P-214 The size of the embryo can be used as a single predictor for selecting embryo for transfer
Human Reproduction(2023)
摘要
Abstract Study question What is the performance of the blastocyst area feature compared to the state-of-art AI algorithms in the embryo ranking problem? Summary answer We study a simple embryo selection rule: the larger the better. This rule gives the same quality answers as other methods including AI. What is known already There are many existing methods for scoring embryos starting from the human executed rules, and ending with AI grading systems. Previous studies indicate systematic problems with comparing these methods. This is caused by the lack of a gold standard algorithm, i.e., the repeatable, easy and objective test that would not be susceptible to human interpretation. Common benchmarks typically lead to speeds-up in development of ML methods. We show that the embryo area, a single, interpretable feature, can be used as such a gold standard. It delivers the same quality results (aucroc = 0.66) as existing state-of-art AI algorithms. Study design, size, duration The data-set for retrospective study was collected from a single-center - Kriobank, Bialystok. The data-set includes 1550 time-lapse videos of embryos cultured in an incubator for up to 140 hours post-fertilization with no hatching. All these embryos were transferred with known implantation outcome (beta-hCG). All the videos have been recorded using the same optical magnification setting. Participants/materials, setting, methods Two methods of embryo ranking are compared: - In the first method, the score was equal to the embryo area on the last frame of time-lapse. The area was computed using an AI segmentation algorithm that was trained on hundreds of manually annotated images/frames. - In the second method, state-of-art AI algorithms were designed and trained to grade embryos directly from image data. AUC ROC was used to compare the ranking performance. Main results and the role of chance The proposed gold standard, i.e., the embryo area, requires no training process. It induces the total ranking of all embryos, which should correlate with the treatment outcome, and the way to test it is ROC AUC score. For the whole data-set available (1550 cases) ROC AUC = 0.659 (CI 0.630-0.688), which is relatively high value and compares to the one reported for state-of-art AI algorithms based on deep-learning. We have compared the area-based algorithms with state-of-art machine learning methods used in commercial solutions. In this test we have split the data-set into training (1236 cases) and test subsets (314 cases). The area-based algorithm resulted in ROC AUC = 0.657 (CI 0.597-0.717). For the deep-learning algorithm we have obtained ROC AUC = 0.659 (CI 0.599-0.719). We note that several previous studies have proven relatively high disagreement between embryologists and AI-algorithms. Thus revealing a need for standardization in this area of study, and the need of development of common grounds for tests. The above study strongly suggests that embryo area can serve as a gold standard for further comparison of the developed algorithms, and clear improvement against such gold standards shall be expected. Limitations, reasons for caution • Currently, the application of the embryo area rule is limited to devices with fixed optical magnification. • Further work is needed to compare the segmentation algorithm with manually annotated images. • Despite initial confirmation of the viability the algorithm should be verified and tested on a large, multi-centric data-set. Wider implications of the findings • While AI based tools have the highest potential of increasing the efficacy of embryo selection, there are simple methods that can support embryologists without or with very simple software. • There is a strong need for gold standards that will be further improved. Trial registration number not applicable
更多查看译文
关键词
embryo
AI 理解论文
溯源树
样例
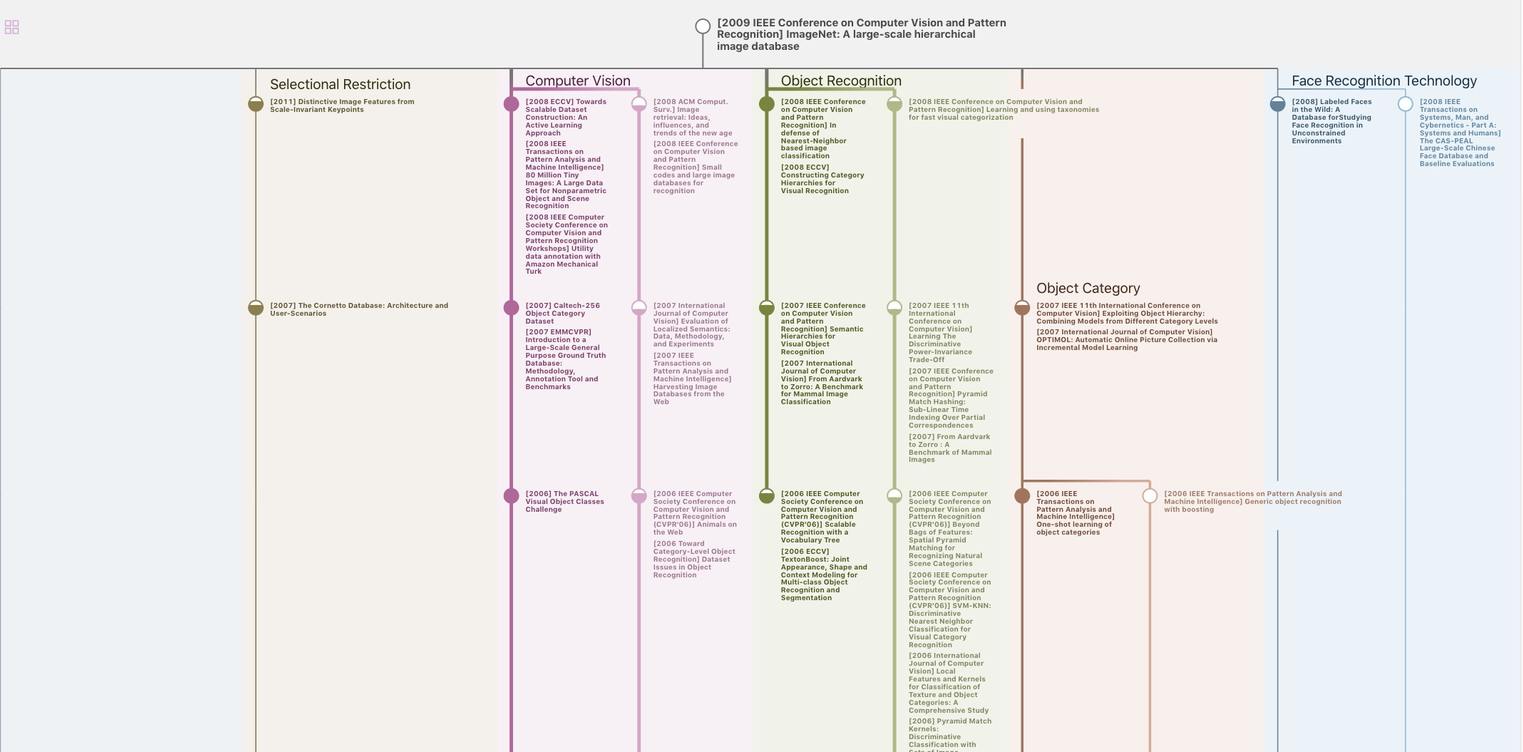
生成溯源树,研究论文发展脉络
Chat Paper
正在生成论文摘要