Joint clinical and molecular subtyping of COPD with variational autoencoders.
medRxiv : the preprint server for health sciences(2024)
摘要
Chronic Obstructive Pulmonary Disease (COPD) is a complex, heterogeneous disease. Traditional subtyping methods generally focus on either the clinical manifestations or the molecular endotypes of the disease, resulting in classifications that do not fully capture the disease's complexity. Here, we bridge this gap by introducing a subtyping pipeline that integrates clinical and gene expression data with variational autoencoders. We apply this methodology to the COPDGene study, a large study of current and former smoking individuals with and without COPD. Our approach generates a set of vector embeddings, called Personalized Integrated Profiles (PIPs), that recapitulate the joint clinical and molecular state of the subjects in the study. Prediction experiments show that the PIPs have a predictive accuracy comparable to or better than other embedding approaches. Using trajectory learning approaches, we analyze the main trajectories of variation in the PIP space and identify five well-separated subtypes with distinct clinical phenotypes, expression signatures, and disease outcomes. Notably, these subtypes are more robust to data resampling compared to those identified using traditional clustering approaches. Overall, our findings provide new avenues to establish fine-grained associations between the clinical characteristics, molecular processes, and disease outcomes of COPD.
更多查看译文
关键词
copd,molecular subtyping
AI 理解论文
溯源树
样例
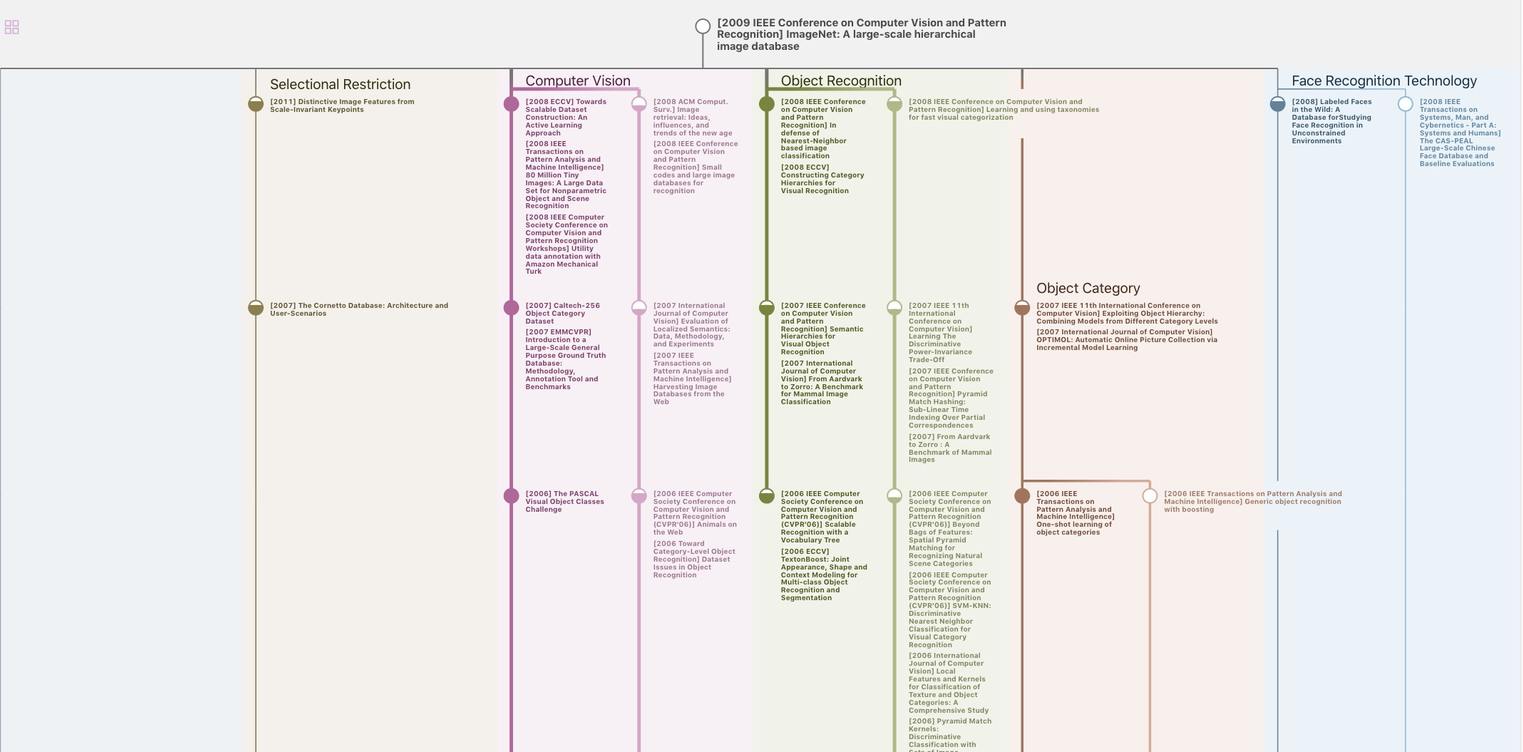
生成溯源树,研究论文发展脉络
Chat Paper
正在生成论文摘要