Deep learning-based rigid motion correction for magnetic resonance imaging: A survey
Meta-Radiology(2023)
摘要
Physiological and physical motions of the subjects, e.g., patients, are the primary sources of image artifacts in magnetic resonance imaging (MRI), causing geometric distortion, blurring, low signal-to-noise ratio, or ghosting. To overcome motion artifacts, various deep learning strategies, and models have been investigated to enable retrospective and prospective motion correction for MRI. This review article provides a survey on current deep learning-based rigid motion correction methods that have been used for MRI. Also, deep learning motion correction methods are compared to conventional motion correction methods and hybrid methods. Furthermore, we discuss the advantages and limitations of the current deep learning motion correction methods, leading to some suggestions for the future development of deep learning motion correction methods and their potential applications in improving clinical MRI.
更多查看译文
关键词
Magnetic resonance imaging,Motion correction,Deep learning,Imaging process
AI 理解论文
溯源树
样例
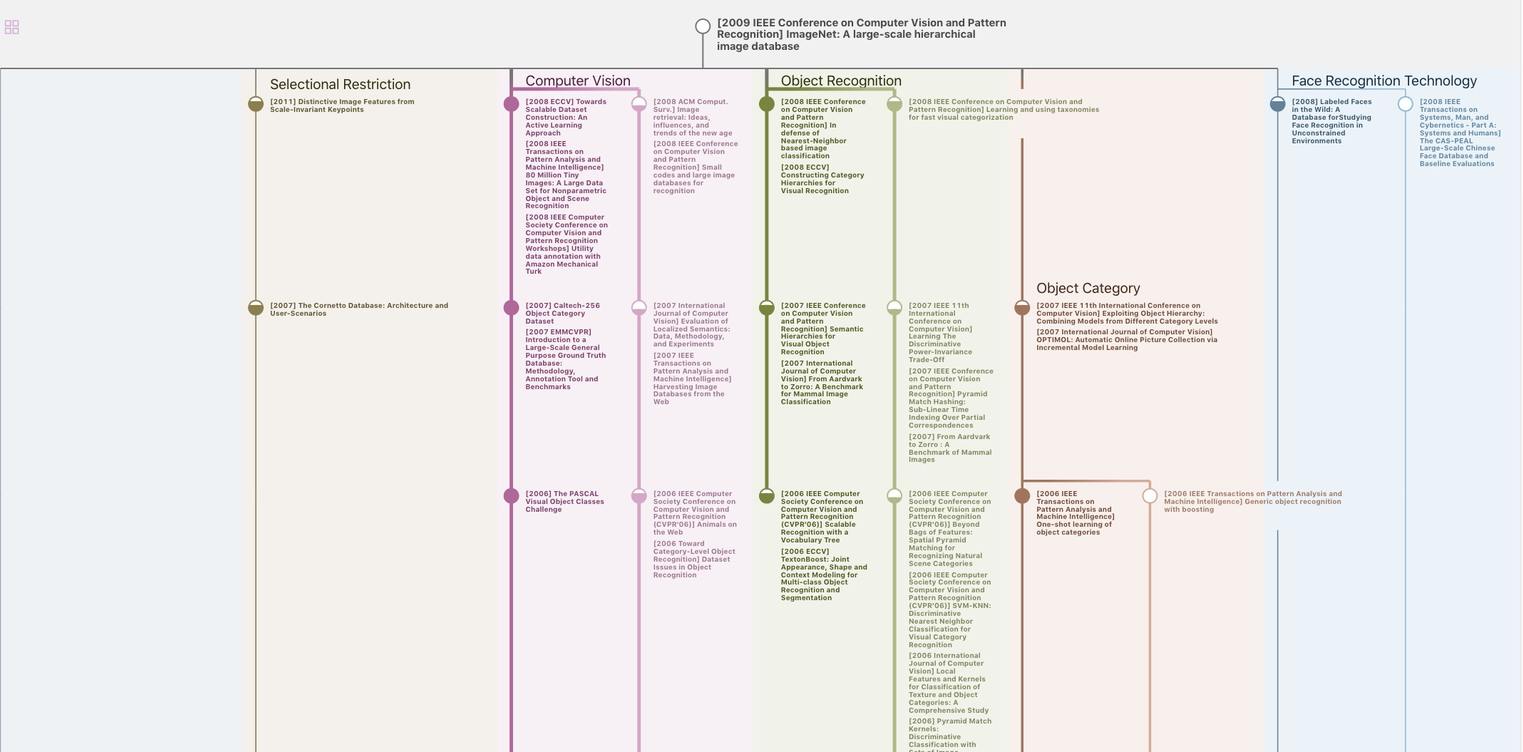
生成溯源树,研究论文发展脉络
Chat Paper
正在生成论文摘要