Adversarial Training Using Feedback Loops
CoRR(2023)
摘要
Deep neural networks (DNN) have found wide applicability in numerous fields due to their ability to accurately learn very complex input-output relations. Despite their accuracy and extensive use, DNNs are highly susceptible to adversarial attacks due to limited generalizability. For future progress in the field, it is essential to build DNNs that are robust to any kind of perturbations to the data points. In the past, many techniques have been proposed to robustify DNNs using first-order derivative information of the network. This paper proposes a new robustification approach based on control theory. A neural network architecture that incorporates feedback control, named Feedback Neural Networks, is proposed. The controller is itself a neural network, which is trained using regular and adversarial data such as to stabilize the system outputs. The novel adversarial training approach based on the feedback control architecture is called Feedback Looped Adversarial Training (FLAT). Numerical results on standard test problems empirically show that our FLAT method is more effective than the state-of-the-art to guard against adversarial attacks.
更多查看译文
关键词
adversarial training,feedback
AI 理解论文
溯源树
样例
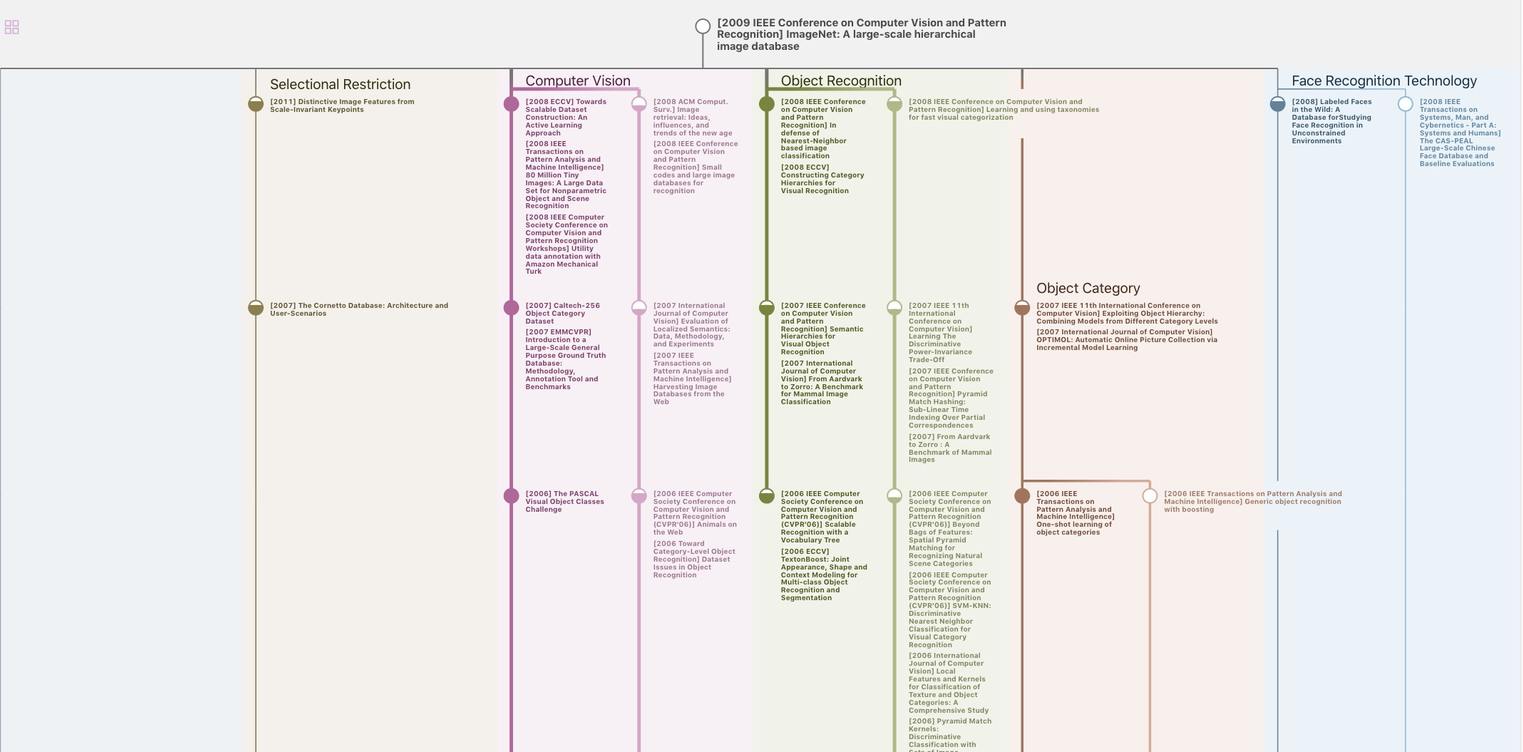
生成溯源树,研究论文发展脉络
Chat Paper
正在生成论文摘要