Robust Reinforcement Learning via Adversarial Kernel Approximation
arXiv (Cornell University)(2023)
摘要
Robust Markov Decision Processes (RMDPs) provide a framework for sequential decision-making that is robust to perturbations on the transition kernel. However, robust reinforcement learning (RL) approaches in RMDPs do not scale well to realistic online settings with high-dimensional domains. By characterizing the adversarial kernel in RMDPs, we propose a novel approach for online robust RL that approximates the adversarial kernel and uses a standard (non-robust) RL algorithm to learn a robust policy. Notably, our approach can be applied on top of any underlying RL algorithm, enabling easy scaling to high-dimensional domains. Experiments in classic control tasks, MinAtar and DeepMind Control Suite demonstrate the effectiveness and the applicability of our method.
更多查看译文
关键词
robust reinforcement learning,adversarial kernel approximation
AI 理解论文
溯源树
样例
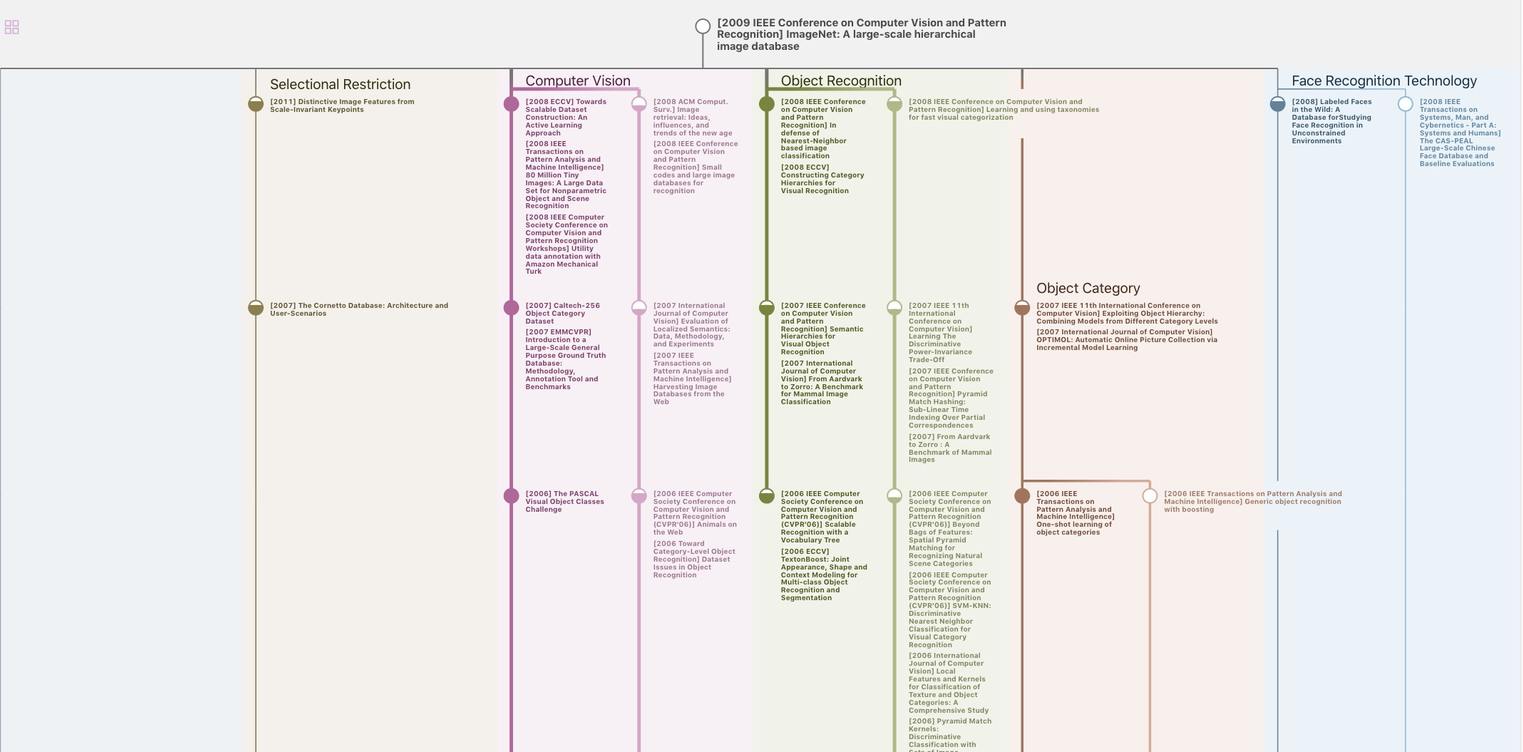
生成溯源树,研究论文发展脉络
Chat Paper
正在生成论文摘要