Adaptive image noise level estimation with Chi-square distribution on the flat patches selected by improved PCANet and ResNet101
Optik(2023)
摘要
Additive Gaussian white noise present in images adversely affects their quality, impeding effective human visualization. Current denoising approaches commonly rely on manual setting of the noise level, leading to insufficient noise removal or excessive smoothing. Traditional noise level estimation methods are not adaptive and their generalization ability is limited. To address this issue, this paper developed an adaptive image noise level estimation with Chi-square distribution on the flat patches selected by improved PCANet and ResNet101, including two key procedures: flat image patches selection and noise level estimation. The former was achieved using the improved PCANet and ResNet101 methods while the latter was completed by applying Chi-square distribution to the selected flat patches. Experimental results demonstrated that the reported method achieved high accuracy and powerful generalization ability, making it more suitable for extensive applications compared to existing methods.
更多查看译文
关键词
Image patches,Additive Gaussian white noise (AGWN) level estimation,Deep learning,Chi-square distribution,AGWN removal
AI 理解论文
溯源树
样例
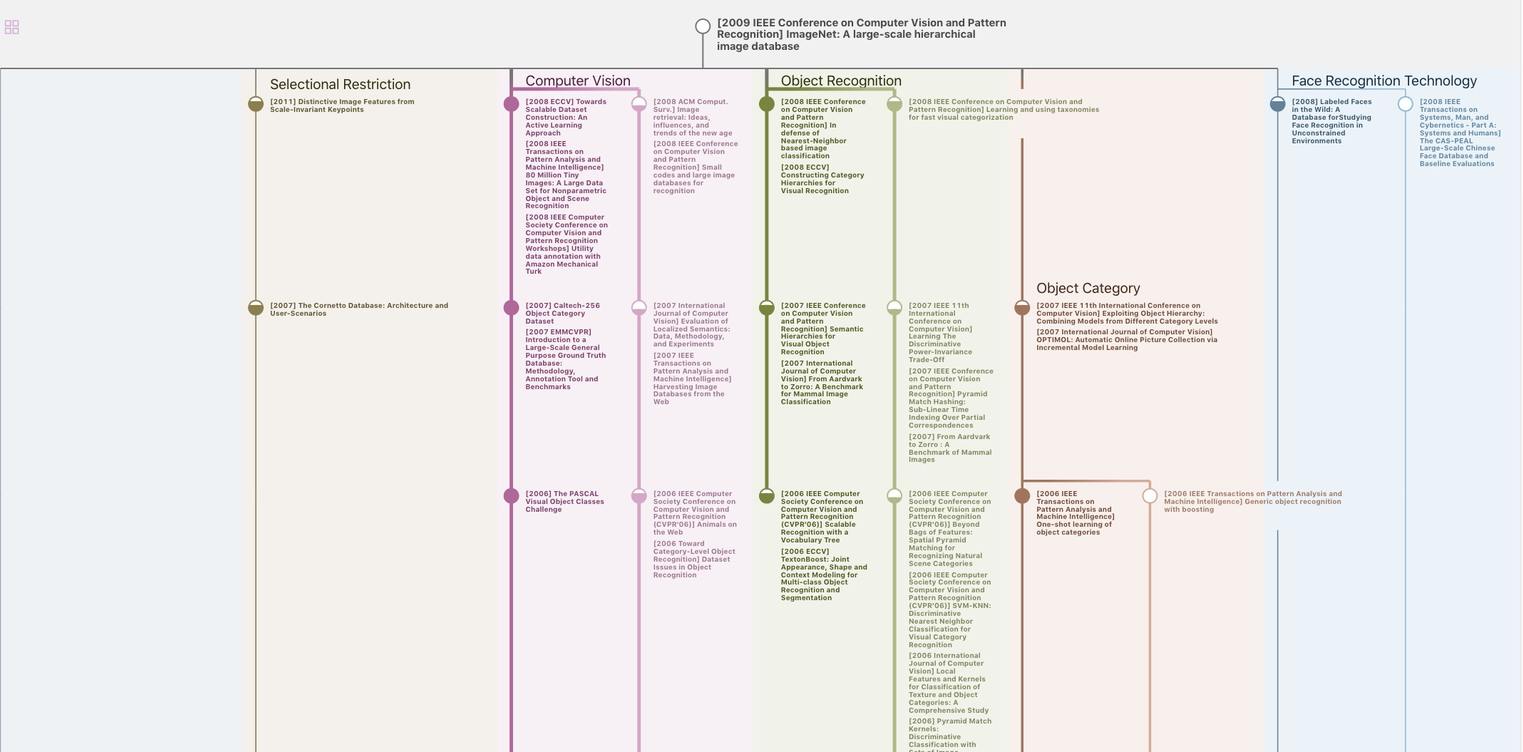
生成溯源树,研究论文发展脉络
Chat Paper
正在生成论文摘要