Otter-Knowledge: benchmarks of multimodal knowledge graph representation learning from different sources for drug discovery
arXiv (Cornell University)(2023)
摘要
Recent research on predicting the binding affinity between drug molecules and proteins use representations learned, through unsupervised learning techniques, from large databases of molecule SMILES and protein sequences. While these representations have significantly enhanced the predictions, they are usually based on a limited set of modalities, and they do not exploit available knowledge about existing relations among molecules and proteins. In this study, we demonstrate that by incorporating knowledge graphs from diverse sources and modalities into the sequences or SMILES representation, we can further enrich the representation and achieve state-of-the-art results for drug-target binding affinity prediction in the established Therapeutic Data Commons (TDC) benchmarks. We release a set of multimodal knowledge graphs, integrating data from seven public data sources, and containing over 30 million triples. Our intention is to foster additional research to explore how multimodal knowledge enhanced protein/molecule embeddings can improve prediction tasks, including prediction of binding affinity. We also release some pretrained models learned from our multimodal knowledge graphs, along with source code for running standard benchmark tasks for prediction of biding affinity.
更多查看译文
关键词
multimodal otter-knowledge graph representation
AI 理解论文
溯源树
样例
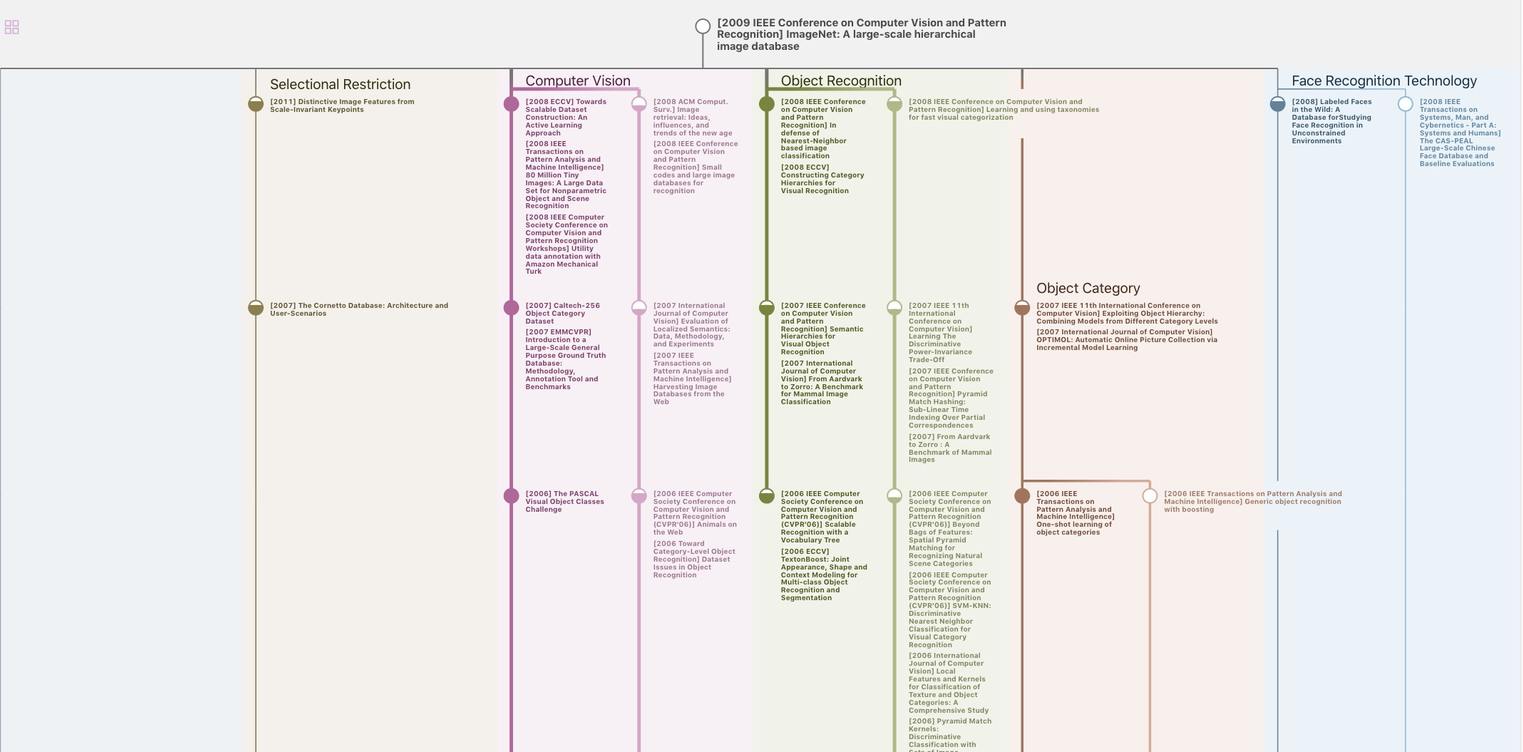
生成溯源树,研究论文发展脉络
Chat Paper
正在生成论文摘要