Machine learning-based decomposition for complex supply chains
Computer-aided chemical engineering(2023)
摘要
Personalised medicine products represent a novel category of therapeutics often characterised by bespoke manufacturing lines and dedicated distribution nodes. An example of such products is Chimeric Antigen Receptor (CAR) T-cells, whose manufacturing poses challenges to volumetric scale-up, leading to increased production and supply chain costs. From a modelling perspective, such networks lead to complex large-scale supply chain models that grow exponentially as the demand increases and more therapies are tracked simultaneously throughout the supply chain. In this work, we present a hybrid model that utilizes the potential of machine learning for strategic planning by forecasting optimal supply chain structures and Mixed Integer Linear Programming (MILP) for detailed scheduling. The proposed model is robust to uncertain demand patterns and can reduce the number of linear constraints and binary variables in the original MILP by more than 64.7%.
更多查看译文
关键词
complex supply chains,decomposition,learning-based
AI 理解论文
溯源树
样例
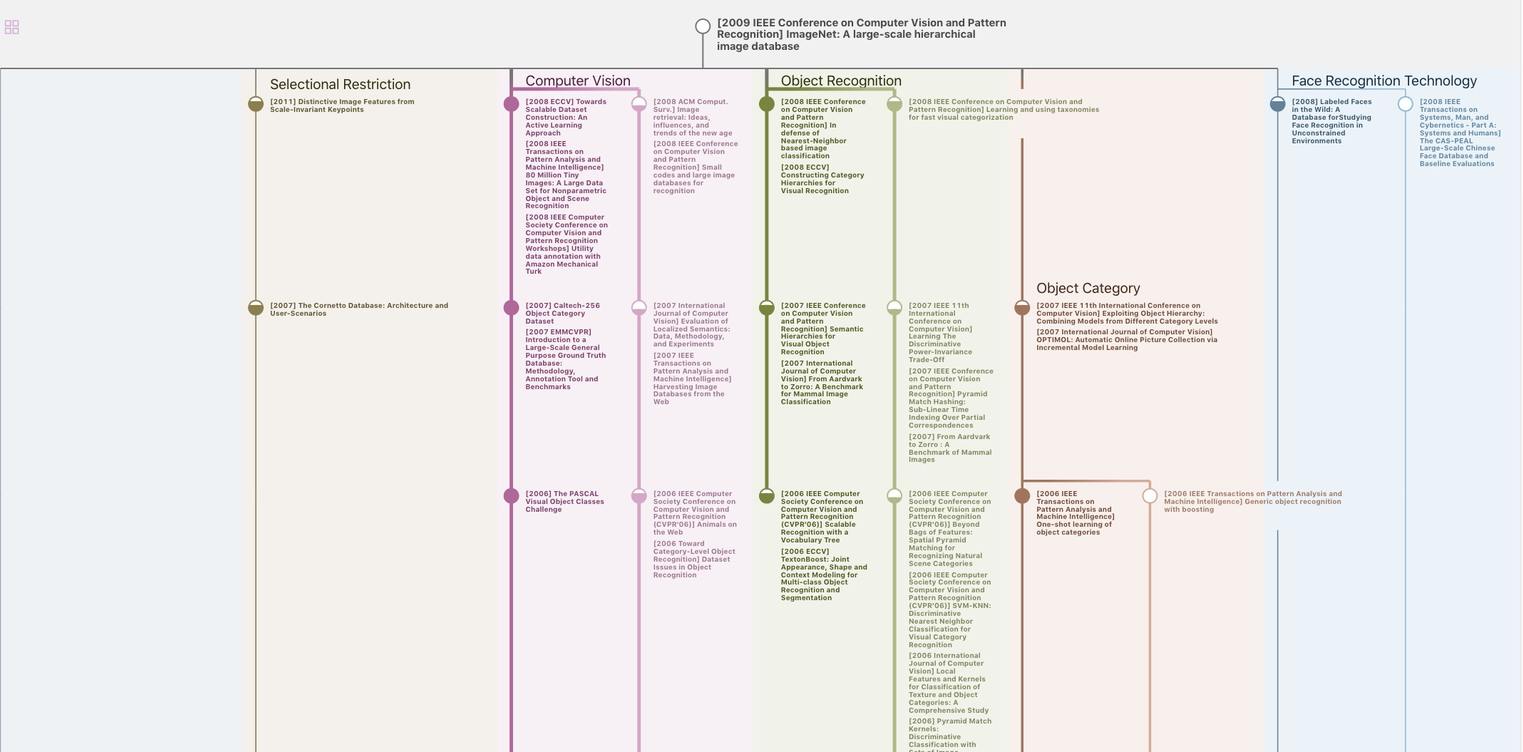
生成溯源树,研究论文发展脉络
Chat Paper
正在生成论文摘要