Benchmark Pathology Report Text Corpus with Cancer Type Classification.
medRxiv : the preprint server for health sciences(2023)
摘要
In cancer research, pathology report text is a largely un-tapped data source. Pathology reports are routinely generated, more nuanced than structured data, and contain added insight from pathologists. However, there are no publicly-available datasets for benchmarking report-based models. Two recent advances suggest the urgent need for a benchmark dataset. First, improved optical character recognition (OCR) techniques will make it possible to access older pathology reports in an automated way, increasing data available for analysis. Second, recent improvements in natural language processing (NLP) techniques using AI allow more accurate prediction of clinical targets from text. We apply state-of-the-art OCR and customized post- processing to publicly available report PDFs from The Cancer Genome Atlas, generating a machine-readable corpus of 9,523 reports. We perform a proof-of-principle cancer-type classification across 32 tissues, achieving 0.992 average AU-ROC. This dataset will be useful to researchers across specialties, including research clinicians, clinical trial investigators, and clinical NLP researchers.
更多查看译文
关键词
cancer type classification,corpus,pathology
AI 理解论文
溯源树
样例
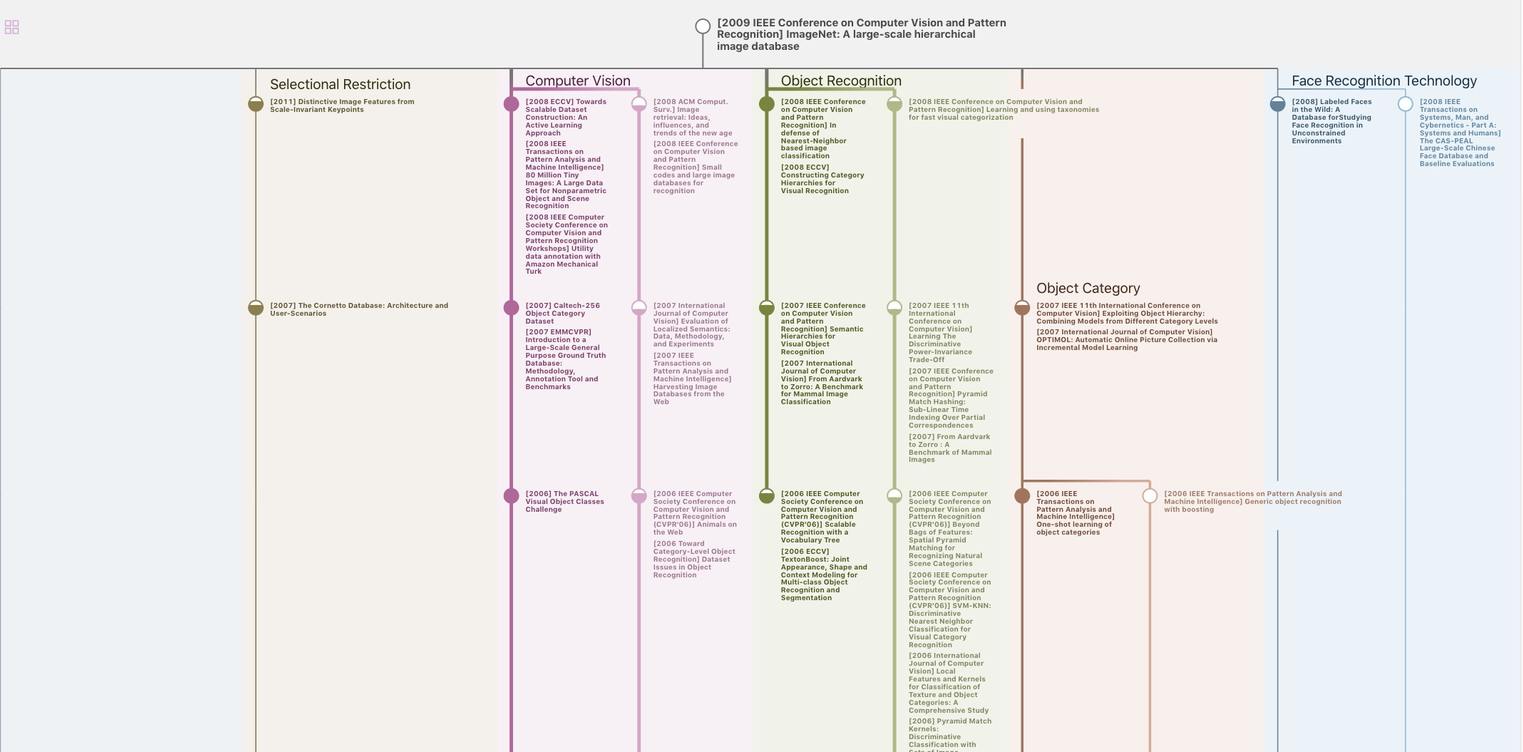
生成溯源树,研究论文发展脉络
Chat Paper
正在生成论文摘要