Machine learning prediction of future amyloid beta positivity in amyloid-negative individuals
Alzheimer's Research & Therapy(2024)
摘要
Background The pathophysiology of Alzheimer’s disease (AD) involves β -amyloid (A β ) accumulation. Early identification of individuals with abnormal β -amyloid levels is crucial, but A β quantification with positron emission tomography (PET) and cerebrospinal fluid (CSF) is invasive and expensive. Methods We propose a machine learning framework using standard non-invasive (MRI, demographics, APOE, neuropsychology) measures to predict future A β -positivity in A β -negative individuals. We separately study A β -positivity defined by PET and CSF. Results Cross-validated AUC for 4-year A β conversion prediction was 0.78 for the CSF-based and 0.68 for the PET-based A β definitions. Although not trained for the clinical status-change prediction, the CSF-based model excelled in predicting future mild cognitive impairment (MCI)/dementia conversion in cognitively normal/MCI individuals (AUCs, respectively, 0.76 and 0.89 with a separate dataset). Conclusion Standard measures have potential in detecting future A β -positivity and assessing conversion risk, even in cognitively normal individuals. The CSF-based definition led to better predictions than the PET-based definition.
更多查看译文
关键词
Machine learning,Amyloid beta,Conversion prediction,Alzheimer’s disease,Mild cognitive impairment
AI 理解论文
溯源树
样例
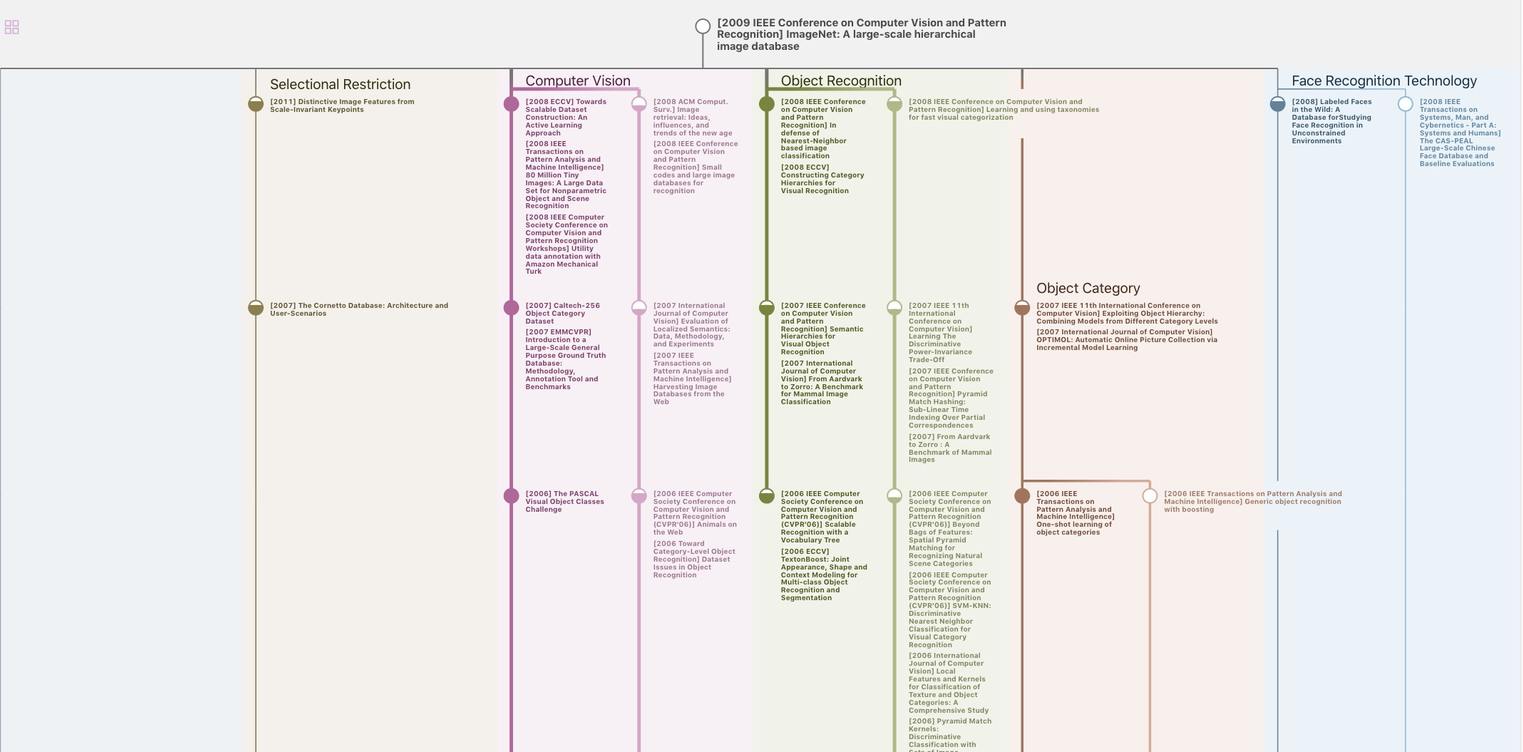
生成溯源树,研究论文发展脉络
Chat Paper
正在生成论文摘要