Acoustic emission source localization using deep transfer learning and finite element modeling-based knowledge transfer
MATERIALS EVALUATION(2023)
摘要
This paper presents a novel data-driven approach to localize two types of acoustic emission sources in an aluminum plate, namely a Hsu-Nielsen source, which simulates a crack-like source, and steel ball impacts of varying diameters acting as the impact source. While deep neural networks have shown promise in previous studies, achieving high accuracy requires a large amount of training data, which may not always be feasible. To address this challenge, we investigated the applicability of transfer learning to address the issue of limited training data. Our approach involves transferring knowledge learned from numerical modeling to the experimental domain to localize nine different source locations. In the process, we evaluated six deep learning architectures using tenfold cross-validation and demonstrated the potential of transfer learning for efficient acoustic emission source localization, even with limited experimental data. This study contributes to the growing demand for running deep learning models with limited capacity and training time and highlights the promise of transfer learning methods such as fine-tuning pretrained models on large semi-related datasets.
更多查看译文
关键词
acoustic emission,deep neural network,finite element modeling,transfer learning,fiber optics,source localization
AI 理解论文
溯源树
样例
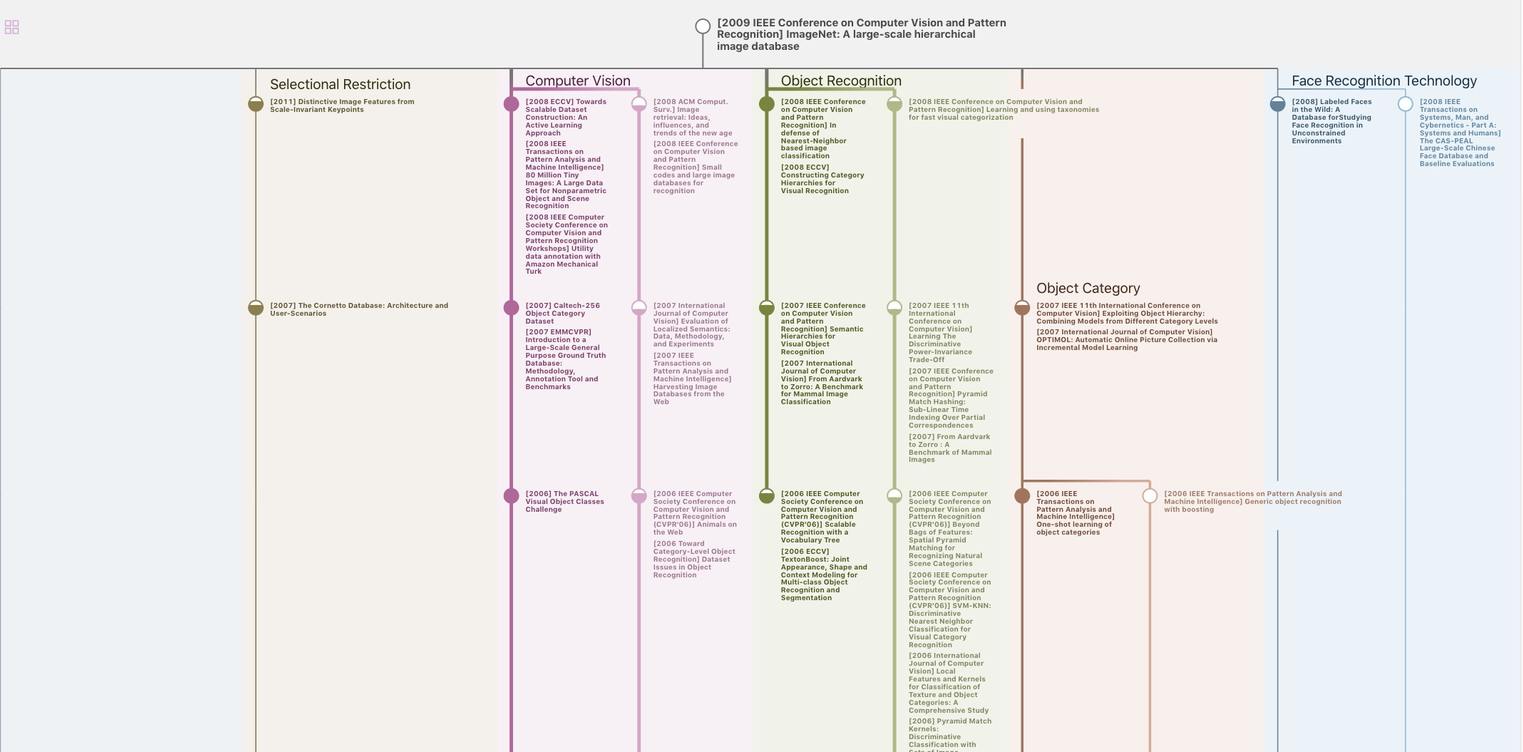
生成溯源树,研究论文发展脉络
Chat Paper
正在生成论文摘要