An analog-AI chip for energy-efficient speech recognition and transcription
NATURE(2023)
摘要
Models of artificial intelligence (AI) that have billions of parameters can achieve high accuracy across a range of tasks 1 , 2 , but they exacerbate the poor energy efficiency of conventional general-purpose processors, such as graphics processing units or central processing units. Analog in-memory computing (analog-AI) 3 – 7 can provide better energy efficiency by performing matrix–vector multiplications in parallel on ‘memory tiles’. However, analog-AI has yet to demonstrate software-equivalent (SW eq ) accuracy on models that require many such tiles and efficient communication of neural-network activations between the tiles. Here we present an analog-AI chip that combines 35 million phase-change memory devices across 34 tiles, massively parallel inter-tile communication and analog, low-power peripheral circuitry that can achieve up to 12.4 tera-operations per second per watt (TOPS/W) chip-sustained performance. We demonstrate fully end-to-end SW eq accuracy for a small keyword-spotting network and near-SW eq accuracy on the much larger MLPerf 8 recurrent neural-network transducer (RNNT), with more than 45 million weights mapped onto more than 140 million phase-change memory devices across five chips.
更多查看译文
关键词
speech recognition,transcription,energy-efficient
AI 理解论文
溯源树
样例
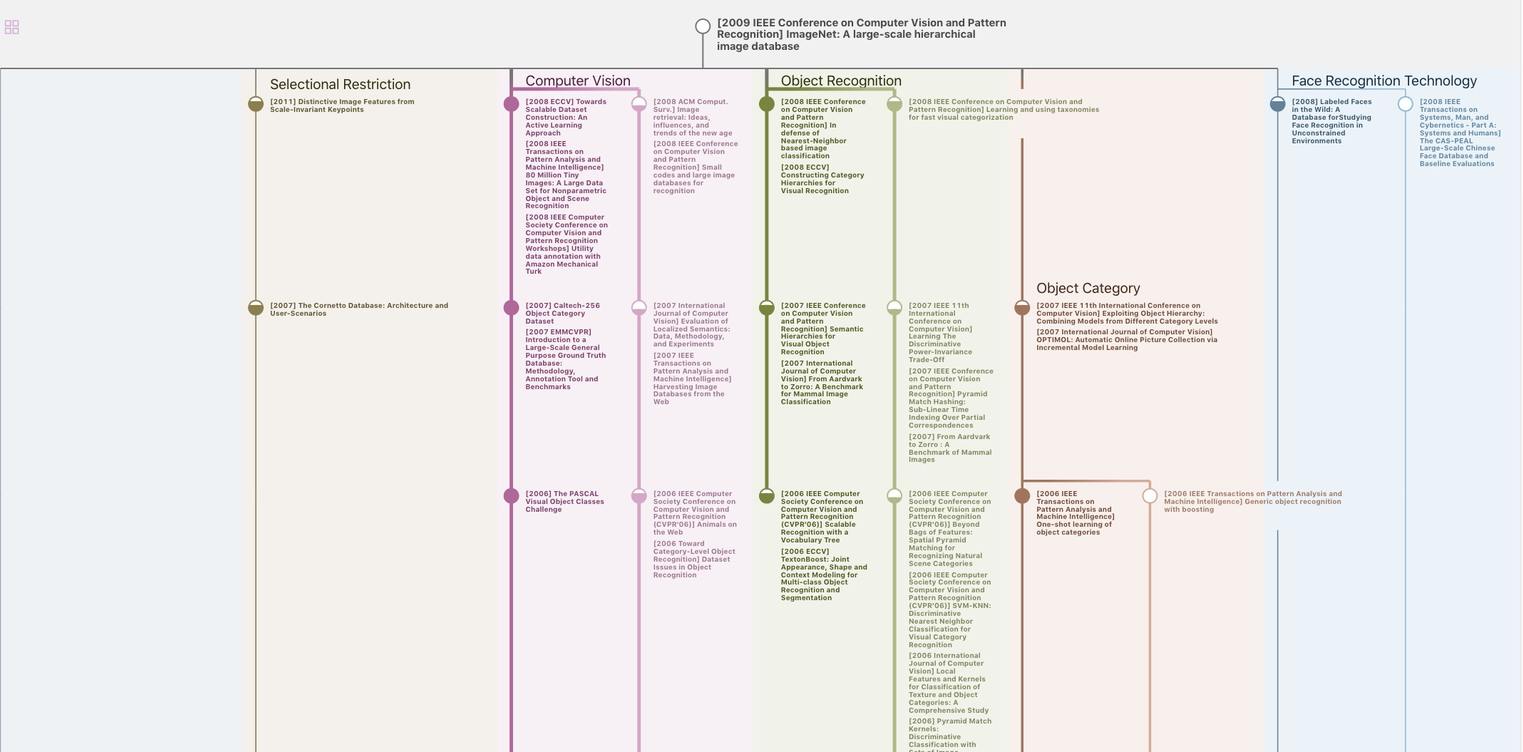
生成溯源树,研究论文发展脉络
Chat Paper
正在生成论文摘要