Automated detection and time measurement of rectal retroflexion using artificial intelligence - a multi centre study
Poster presentations(2023)
摘要
Introduction
Rectal retroflexion rate (RR) is a key performance indicator (KPI) for colonoscopy. However, its measurement is often imprecise and cumbersome to audit as it relies on manual photo documentation and manual data entry in endoscopy reporting systems. Furthermore, it is not possible to quantify inspection time in the RR position. We aimed to develop a convolutional neural network (CNN) to automate detection of RR and quantify inspection time in the RR position (‘rectal retroflexion time’).Methods
Endoscopy videos were prospectively collected from a single centre for training data (Site 1). Each video frame with visualisation of the endoscope in the RR position was annotated with a label of ‘RR’, and the remaining frames labelled as ‘negative’ for RR. The RR CNN was then evaluated with colonoscopy videos recorded from nine sites enrolled in a randomised controlled trial (‘CADDIE Trial’) that evaluates a polyp detection CNN. We randomly selected two endoscopists from each site (n=18) enrolled in the CADDIE trial and ten procedures from each endoscopist (n=180). These nine sites include Site 1 (internal test-set) (n=20) and Sites 2–9 (external test-set) (n=160). Videos were annotated as outlined above which was referenced as the ground truth.Results
A weakly-supervised ResNet-101 CNN was trained with 185 video procedures collected from Site 1 (71,121 RR frames and 142,242 randomly sampled negative frames). RR was performed in each procedure in the CADDIE Trial test set (180/180). The CNN detected RR in 98.3% of procedures in the test-set (177/180). Each of the three procedures where it failed to detect RR were from a different site. In the per-frame analysis (51,134 RR frames, 102,268 negative frames), the accuracy was 97.6%, sensitivity 94.7%, specificity 99.0% and area under the curve 0.98. The ground truth median RR time was 7.6 seconds (IQR 4.5 – 12.3), and the artificial intelligence (AI) predicted median RR time was 7.4 seconds (IQR 4.2 – 12.2) (figure 1).Conclusions
We demonstrated robust results in this novel application of AI to automate RR ‘detection’ and ‘time measurement’. The considerable variation in RR time amongst endoscopists warrants further exploration as a possible new KPI.查看译文
关键词
rectal retroflexion
AI 理解论文
溯源树
样例
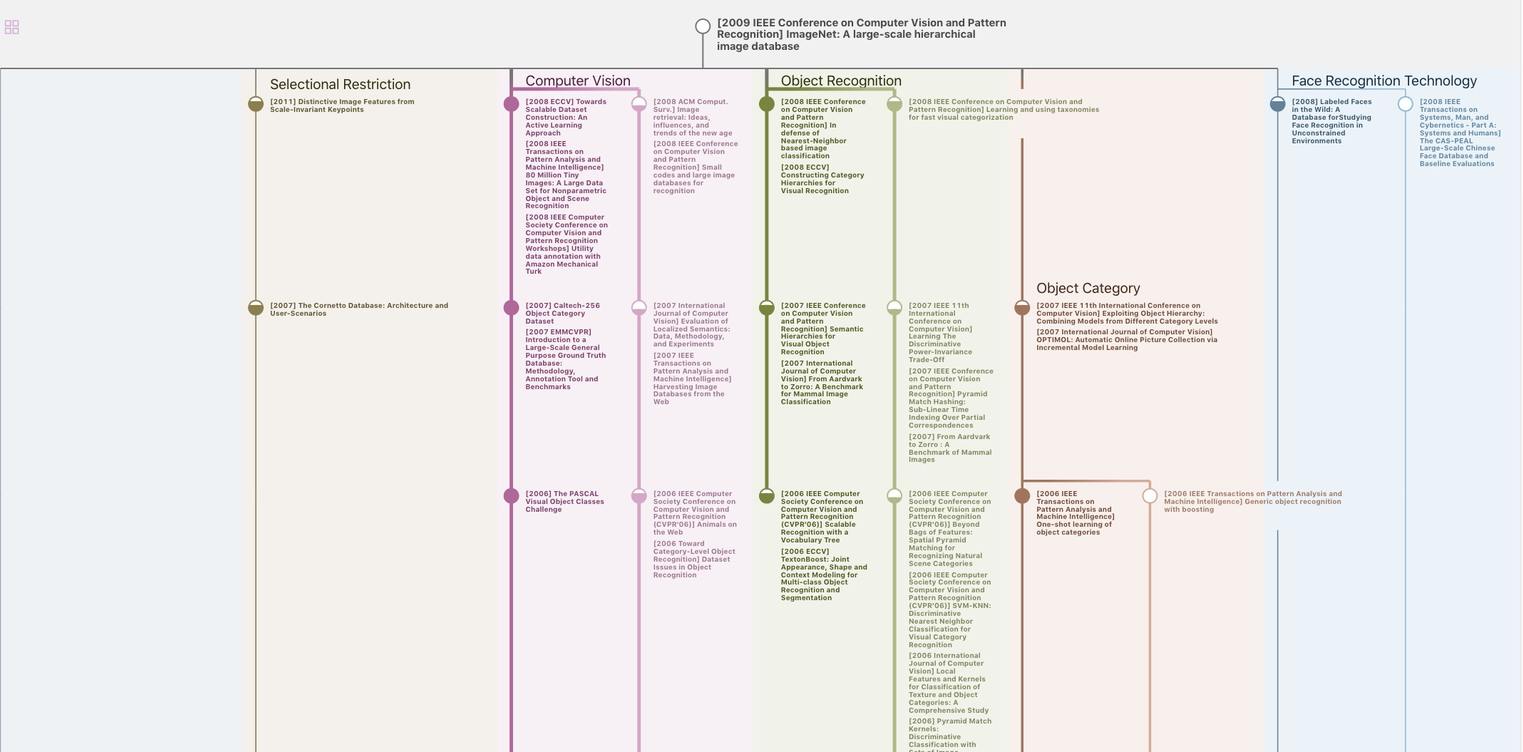
生成溯源树,研究论文发展脉络
Chat Paper
正在生成论文摘要