Image-to-Image Translation With Disentangled Latent Vectors for Face Editing
IEEE TRANSACTIONS ON PATTERN ANALYSIS AND MACHINE INTELLIGENCE(2023)
摘要
We propose an image-to-image translation framework for facial attribute editing with disentangled interpretable latent directions. Facial attribute editing task faces the challenges of targeted attribute editing with controllable strength and disentanglement in the representations of attributes to preserve the other attributes during edits. For this goal, inspired by the latent space factorization works of fixed pretrained GANs, we design the attribute editing by latent space factorization, and for each attribute, we learn a linear direction that is orthogonal to the others. We train these directions with orthogonality constraints and disentanglement losses. To project images to semantically organized latent spaces, we set an encoder-decoder architecture with attention-based skip connections. We extensively compare with previous image translation algorithms and editing with pretrained GAN works. Our extensive experiments show that our method significantly improves over the state-of-the-arts.
更多查看译文
关键词
Image translation,generative adversarial net works,latent space manipulation,face attribute editing
AI 理解论文
溯源树
样例
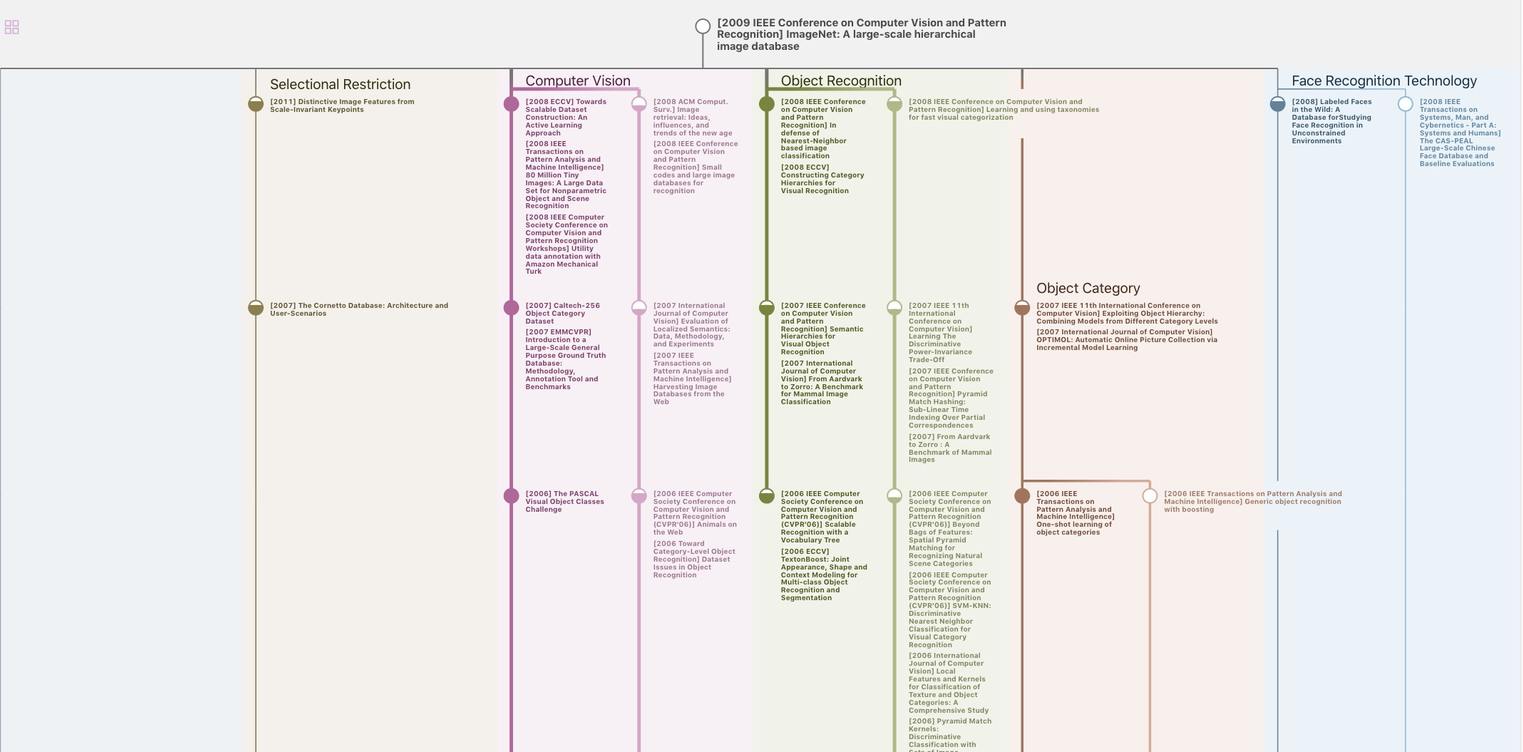
生成溯源树,研究论文发展脉络
Chat Paper
正在生成论文摘要