The Auto-Lindberg Project: Standardized Target Nomenclature in Radiation Oncology Enables Real-World Data Extraction From Radiation Treatment Plans
INTERNATIONAL JOURNAL OF RADIATION ONCOLOGY BIOLOGY PHYSICS(2024)
摘要
To automatically interrogate treatment plans with standardized target nomenclatures to generate real world data on lymph node (LN) involvement in patients with head and neck cancer.Using a large dataset of head and neck treatment plans (TPs), the full dataset including structure names was extracted for analysis and combined with relevant clinical variables. For each patient, the standardized nomenclature describing the LN targets was interrogated to determine the LN levels which were intended for gross nodal/high dose treatment. The dataset was further stratified based on primary site and T-category. The entire dataset and sub-stratified datasets were visualized using a traditional format for ipsilateral and contralateral cervical lymph node regions as well as with summary statistics related to the levels of cervical LN involvement.Of the dataset, 2030 patients had a clinically assigned laterality including 1053 oropharynx (OPC), 552 larynx (LC), 162 nasopharynx (NPC), and 123 hypopharynx (HPC). Patients with OPC had 80% ipsilateral nodal involvement (INI), 33% contralateral nodal involvement (CNI), and 18% retropharyngeal nodal involvement (RNI). Patients with LC had 18% INI, 7% CNI, and 2% retropharyngeal LN. Patients with NPC had 73% INI and 55% CNI, and 64% RNI. Patients with HPC had 72% INI, 28% CNI, and 11% RNI. When stratified by level, patients with OPC, NPC, larynx, and HPC had substantially different proportions of each neck level involved. OPC LN involvement also varied significantly in HPV-positive vs. HPV-negative patients.Routine clinical use of standardized detailed target volume nomenclature including separate LN targets specifying laterality and specific levels of neck involvement allows for automated real world data extraction and visualization. This simple strategy can be easily adopted in any radiation oncology center to develop real world data for any clinical disease site with minimal adaptation.
更多查看译文
AI 理解论文
溯源树
样例
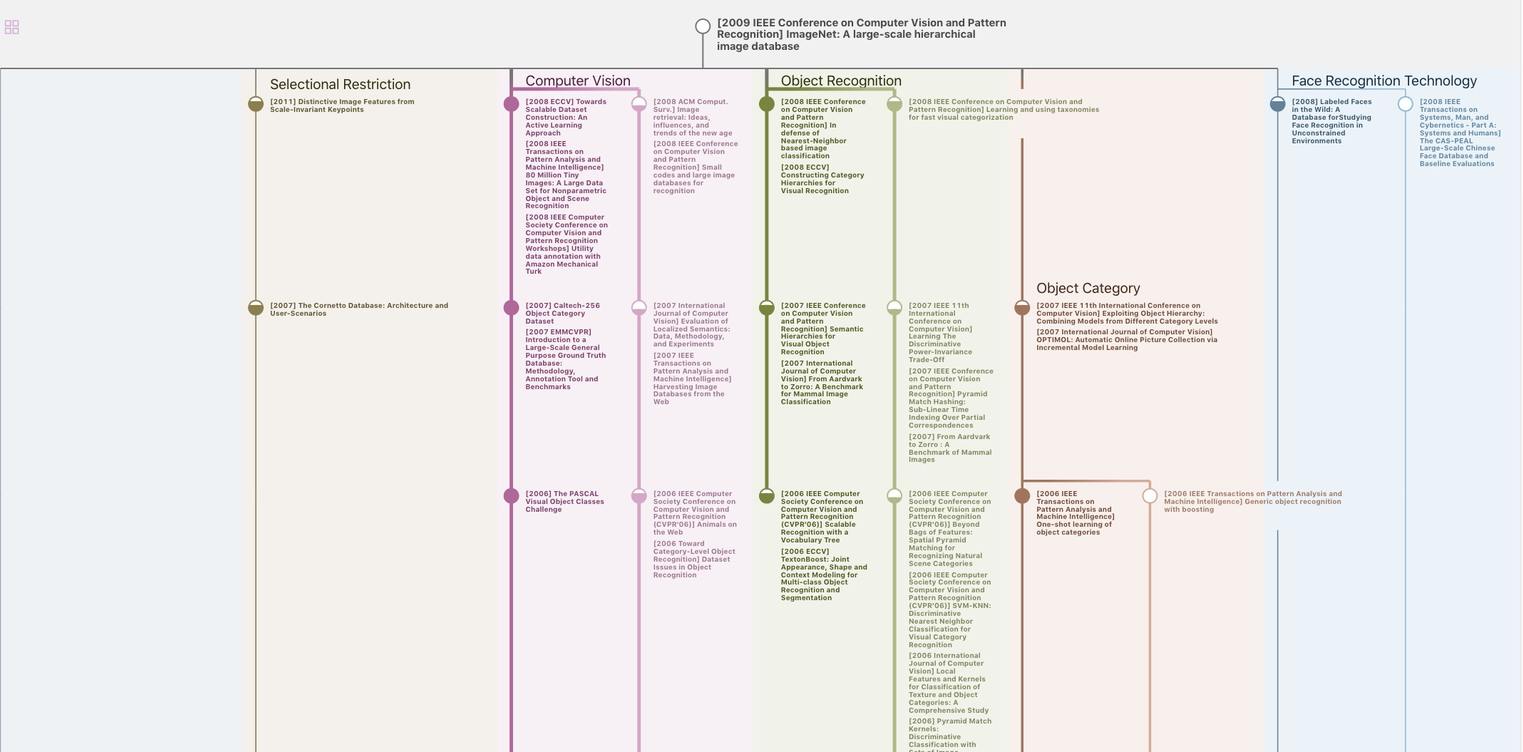
生成溯源树,研究论文发展脉络
Chat Paper
正在生成论文摘要