Pretrained transformer models for predicting the withdrawal of drugs from the market
BIOINFORMATICS(2023)
摘要
Motivation: The process of drug discovery is notoriously complex, costing an average of 2.6 billion dollars and taking similar to 13 years to bring a new drug to the market. The success rate for new drugs is alarmingly low (around 0.0001%), and severe adverse drug reactions (ADRs) frequently occur, some of which may even result in death. Early identification of potential ADRs is critical to improve the efficiency and safety of the drug development process. Results: In this study, we employed pretrained large language models (LLMs) to predict the likelihood of a drug being withdrawn from the market due to safety concerns. Our method achieved an area under the curve (AUC) of over 0.75 through cross-database validation, outperforming classical machine learning models and graph-based models. Notably, our pretrained LLMs successfully identified over 50% drugs that were subsequently withdrawn, when predictions were made on a subset of drugs with inconsistent labeling between the training and test sets. Availability and implementation: The code and datasets are available at https://github.com/eyalmazuz/DrugWithdrawn.
更多查看译文
AI 理解论文
溯源树
样例
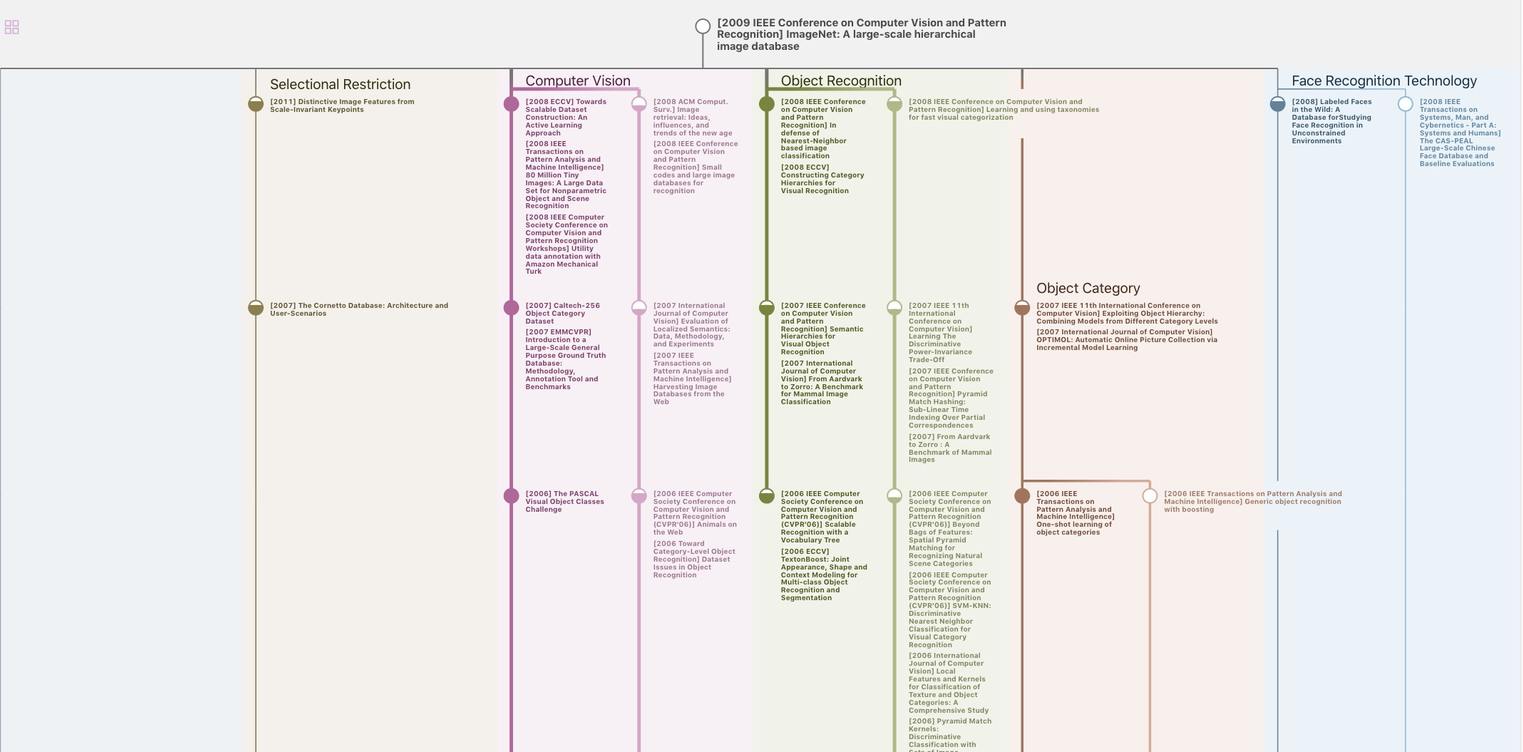
生成溯源树,研究论文发展脉络
Chat Paper
正在生成论文摘要