Fixed-effect and random-effects models in meta-analysis
CHINESE MEDICAL JOURNAL(2024)
摘要
A systematic review synthesizes evidence addressing a structured clinical question using systematic and explicit methods to search, identify, screen, critically appraise, and extract and analyze data from relevant studies.[1] A meta-analysis, on the other hand, is a statistical method that combines the quantitative results of available primary studies addressing the same question to generate a pooled summary estimate and confidence interval (CI).[2] Meta-analyses can increase statistical power, enhance precision, and answer questions that single trials are underpowered to address. Although there are systematic reviews where meta-analyses are not appropriate, when they are well-conducted and reported, systematic reviews combined with meta-analyses provide valuable information for clinicians, researchers, and policymakers. Fifty teachers are enrolled in a study of a new math curriculum. For each teacher, the classes are randomized so that half the classes receive the old curriculum and half receive the new curriculum. The researcher then evaluates the effectiveness of the curricula in optimizing test scores. What is this research trying to address? There are two questions with two underlying assumptions. Question 1. Among these 50 teachers and no others, what is the impact of these two curricula on student examination scores? (Assumption 1: The effect of the new vs. the old curriculum is the same in all teachers.) Question 2. Among all teachers who might ever teach this math course, of whom these 50 teachers are a random sample, what is the impact of these two curricula on student examination scores? (Assumption 2: The effect of the new vs. the old curriculum differs among teachers. For example, some teachers are better suited to the new curriculum than others.) The differences between these two scenarios: In terms of the questions: Are we interested in the effect of the curricula in these 50 teachers or the effect in all teachers? In terms of assumptions: The relative effect of the old vs. the new curricula is the same or different across teachers. Substitute "studies" for teachers and "therapies" for curricula and you get the questions and assumptions for fixed-effect (question 1) and random-effects (question 2) models. Data from eligible studies can be combined in a meta-analysis using either a fixed-effect model or a random-effects model.[3] The choice of model is not straightforward and remains controversial: eminent biostatisticians disagree on the optimal approach. Table 1 presents a summary of the differences between these two models based on conceptual, statistical, and practical considerations. Table 1 - Differences between fixed-effect and random-effects models. Parameters Fixed-effect model Random-effects model Conceptual considerations • Estimates effect in this sample of studies• Assumes effects are the same in all studies • Estimates effect in a population of studies from which the available studies are a random sample• Assumes effects differ across studies and the pooled estimate is the mean effect Statistical considerations • Variance is only derived from within-study variance • Variance is derived from both within-study and between-study variances Practical considerations • Narrow CI• Large studies have much more weight than small studies • Wider CI• Large studies have more weight than small studies, but the gradient is smaller than in fixed-effects models CI: Confidence interval. A fixed-effect model focuses only on the included studies in a meta-analysis and assumes that there is a single true effect size (hence, the term fixed-effect) that is shared by all the included studies. The variation among observed effects is due to random error.[4] Hypothetically, if all studies had an infinite sample size and were completely free of bias, they would generate identical estimates of the effect. This assumes that any differences in the population enrolled, implementation of interventions, the way the outcome was measured, and follow-up length have no (or minimal) impact on the magnitude of the effect. Therefore, the error term in a fixed-effect model comes only from within-study variation. A fixed-effect model aims to estimate this common true effect size and the uncertainty around this estimate. Thus, one can argue that a fixed-effect model is most appropriate when studies have the same design and methodology, or when the variability in results between studies is small, and the variance is thought to be due to random error. A random-effects model assumes that the included studies are a random sample of a population of hypothetical studies. Further, the model assumes no single true effect size exists, but rather the true effect size in each study varies (hence, the term random-effects). Thus, even if all studies had an infinitely large sample size, the observed study effects would still vary because of the real differences in treatment effects across studies.[5] If it was possible to perform a large enough number of studies, the effect estimates of all the studies would follow a normal distribution. The random-effects model takes into account both within-study variability and between-study variability (heterogeneity). The statistical test for heterogeneity addresses the null hypothesis that all studies in a meta-analysis have the same underlying magnitude of effect. The goal of a random-effects model is to estimate not a single effect size but the mean effect size of the intervention across the included studies.[6] Statisticians choose random effects models when the studies are assumed to differ in underlying effects, even if a heterogeneity test that addresses whether chance can explain differences in study results from study to study does not show a significant result (i.e., differences are consistent with chance).[7] The effect of model choice on precision: Under the fixed-effect model, the only source of error in our estimate of the combined effect is the random error within studies. Under the random effects model, we need to take into account two sources of error. So the random-effects model includes both within- and between-study variabilities, and when results vary substantially across studies, the CI of the combined (summary) estimate will be wider [Table 1] than that of a fixed-effect model. In this sense, the random-effects model generally produces a more conservative assessment of the precision of the summary estimate than the fixed-effect model. Figure 1A shows four studies of equal sample size (you can tell because the width of the CI is the same in all four studies). When results do not vary much among studies (i.e., low heterogeneity), the CIs of the two models will be similar or the same. Figure 1B shows hypothetical data with a large amount of variability among studies. As a result, the CI is much wider in the random-effects model than in the fixed-effect model.Figure 1: Hypothetical examples. (A) Minimal variability: Fixed and random models yield identical results. (B) The effect of model choice on precision. (C) The effect of model choice on the point estimate and precision.The effect of model choice on the point estimate: A meta-analysis presents a summary estimate by weighting each study according to the amount of information each study contributes. The weights are calculated based on the variance of each study, which is closely related to the sample size.[8] Generally, larger studies with smaller variance or more precise results will receive larger weight than smaller ones that have larger variance and less precise results.[9] However, while random-effects models give large studies greater weight than smaller studies, the difference in weight between large and small studies is substantially smaller than in fixed-effect models [Table 1]. Thus, the weights assigned under a random-effects model are more balanced between large and small studies, and smaller studies will contribute relatively more to the direction and magnitude of the summary estimate in random-effects than fixed-effect models. Random-effects models, therefore, generate summary estimates closer to the smaller study results than fixed-effect models. Figure 1C shows the effect of small studies on the summary estimate using both models. The point estimate of the random-effects model is closer to small studies than that of the fixed-effect model. Consider a systematic review and meta-analysis that compared calcium channel blockers (CCBs) with other classes of drugs on major cardiovascular events in patients with hypertension.[10] In one comparison, the authors addressed the effect of CCBs vs. beta-blockers. In this comparison, the studies showed very similar results; the variability can be fully explained by chance, heterogeneity P values will be relatively high (P = 0.45), and I2 (which is a measure of the variability among studies and ranges from 0 to 100%) is zero. In this case, the fixed and random effects models will yield identical results [Figure 2A].Figure 2: Real-world examples. (A) Meta-analysis comparing CCBs and beta-blockers on major cardiovascular events in patients with hypertension. (B) Meta-analysis comparing CCBs and ACE inhibitors on major cardiovascular events in patients with hypertension. (C) Meta-analysis comparing restrictive and liberal transfusion on 30-day mortality in patients with anemia and acute myocardial infarction. ACE: Angiotensin-converting enzyme; CCBs: Calcium channel blockers; CI: Confidence interval; RR: Risk ratio.In another comparison between CCBs and angiotensin-converting enzyme (ACE) inhibitors, the heterogeneity is small and I2 is relatively moderate at 45.5%. In such a situation, both models provide similar results [Figure 2B]. But in some other meta-analyses, the heterogeneity is large, and under such a circumstance, these two models would generate quite different results [Figure 2C]. Another real-world example of differences in point estimates and CIs between these two models: You are a physician treating a patient with anemia and acute myocardial infarction. The patient needs a red blood cell transfusion now. You have two transfusion strategies: restrictive transfusion and liberal transfusion. The restrictive transfusion threshold uses a lower hemoglobin concentration as a threshold for transfusion (most commonly, 7.0–8.0 g/dL), and the liberal transfusion threshold uses a higher hemoglobin concentration as a threshold for transfusion (most commonly, 9.0–10.0 g/dL). You are interested in the relative impact of each of the two strategies on 30-day mortality in patients with anemia and acute myocardial infarction. A systematic review and meta-analysis compared these two strategies for mortality in these patients [Figure 2C].[11] This meta-analysis included only three trials.[12–14] Two trials were relatively small (Carson et al[12] with 110 patients and Cooper et al[13] with 44 patients), and one was larger (Ducrocq et al[14] with 666 patients). This analysis was associated with substantial heterogeneity (I2 = 59.1%, P value for the heterogeneity test = 0.09). Under the random-effects model, the point estimate suggests a markedly increased mortality with the restrictive transfusion strategy, but chance can easily explain the difference (risk ratio [RR]: 1.61, 95% CI: 0.38–6.88, P = 0.52). Using the fixed-effect model, the point estimate is very close to the null, and chance can of course easily explain the extremely small difference results (RR: 0.99 with 95% CI: 0.59–1.65 and P = 0.96). From the forest plot, we can see that the points estimate in the random-effects model is closer to the smaller study results and the CI is wider than that of the fixed-effect model. In this case, we are inclined to believe the results of the larger studies. The random-effects model results are therefore misleading. The figure also shows the relative weight of each trial. For example, the largest trial (Ducrocq et al[14]) has a relative weight of 92.62% under the fixed-effect model but 51.00% under the random-effects model. Meta-analysts can choose a fixed-effect or a random-effects model to combine the results in a meta-analysis. If there is minimal heterogeneity among the included studies, these two models will present very similar results. If there is large heterogeneity, the random-effects model will generate wider CI than the fixed-effect model. If the results are different in large and small studies, the point estimate in the random-effects model will be closer to small studies than in the fixed-effect model. Meta-analyses often include trials with substantially different results and, when that is the case, results from fixed and random effects models can differ substantially. The choice between fixed-effect and random-effects models should never be only based on the statistical tests of heterogeneity. Understanding the implications associated with the choice of the model will help clinicians make sense of situations in which large variability in study results exists. Acknowledgments Kameshwar Prasad (Rajendra Institute of Medical Sciences, India) contributed greatly to developing the idea of the analogy. Conflicts of interest None.
更多查看译文
关键词
models,fixed-effect,random-effects,meta-analysis
AI 理解论文
溯源树
样例
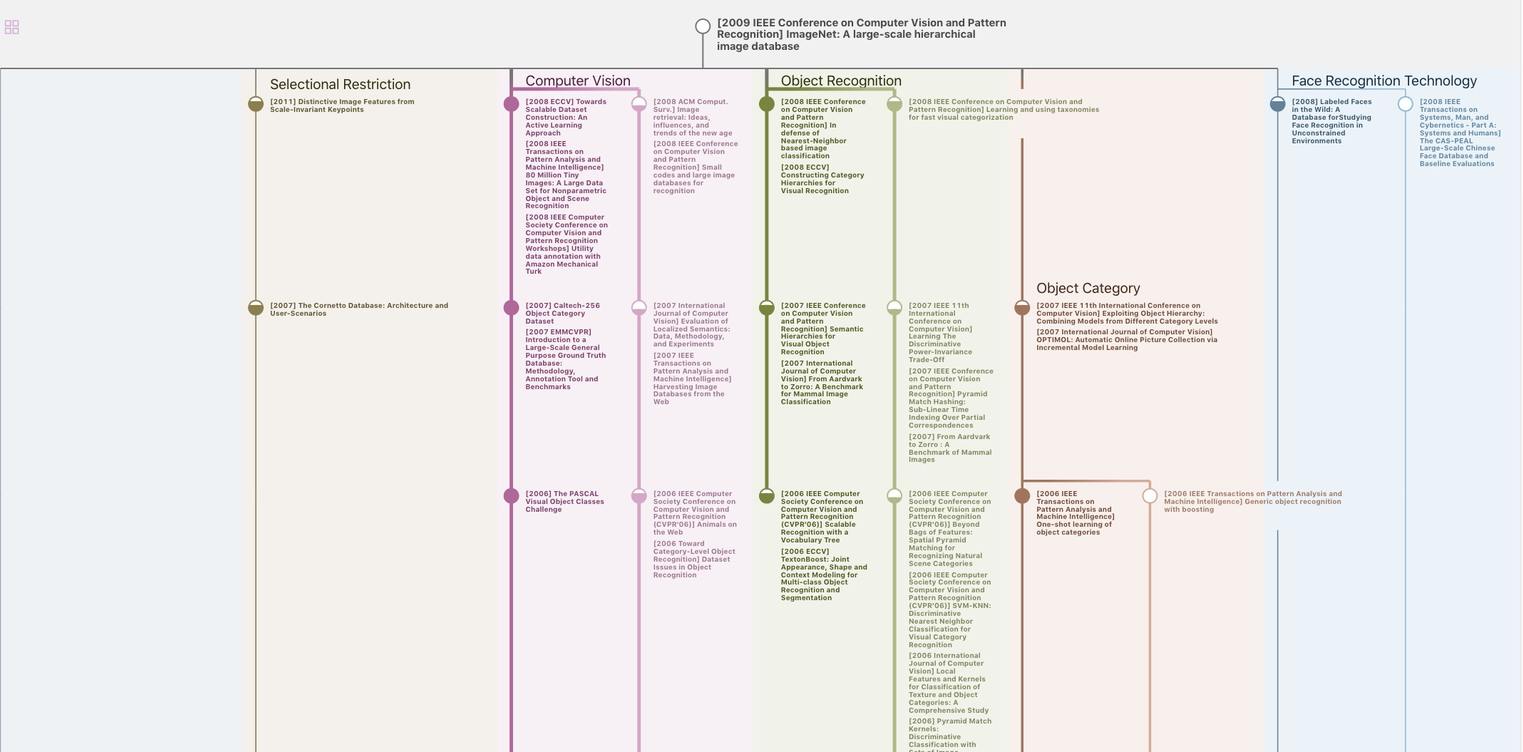
生成溯源树,研究论文发展脉络
Chat Paper
正在生成论文摘要