Pose Modulated Avatars from Video
arXiv (Cornell University)(2023)
摘要
It is now possible to reconstruct dynamic human motion and shape from a sparse set of cameras using Neural Radiance Fields (NeRF) driven by an underlying skeleton. However, a challenge remains to model the deformation of cloth and skin in relation to skeleton pose. Unlike existing avatar models that are learned implicitly or rely on a proxy surface, our approach is motivated by the observation that different poses necessitate unique frequency assignments. Neglecting this distinction yields noisy artifacts in smooth areas or blurs fine-grained texture and shape details in sharp regions. We develop a two-branch neural network that is adaptive and explicit in the frequency domain. The first branch is a graph neural network that models correlations among body parts locally, taking skeleton pose as input. The second branch combines these correlation features to a set of global frequencies and then modulates the feature encoding. Our experiments demonstrate that our network outperforms state-of-the-art methods in terms of preserving details and generalization capabilities.
更多查看译文
关键词
modulated avatars,video
AI 理解论文
溯源树
样例
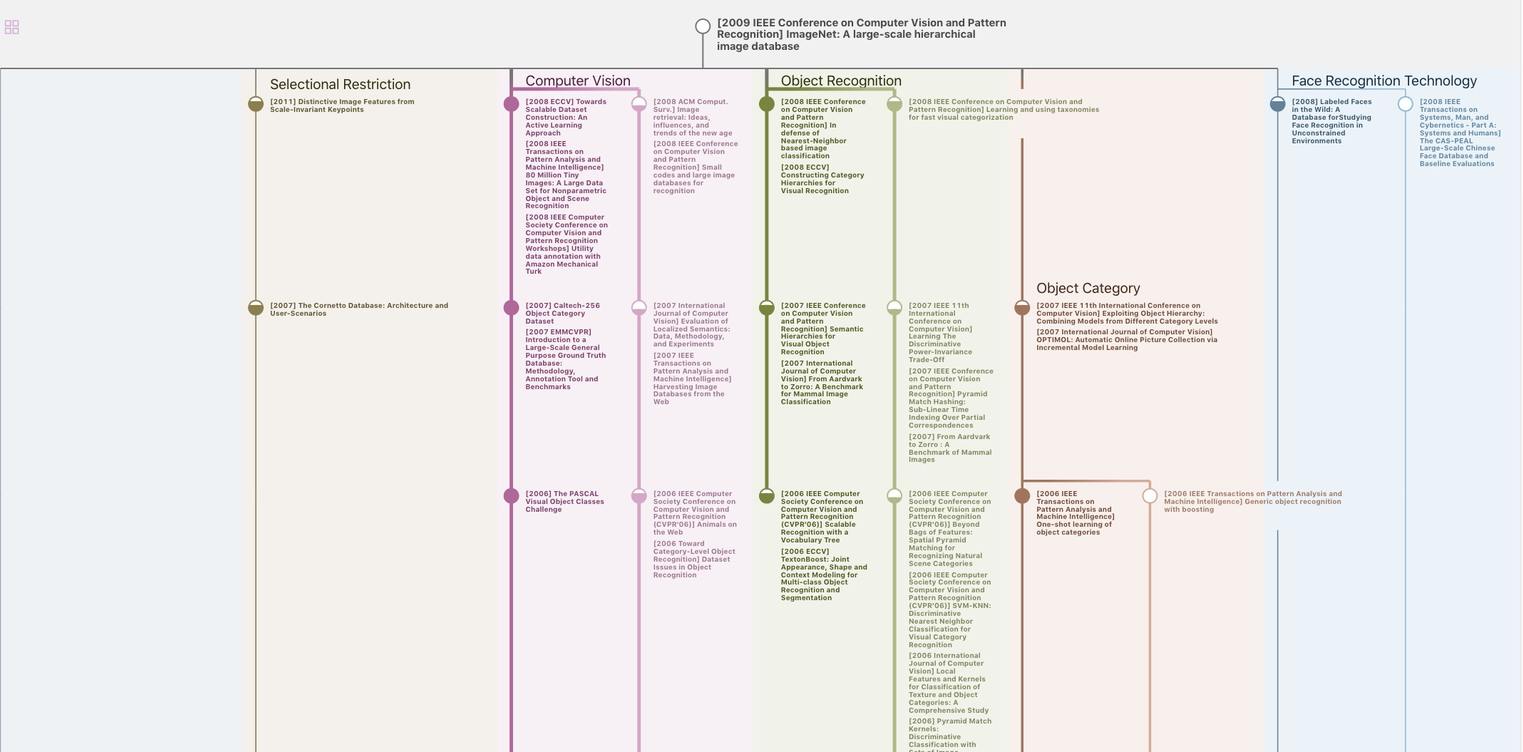
生成溯源树,研究论文发展脉络
Chat Paper
正在生成论文摘要