Devising and Detecting Phishing: large language models vs. Smaller Human Models
CoRR(2023)
摘要
AI programs, built using large language models, make it possible to automatically create phishing emails based on a few data points about a user. They stand in contrast to traditional phishing emails that hackers manually design using general rules gleaned from experience. The V-Triad is an advanced set of rules for manually designing phishing emails to exploit our cognitive heuristics and biases. In this study, we compare the performance of phishing emails created automatically by GPT-4 and manually using the V-Triad. We also combine GPT-4 with the V-Triad to assess their combined potential. A fourth group, exposed to generic phishing emails, was our control group. We utilized a factorial approach, sending emails to 112 randomly selected participants recruited for the study. The control group emails received a click-through rate between 19-28%, the GPT-generated emails 30-44%, emails generated by the V-Triad 69-79%, and emails generated by GPT and the V-Triad 43-81%. Each participant was asked to explain for why they pressed or did not press a link in the email. These answers often contradict each other, highlighting the need for personalized content. The cues that make one person avoid phishing emails make another person fall for them. Next, we used four popular large language models (GPT, Claude, PaLM, and LLaMA) to detect the intention of phishing emails and compare the results to human detection. The language models demonstrated a strong ability to detect malicious intent, even in non-obvious phishing emails. They sometimes surpassed human detection, although often being slightly less accurate than humans.
更多查看译文
关键词
detecting phishing,large language models
AI 理解论文
溯源树
样例
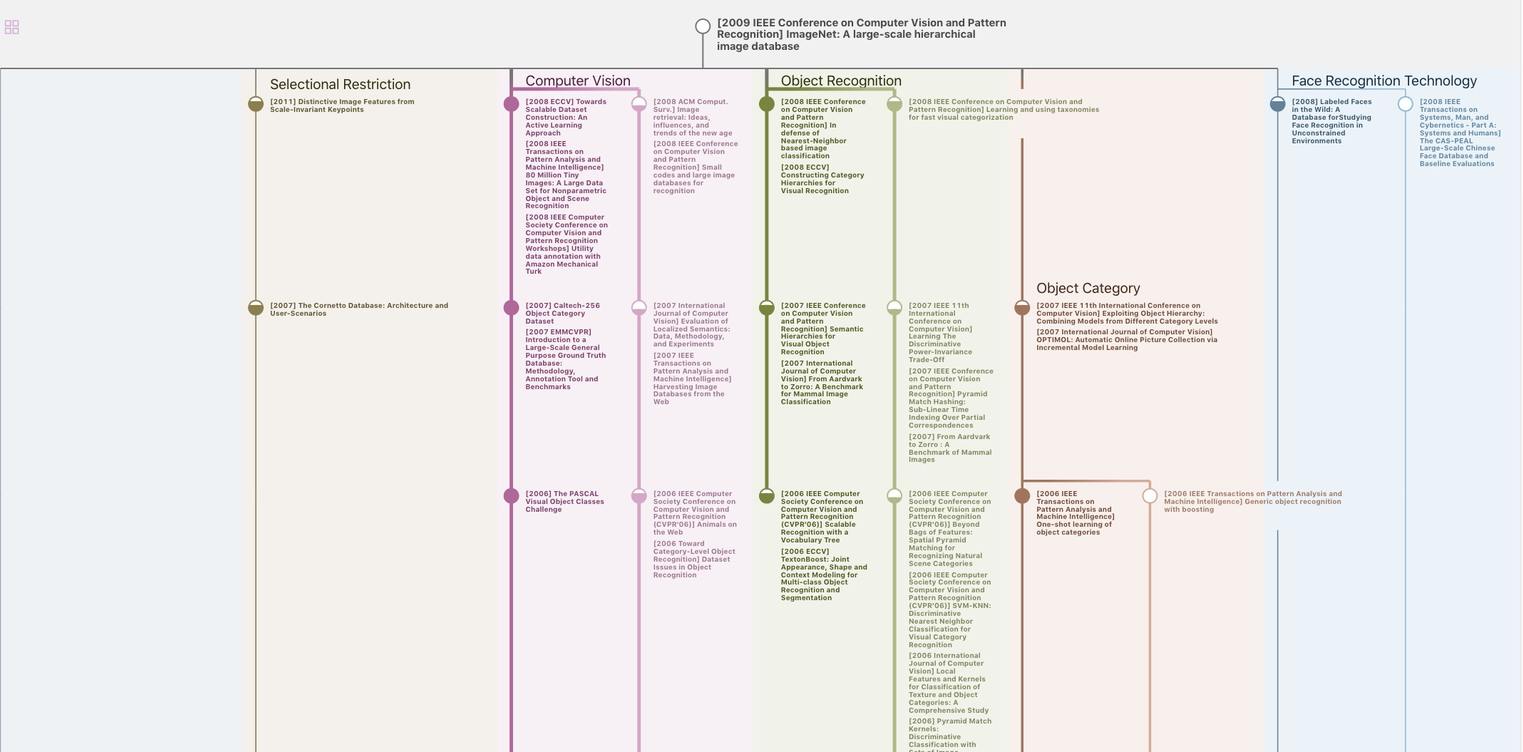
生成溯源树,研究论文发展脉络
Chat Paper
正在生成论文摘要