Improving Generative Model-based Unfolding with Schr\"{o}dinger Bridges
arXiv (Cornell University)(2023)
摘要
Machine learning-based unfolding has enabled unbinned and high-dimensional differential cross section measurements. Two main approaches have emerged in this research area: one based on discriminative models and one based on generative models. The main advantage of discriminative models is that they learn a small correction to a starting simulation while generative models scale better to regions of phase space with little data. We propose to use Schroedinger Bridges and diffusion models to create SBUnfold, an unfolding approach that combines the strengths of both discriminative and generative models. The key feature of SBUnfold is that its generative model maps one set of events into another without having to go through a known probability density as is the case for normalizing flows and standard diffusion models. We show that SBUnfold achieves excellent performance compared to state of the art methods on a synthetic Z+jets dataset.
更多查看译文
关键词
unfolding,bridges,model-based
AI 理解论文
溯源树
样例
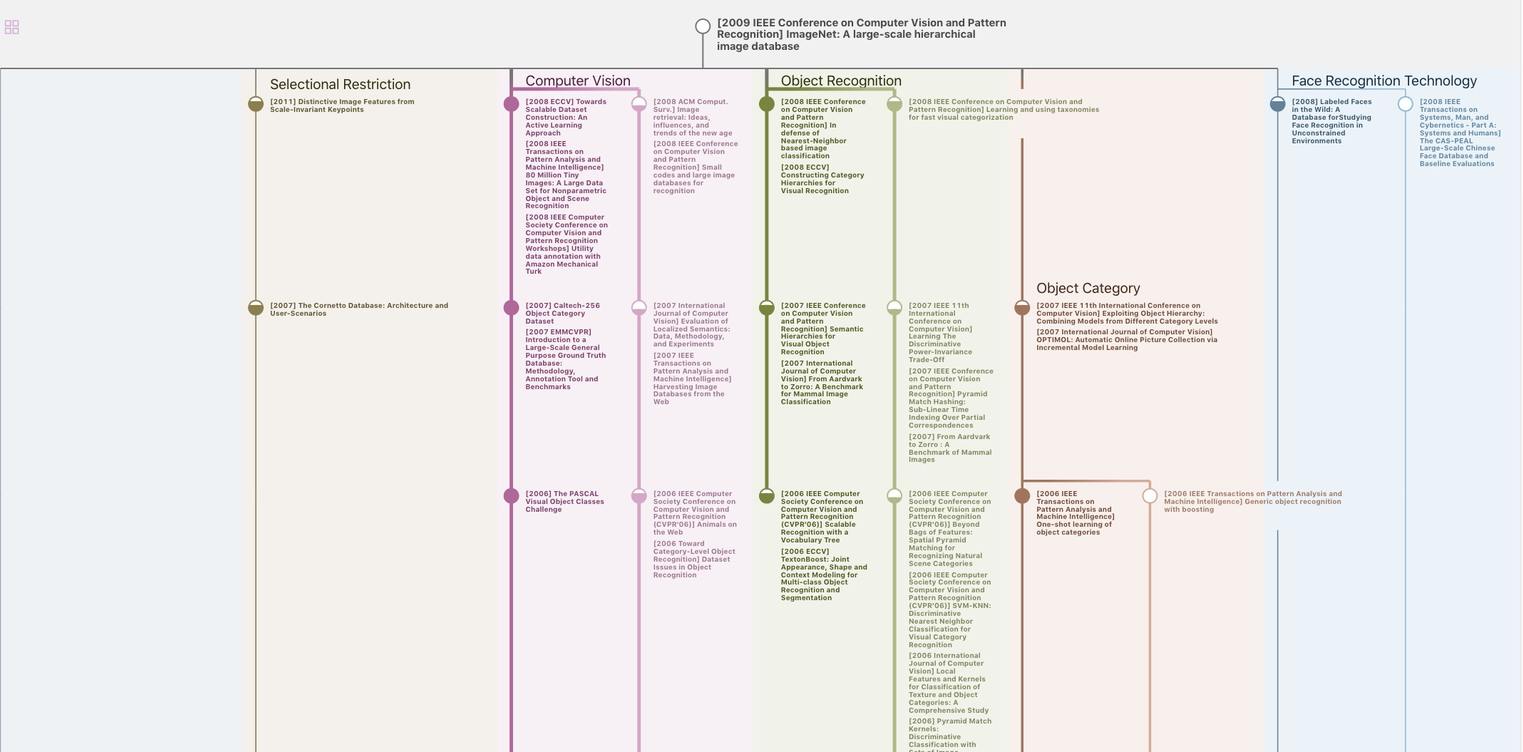
生成溯源树,研究论文发展脉络
Chat Paper
正在生成论文摘要