Acquiring Qualitative Explainable Graphs for Automated Driving Scene Interpretation
CoRR(2023)
摘要
The future of automated driving (AD) is rooted in the development of robust, fair and explainable artificial intelligence methods. Upon request, automated vehicles must be able to explain their decisions to the driver and the car passengers, to the pedestrians and other vulnerable road users and potentially to external auditors in case of accidents. However, nowadays, most explainable methods still rely on quantitative analysis of the AD scene representations captured by multiple sensors. This paper proposes a novel representation of AD scenes, called Qualitative eXplainable Graph (QXG), dedicated to qualitative spatiotemporal reasoning of long-term scenes. The construction of this graph exploits the recent Qualitative Constraint Acquisition paradigm. Our experimental results on NuScenes, an open real-world multi-modal dataset, show that the qualitative eXplainable graph of an AD scene composed of 40 frames can be computed in real-time and light in space storage which makes it a potentially interesting tool for improved and more trustworthy perception and control processes in AD.
更多查看译文
关键词
qualitative explainable graphs,automated driving scene
AI 理解论文
溯源树
样例
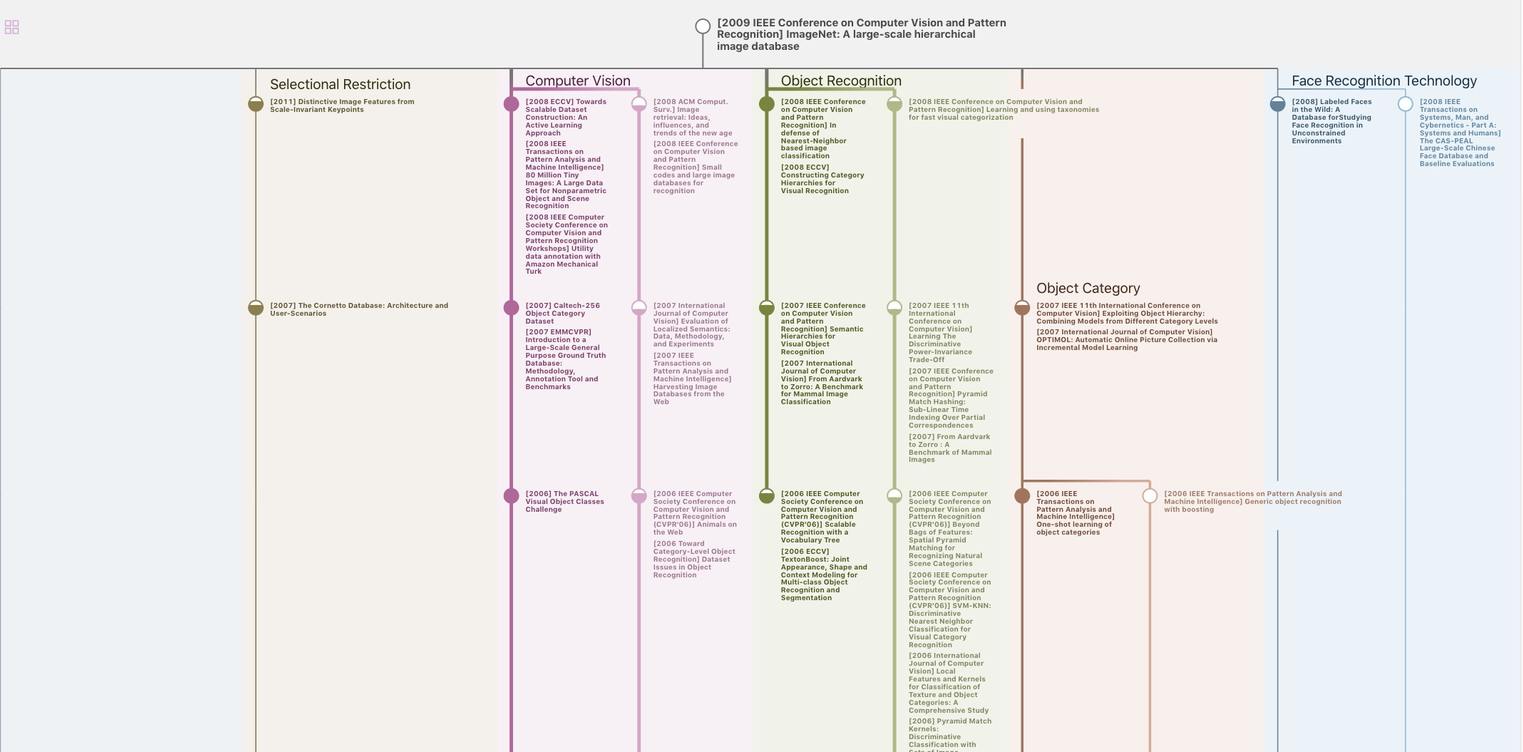
生成溯源树,研究论文发展脉络
Chat Paper
正在生成论文摘要