A novel neural network bounds-tightening procedure for multiparametric programming and control
Computer-aided chemical engineering(2023)
摘要
Recently, it has been shown that rectified linear unit (ReLU) based neural networks (NN) are mixed integer linear representable and therefore can be incorporated into mixed integer linear programming frameworks. In this work we propose a novel tightening procedure that is based on a multiparametric programming formulation of the corresponding ReLU-reformulated optimization problem. The bounding procedure features 1) the generation of valid tight bounds on the individual auxiliary variables introduced from the ReLU NN reformulation, and 2) bounds on groups of input and output variables to these ReLU NNs. The tightened bounds are valid for all parameter realizations, and thus can be reused between different problem realizations. This tightening only needs to be computed a single time and thus the overall procedure is once-and-offline. As this bounds-tightening procedure is applied offline, more computationally expensive methods for formulation tightening can then be applied to the online case. We demonstrate the effectiveness of this method in a case study of model predictive control of a nonlinear chemostat where the dynamics are approximated with a ReLU NN.
更多查看译文
关键词
multiparametric programming,neural network,bounds-tightening
AI 理解论文
溯源树
样例
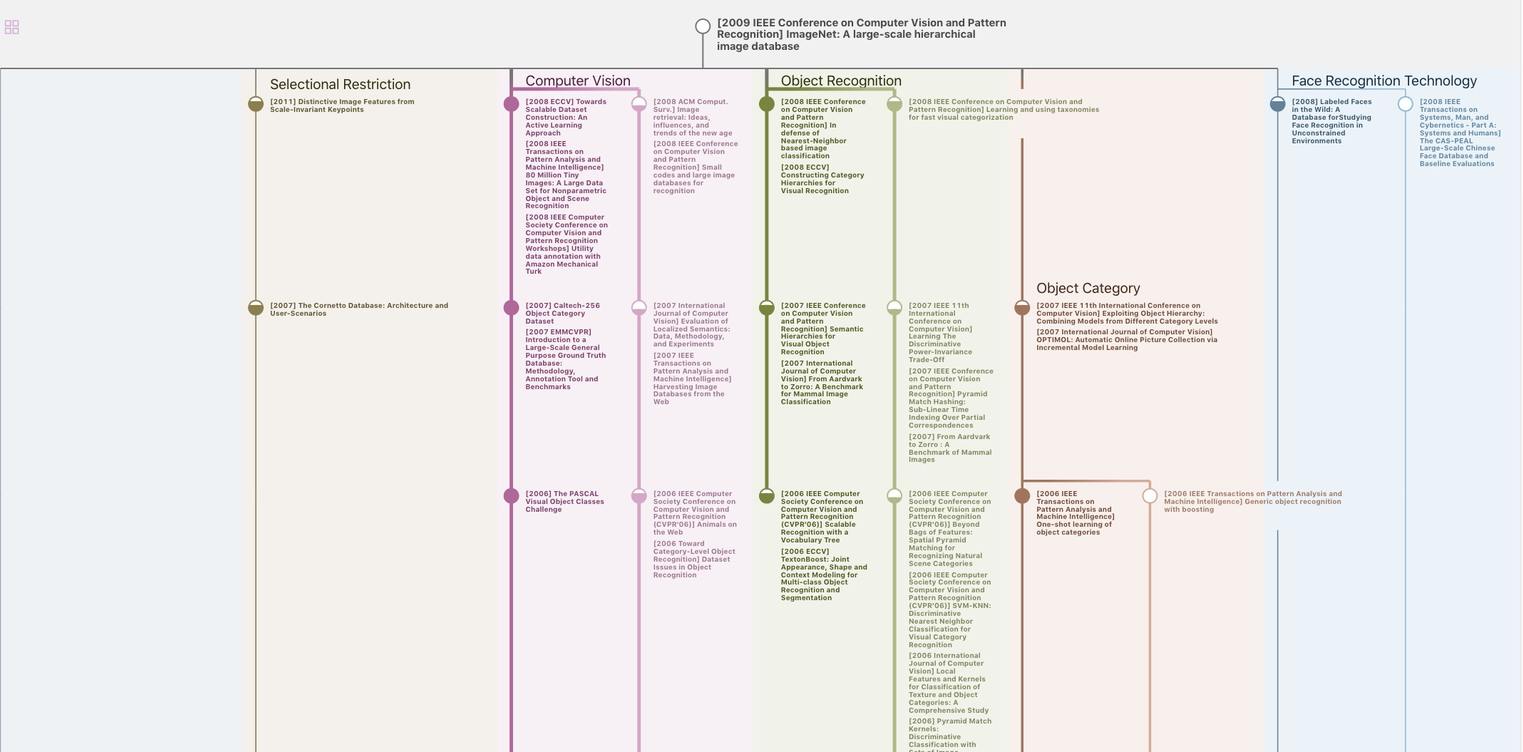
生成溯源树,研究论文发展脉络
Chat Paper
正在生成论文摘要