SayCanPay: Heuristic Planning with Large Language Models Using Learnable Domain Knowledge
AAAI 2024(2024)
摘要
Large Language Models (LLMs) have demonstrated impressive planning abilities due to their vast "world knowledge". Yet, obtaining plans that are both feasible (grounded in affordances) and cost-effective (in plan length), remains a challenge, despite recent progress. This contrasts with heuristic planning methods that employ domain knowledge (formalized in action models such as PDDL) and heuristic search to generate feasible, optimal plans. Inspired by this, we propose to combine the power of LLMs and heuristic planning by leveraging the world knowledge of LLMs and the principles of heuristic search. Our approach, SayCanPay, employs LLMs to generate actions (Say) guided by learnable domain knowledge, that evaluates actions' feasibility (Can) and long-term reward/payoff (Pay), and heuristic search to select the best sequence of actions. Our contributions are (1) a novel framing of the LLM planning problem in the context of heuristic planning, (2) integrating grounding and cost-effective elements into the generated plans, and (3) using heuristic search over actions. Our extensive evaluations show that our model surpasses other LLM planning approaches.
更多查看译文
关键词
PRS: Planning with Language Models
AI 理解论文
溯源树
样例
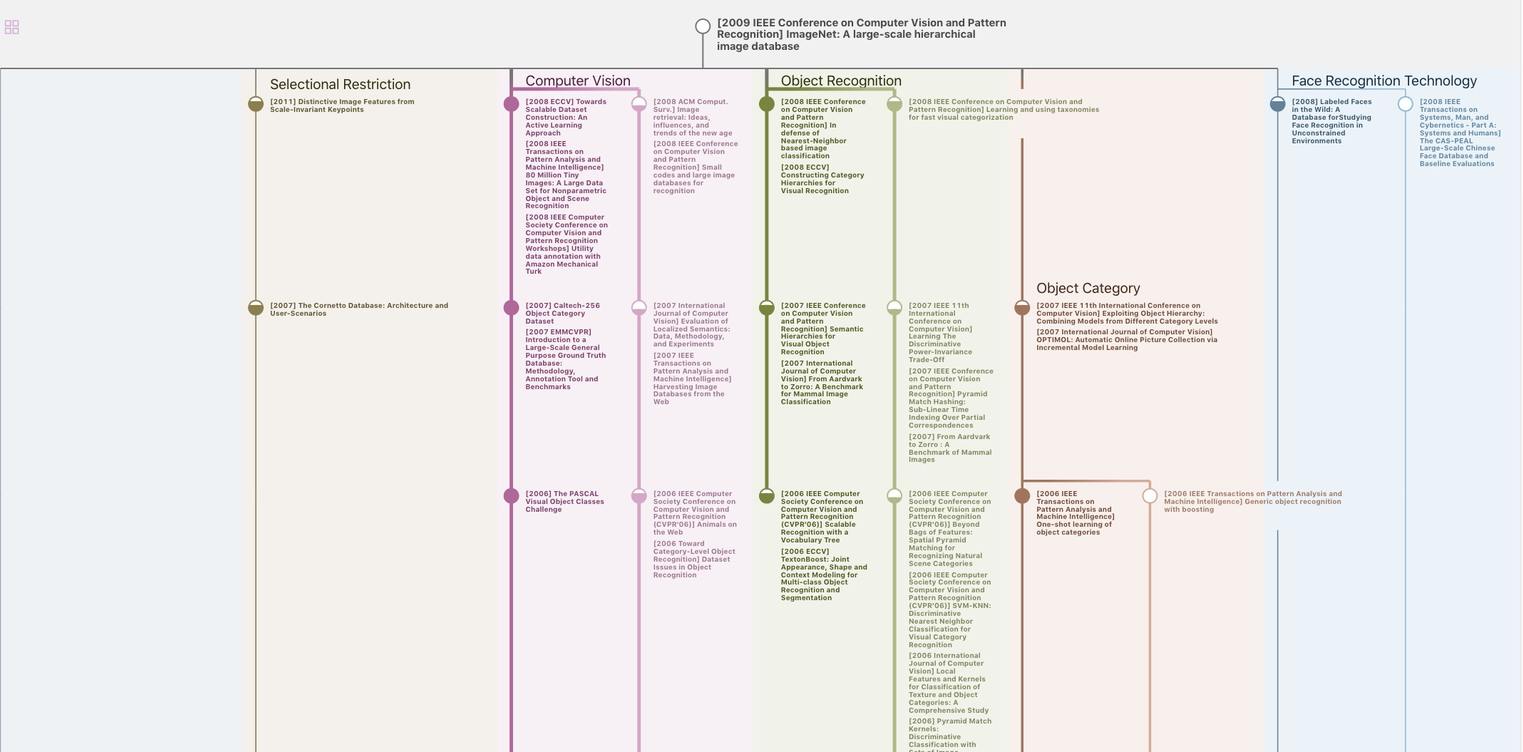
生成溯源树,研究论文发展脉络
Chat Paper
正在生成论文摘要