Self-supervised Learning for Endoscopic Video Analysis
MEDICAL IMAGE COMPUTING AND COMPUTER ASSISTED INTERVENTION, MICCAI 2023, PT V(2023)
摘要
Self-supervised learning (SSL) has led to important break-throughs in computer vision by allowing learning from large amounts of unlabeled data. As such, it might have a pivotal role to play in biomedicine where annotating data requires a highly specialized expertise. Yet, there are many healthcare domains for which SSL has not been extensively explored. One such domain is endoscopy, minimally invasive procedures which are commonly used to detect and treat infections, chronic inflammatory diseases or cancer. In this work, we study the use of a leading SSL framework, namely Masked Siamese Networks (MSNs), for endoscopic video analysis such as colonoscopy and laparoscopy. To fully exploit the power of SSL, we create sizable unlabeled endoscopic video datasets for training MSNs. These strong image representations serve as a foundation for secondary training with limited annotated datasets, resulting in state-of-the-art performance in endoscopic benchmarks like surgical phase recognition during laparoscopy and colonoscopic polyp characterization. Additionally, we achieve a 50% reduction in annotated data size without sacrificing performance. Thus, our work provides evidence that SSL can dramatically reduce the need of annotated data in endoscopy.
更多查看译文
关键词
Artificial intelligence,Self-Supervised Learning,Endoscopy Video Analysis
AI 理解论文
溯源树
样例
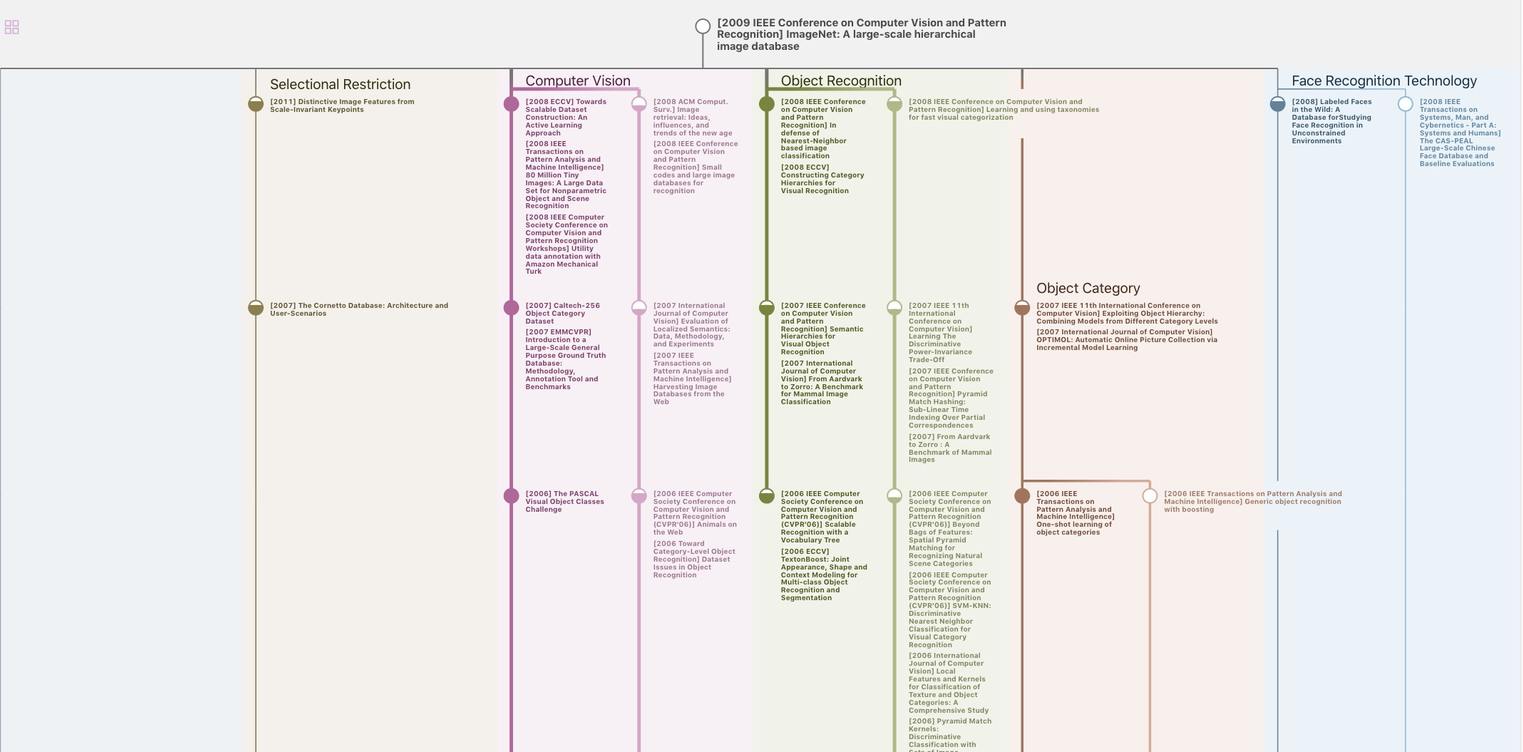
生成溯源树,研究论文发展脉络
Chat Paper
正在生成论文摘要