despite their great success, training GCNs on large graphs suffers from computational and memory issues. A potential path to circumvent these obstacles is sampling-based methods, where at each layer a subset of nodes is sampled. Although recent studies have empirically demonstrated the effectiveness of sampling-based methods, these works lack theoretical convergence guarantees under realistic settings and cannot fully leverage the information of evolving parameters during optimization. In this paper, we describe and analyze a general doubly variance reduction schema that can accelerate any sampling method under the memory budget. The motivating impetus for the proposed schema is a careful analysis of the variance of sampling methods where it is shown that the induced variance can be decomposed into node embedding approximation variance (zeroth-order variance) during forward propagation and layerwise-gradient variance (first-order variance) during backward propagation. We theoretically analyze the convergence of the proposed schema and show that it enjoys an $\mathcal{O}(1/T)$ convergence rate. We complement our theoretical results by integrating the proposed schema in different sampling methods and applying them to different large real-world graphs.
更多查看译文
关键词
training gcns,sampling,variance reduction
AI 理解论文
溯源树
样例
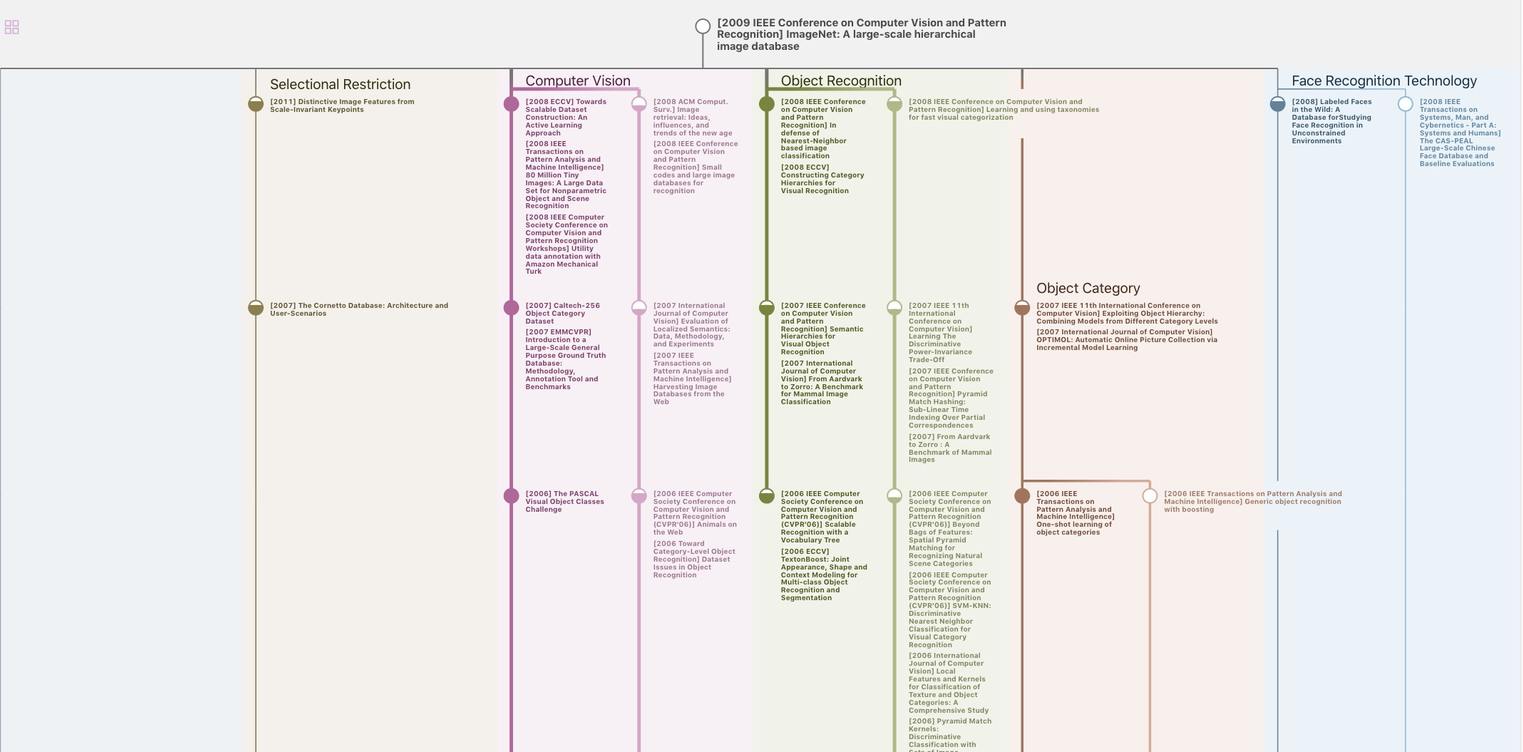
生成溯源树,研究论文发展脉络
Chat Paper
正在生成论文摘要