Studying the Impact of Augmentations on Medical Confidence Calibration
2023 IEEE/CVF INTERNATIONAL CONFERENCE ON COMPUTER VISION WORKSHOPS, ICCVW(2023)
摘要
The clinical explainability of convolutional neural networks (CNN) heavily relies on the joint interpretation of a model's predicted diagnostic label and associated confidence. A highly certain or uncertain model can significantly impact clinical decision-making. Thus, ensuring that confidence estimates reflect the true correctness likelihood for a prediction is essential. CNNs are often poorly calibrated and prone to overconfidence leading to improper measures of uncertainty. This creates the need for confidence calibration. However, accuracy and performance-based evaluations of CNNs are commonly used as the sole benchmark for medical tasks. Taking into consideration the risks associated with miscalibration is of high importance. In recent years, modern augmentation techniques, which cut, mix, and combine images, have been introduced. Such augmentations have benefited CNNs through regularization, robustness to adversarial samples, and calibration. Standard augmentations based on image scaling, rotating, and zooming, are widely leveraged in the medical domain to combat the scarcity of data. In this paper, we evaluate the effects of three modern augmentation techniques, CutMix, MixUp, and CutOut on the calibration and performance of CNNs for medical tasks. CutMix improved calibration the most while CutOut often lowered the level of calibration.
更多查看译文
关键词
Medical Image Analysis,Confidence Calibration,Modern Augmentations
AI 理解论文
溯源树
样例
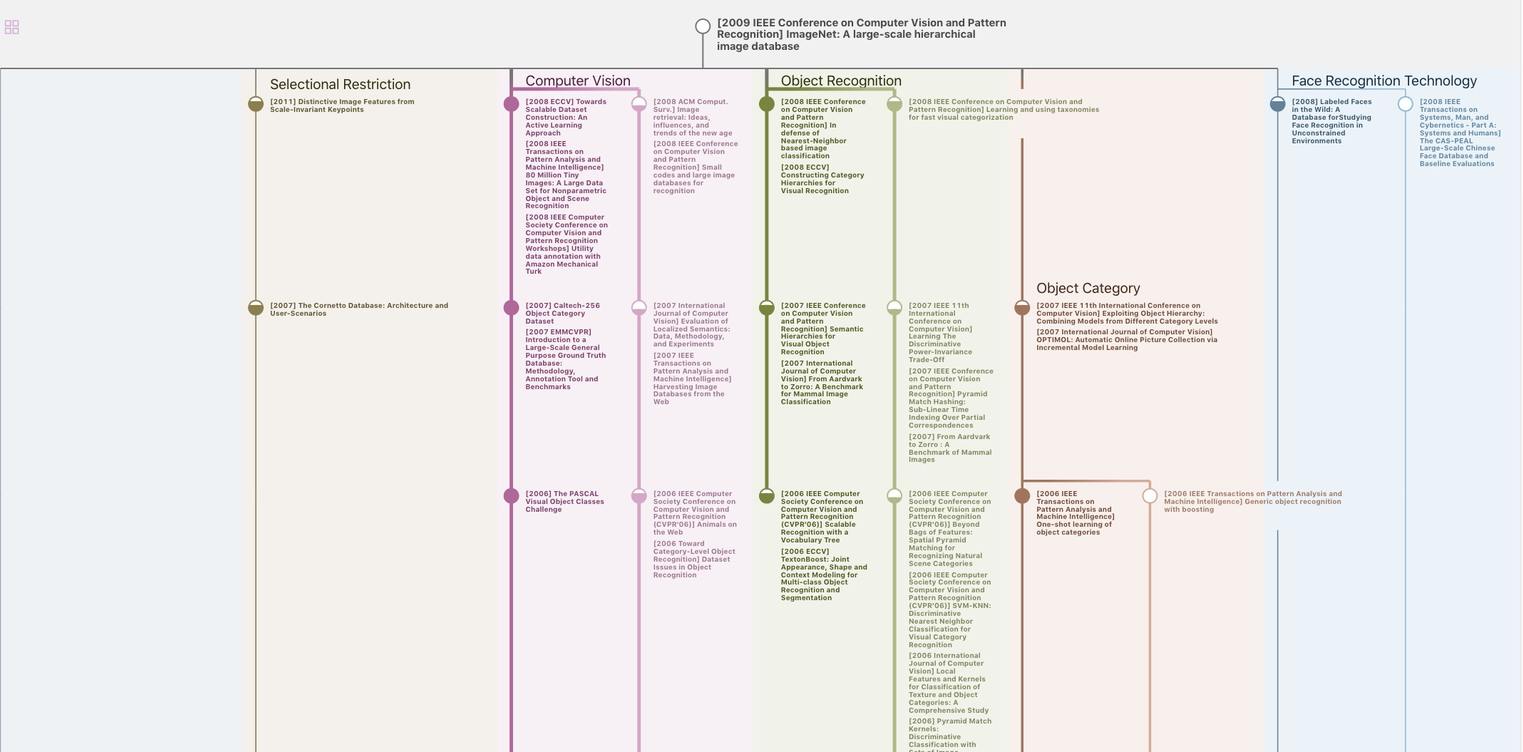
生成溯源树,研究论文发展脉络
Chat Paper
正在生成论文摘要