HexNet: An Orientation-Aware Deep Learning Framework for Omni-Directional Input
IEEE TRANSACTIONS ON PATTERN ANALYSIS AND MACHINE INTELLIGENCE(2023)
摘要
While omni-directional sensors provide holistic representations typical deep learning frameworks reduce the benefits by introducing distortions and discontinuities as spherical data is supplied as planar input. On the other hand, recent spherical convolutional neural networks (CNNs) often require significant memory and parameters, thus enabling execution only at very low resolutions and shallow architectures. We propose HexNet, an orientation-aware deep learning framework for spherical signals, that allows for fast computation as we exploit standard planar network operations on an efficiently arranged projection of the sphere. Furthermore, we introduce a graph-based version for partial spheres, allowing us to compete at high-resolution with planar CNNs using residual network architectures. Our kernels operate on the tangent of the sphere and thus standard feature weights, pretrained on perspective data, can be transferred, enabling spherical pretraining on ImageNet. As our design is free of distortions and discontinuity, our orientation-aware CNN becomes a new state of the art for semantic segmentation on the recent 2D3DS dataset, and the omni-directional version of SYNTHIA introduced in this work. Moreover, we experimentally show the benefit of our spherical representation over standard images on the Cityscapes dataset by reducing distortion effects of planar CNNs. We implement object detection for the spherical domain. Rotation invariant classification and segmentation tasks are additionally presented for comparison to prior art.
更多查看译文
关键词
Spherical deep learning,omni-directional cameras,semantic segmentation,object detection
AI 理解论文
溯源树
样例
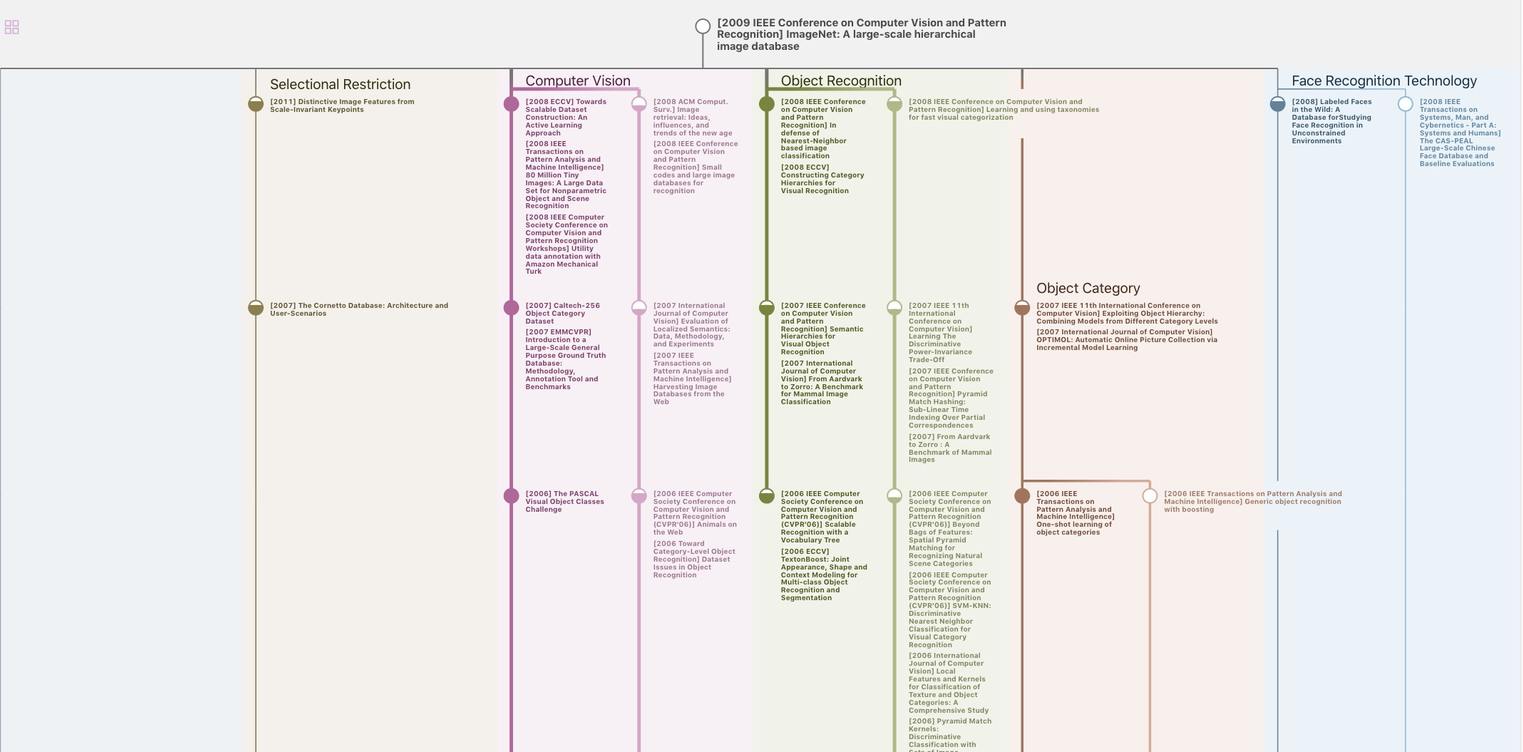
生成溯源树,研究论文发展脉络
Chat Paper
正在生成论文摘要