RLlib Flow: Distributed Reinforcement Learning is a Dataflow Problem
arXiv (Cornell University)(2020)
摘要
Researchers and practitioners in the field of reinforcement learning (RL) frequently leverage parallel computation, which has led to a plethora of new algorithms and systems in the last few years. In this paper, we re-examine the challenges posed by distributed RL and try to view it through the lens of an old idea: distributed dataflow. We show that viewing RL as a dataflow problem leads to highly composable and performant implementations. We propose RLlib Flow, a hybrid actor-dataflow programming model for distributed RL, and validate its practicality by porting the full suite of algorithms in RLlib, a widely adopted distributed RL library. Concretely, RLlib Flow provides 2-9 code savings in real production code and enables the composition of multi-agent algorithms not possible by end users before. The open-source code is available as part of RLlib at https://github.com/ray-project/ray/tree/master/rllib.
更多查看译文
关键词
reinforcement learning,dataflow problem,flow
AI 理解论文
溯源树
样例
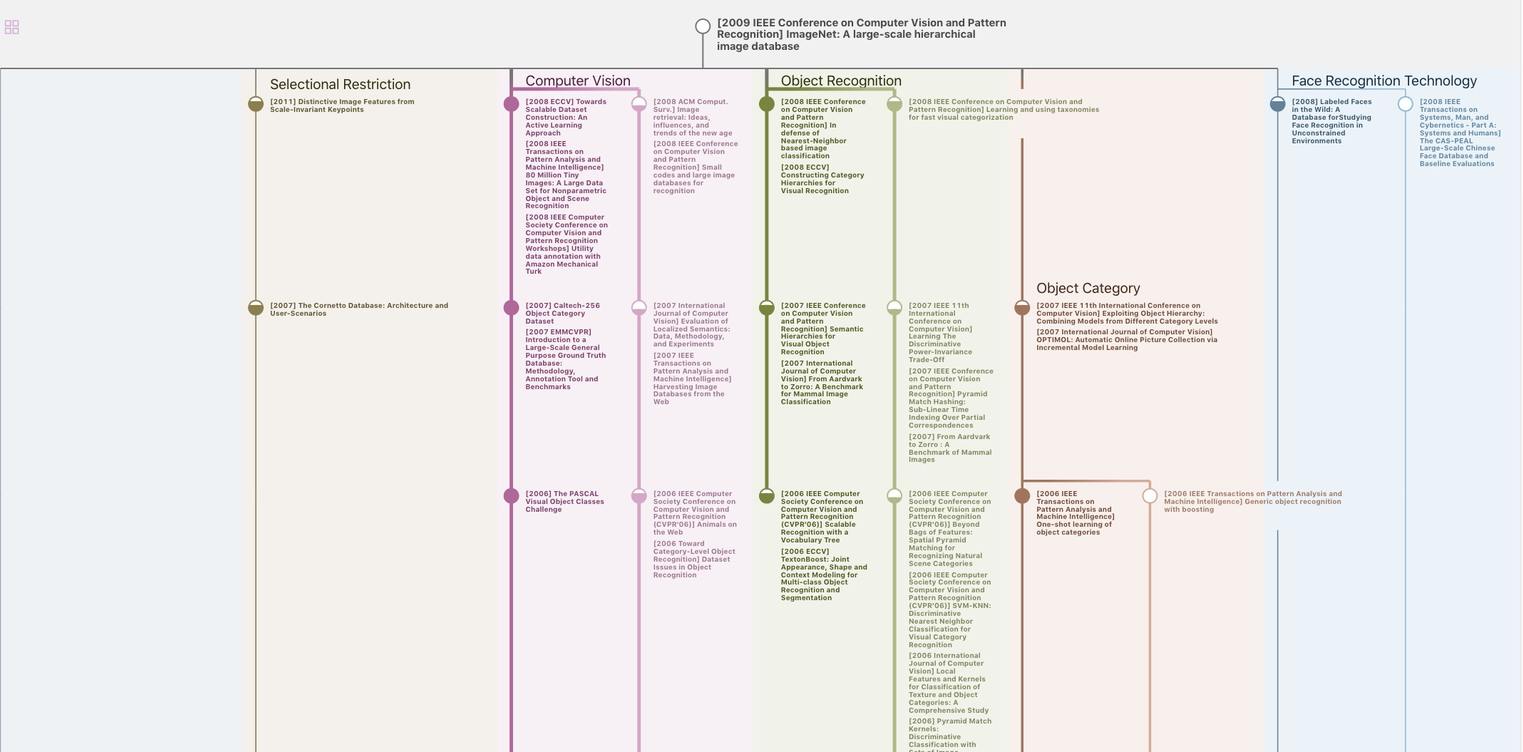
生成溯源树,研究论文发展脉络
Chat Paper
正在生成论文摘要