The Limits of Prompt Engineering in Medical Problem-Solving: A Comparative Analysis with ChatGPT on calculation based USMLE Medical Questions
medRxiv (Cold Spring Harbor Laboratory)(2023)
摘要
Background Prompt engineering significantly improves the performance of Large Language Models (LLMs), including GPT-3.5 and GPT-4. However, its utilization remains largely uncharted in the medical field.
Objective This research aimed to assess the influence of different prompt engineering strategies on ChatGPT (GPT-3.5) in solving medical problems, specifically focusing on medical calculations and clinical scenarios.
Design We utilized three different prompting strategies—direct prompting, the chain of thoughts (CoT), and a modified CoT method—across two sets of USMLE-style questions.
Setting The experiment was conducted using a 1000-question dataset, generated by GPT-4 with a specialized prompt, and a secondary analysis with 95 actual USMLE Step 1 questions.
Measurements Model performance was assessed based on accuracy in answering medical calculation and clinical scenario questions across varying difficulty levels and medical subjects.
Results Direct prompting demonstrated non-inferior accuracy compared to the CoT and modified CoT methods in both question categories. This trend remained consistent regardless of difficulty level or subject matter in the GPT-4-generated dataset and USMLE Step 1 sample questions.
Limitations The study evaluated GPT-3.5 for answering and GPT 4 for question generation, limiting generalizability.
Conclusion Our findings indicate that while prompt engineering can facilitate question generation, as exemplified by GPT-4, it does not necessarily improve model performance in answering medical calculation or clinical scenario questions. This suggests that the ChatGPT model is already effectively optimized for such tasks. Additionally, this finding simplifies the use of such models in healthcare settings, allowing practitioners to interact effectively with tools like ChatGPT without the need for complex prompt engineering, potentially encouraging wider adoption in clinical practice for problem-solving, patient care, and continuous learning.
### Competing Interest Statement
The authors have declared no competing interest.
### Funding Statement
This study did not receive any funding
### Author Declarations
I confirm all relevant ethical guidelines have been followed, and any necessary IRB and/or ethics committee approvals have been obtained.
Yes
I confirm that all necessary patient/participant consent has been obtained and the appropriate institutional forms have been archived, and that any patient/participant/sample identifiers included were not known to anyone (e.g., hospital staff, patients or participants themselves) outside the research group so cannot be used to identify individuals.
Yes
I understand that all clinical trials and any other prospective interventional studies must be registered with an ICMJE-approved registry, such as ClinicalTrials.gov. I confirm that any such study reported in the manuscript has been registered and the trial registration ID is provided (note: if posting a prospective study registered retrospectively, please provide a statement in the trial ID field explaining why the study was not registered in advance).
Yes
I have followed all appropriate research reporting guidelines, such as any relevant EQUATOR Network research reporting checklist(s) and other pertinent material, if applicable.
Yes
All data produced in the present study are available upon reasonable request to the authors
更多查看译文
关键词
prompt engineering,chatgpt,questions,problem-solving
AI 理解论文
溯源树
样例
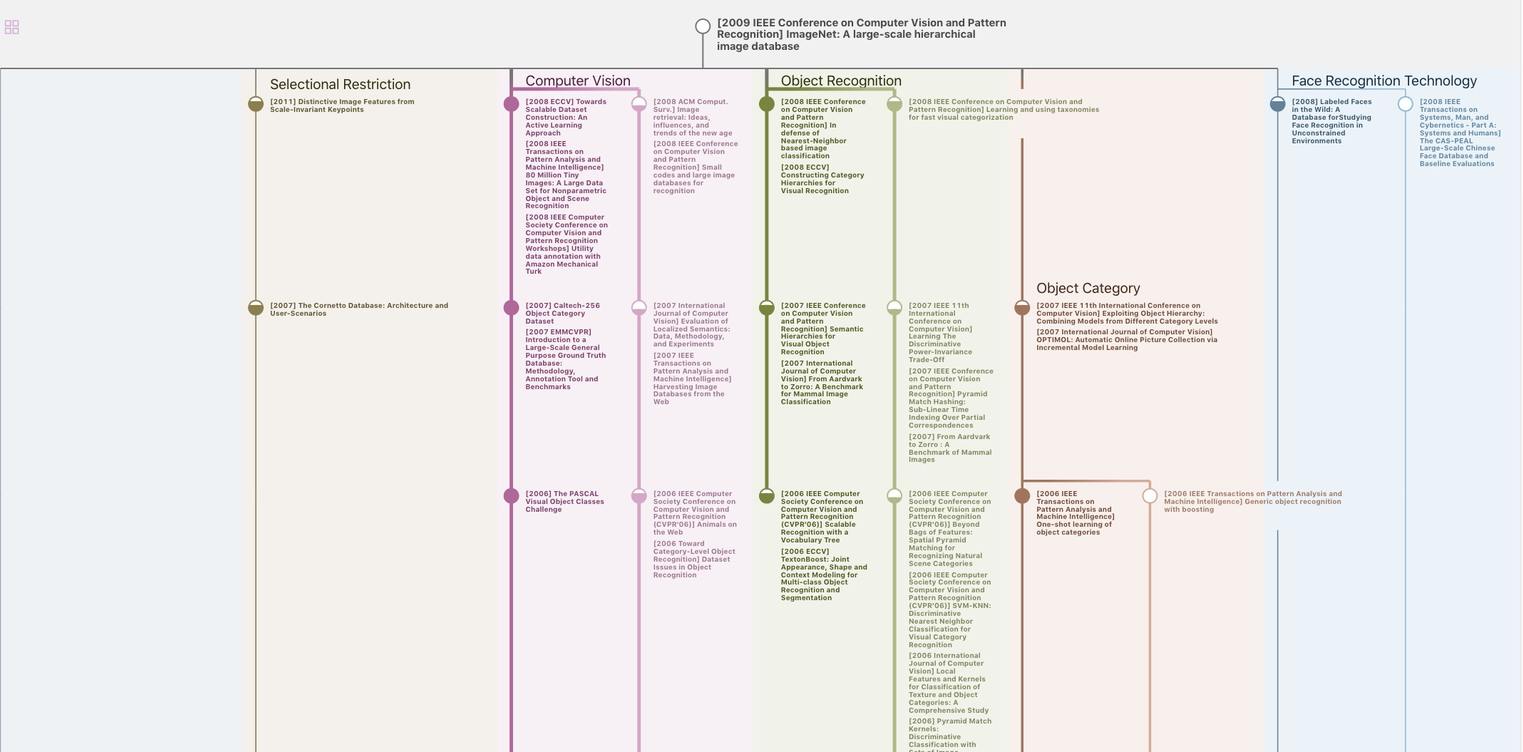
生成溯源树,研究论文发展脉络
Chat Paper
正在生成论文摘要