Collect, Measure, Repeat: Reliability Factors for Responsible AI Data Collection
CoRR(2023)
摘要
The rapid entry of machine learning approaches in our daily activities and high-stakes domains demands transparency and scrutiny of their fairness and reliability. To help gauge machine learning models' robustness, research typically focuses on the massive datasets used for their deployment, e.g., creating and maintaining documentation for understanding their origin, process of development, and ethical considerations. However, data collection for AI is still typically a one-off practice, and oftentimes datasets collected for a certain purpose or application are reused for a different problem. Additionally, dataset annotations may not be representative over time, contain ambiguous or erroneous annotations, or be unable to generalize across issues or domains. Recent research has shown these practices might lead to unfair, biased, or inaccurate outcomes. We argue that data collection for AI should be performed in a responsible manner where the quality of the data is thoroughly scrutinized and measured through a systematic set of appropriate metrics. In this paper, we propose a Responsible AI (RAI) methodology designed to guide the data collection with a set of metrics for an iterative in-depth analysis of the factors influencing the quality and reliability} of the generated data. We propose a granular set of measurements to inform on the internal reliability of a dataset and its external stability over time. We validate our approach across nine existing datasets and annotation tasks and four content modalities. This approach impacts the assessment of data robustness used for AI applied in the real world, where diversity of users and content is eminent. Furthermore, it deals with fairness and accountability aspects in data collection by providing systematic and transparent quality analysis for data collections.
更多查看译文
关键词
data collection,ai,reliability factors
AI 理解论文
溯源树
样例
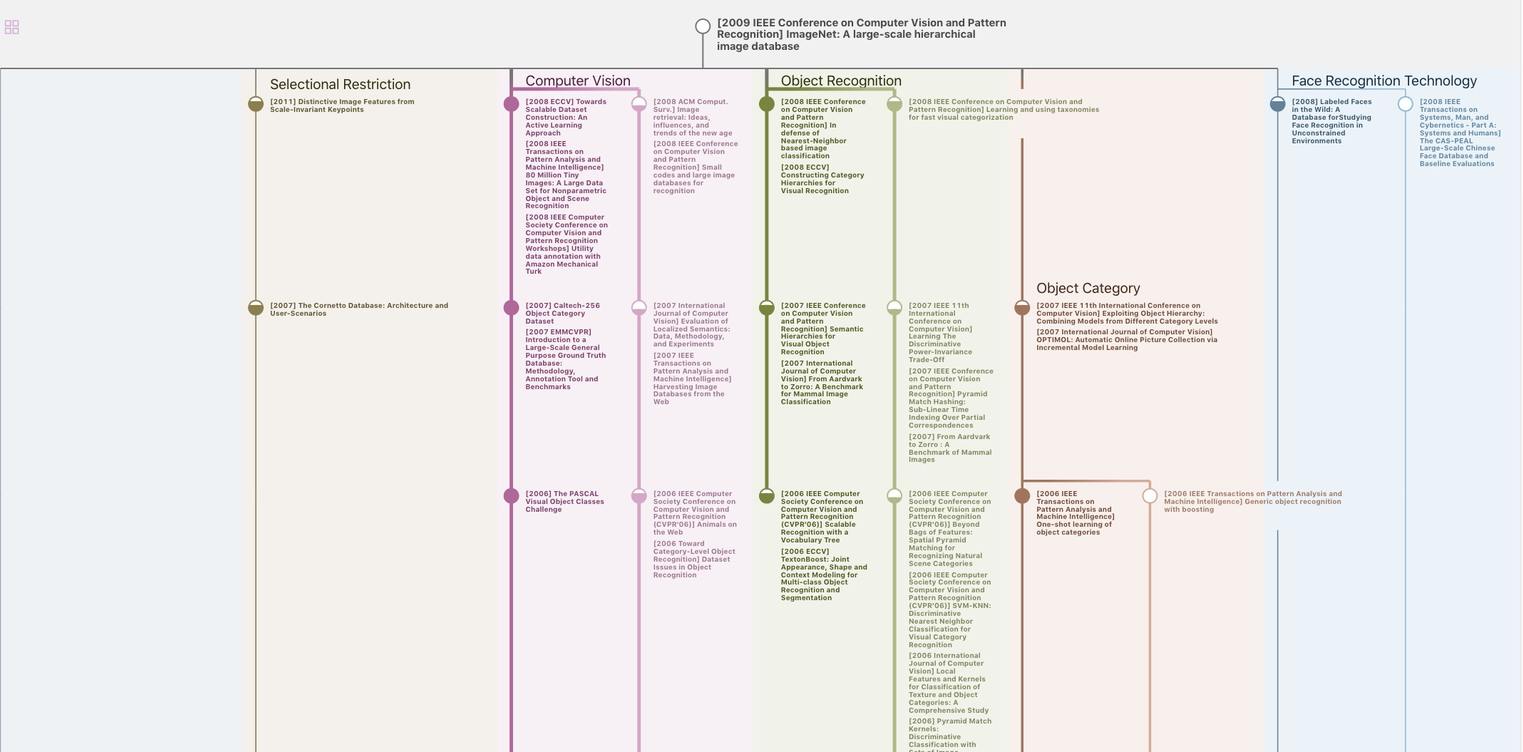
生成溯源树,研究论文发展脉络
Chat Paper
正在生成论文摘要