From Zero to Turbulence: Generative Modeling for 3D Flow Simulation
arXiv (Cornell University)(2023)
摘要
Simulations of turbulent flows in 3D are one of the most expensive simulations in computational fluid dynamics (CFD). Many works have been written on surrogate models to replace numerical solvers for fluid flows with faster, learned, autoregressive models. However, the intricacies of turbulence in three dimensions necessitate training these models with very small time steps, while generating realistic flow states requires either long roll-outs with many steps and significant error accumulation or starting from a known, realistic flow state - something we aimed to avoid in the first place. Instead, we propose to approach turbulent flow simulation as a generative task directly learning the manifold of all possible turbulent flow states without relying on any initial flow state. For our experiments, we introduce a challenging 3D turbulence dataset of high-resolution flows and detailed vortex structures caused by various objects and derive two novel sample evaluation metrics for turbulent flows. On this dataset, we show that our generative model captures the distribution of turbulent flows caused by unseen objects and generates high-quality, realistic samples amenable for downstream applications without access to any initial state.
更多查看译文
关键词
pde,generative,diffusion,navier-stokes,cfd
AI 理解论文
溯源树
样例
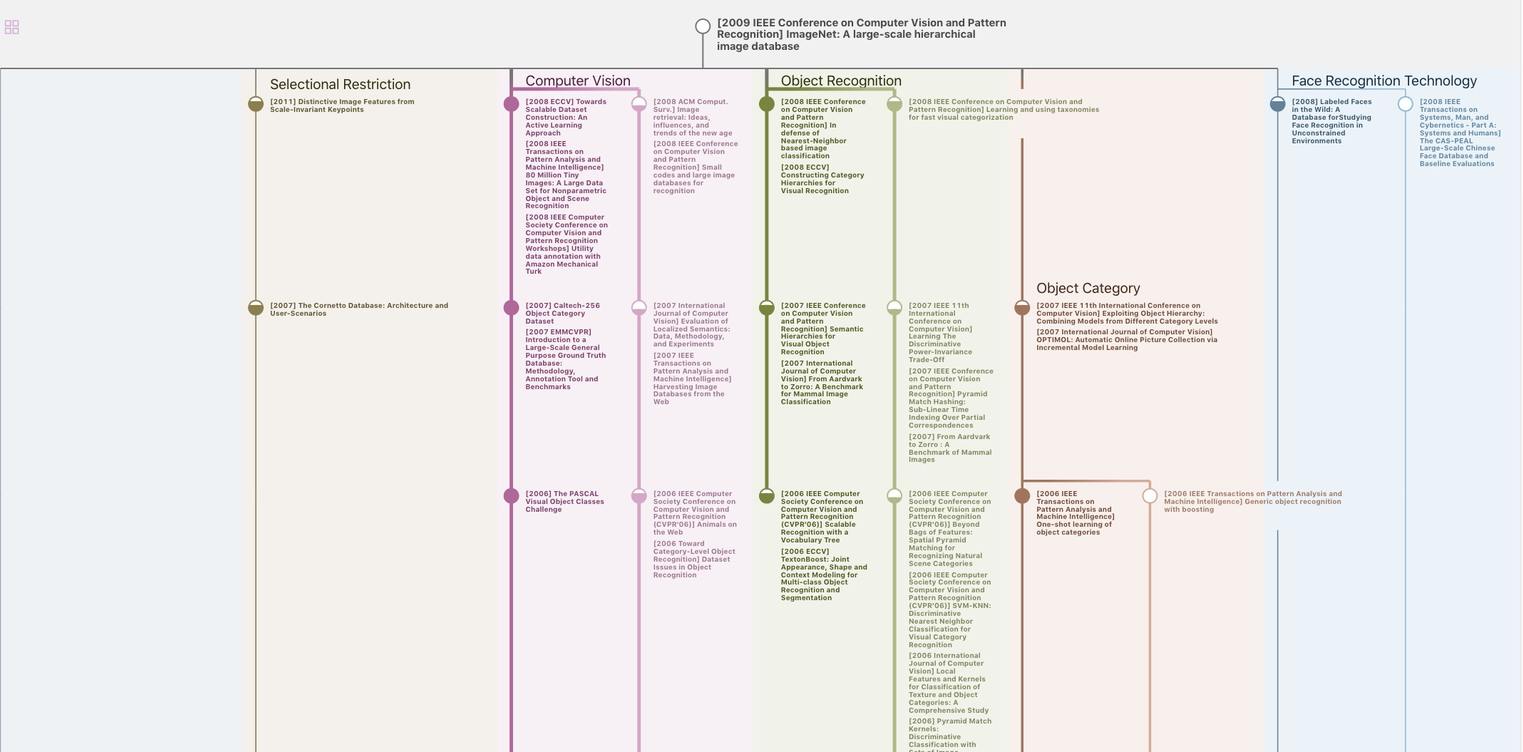
生成溯源树,研究论文发展脉络
Chat Paper
正在生成论文摘要