Speech emotion recognition using MFCC-based entropy feature
SIGNAL IMAGE AND VIDEO PROCESSING(2023)
摘要
The prime objective of speech emotion recognition is to accurately recognize the emotion from the speech signal. It is a challenging task to accomplish. Speech emotion recognition (SER) has many applications, including medicine, online marketing, strengthening human-computer interaction (HCI), online education, and many more. Hence, it has been a topic of interest for many researchers for last three decades. The researchers used different methodologies to improve the classification accuracy of emotions. In this study, we tried to improve emotion classification accuracy using mel-frequency cepstral coefficient (MFCC)-based entropy features. First, we extracted the MFCC coefficient matrix from every speech of the EMO-DB, RAVDESS and SAVEE datasets, and then we calculated the proposed features: statistical mean (MFCCmean), MFCC-based approximate entropy (MFCCAE), and MFCC-based spectral entropy (MFCCSE), from the MFCC coefficient matrix of every utterance. The performance of the proposed features is accessed using the DNN classifier. We achieved a classification accuracy of 87.48%, 75.9%, and 79.64% using the combination of MFCCmean and MFCCSE features and obtained classification accuracies of 85.61%, 77.54%, and 76.26% using the combination of MFCCmean, MFCCAE, and MFCCSE features for the EMO-DB, RAVDESS, and SAVEE datasets, respectively.
更多查看译文
关键词
Deep neural network,Speech emotion recognition,MFCC,Spectral entropy,Approximate entropy
AI 理解论文
溯源树
样例
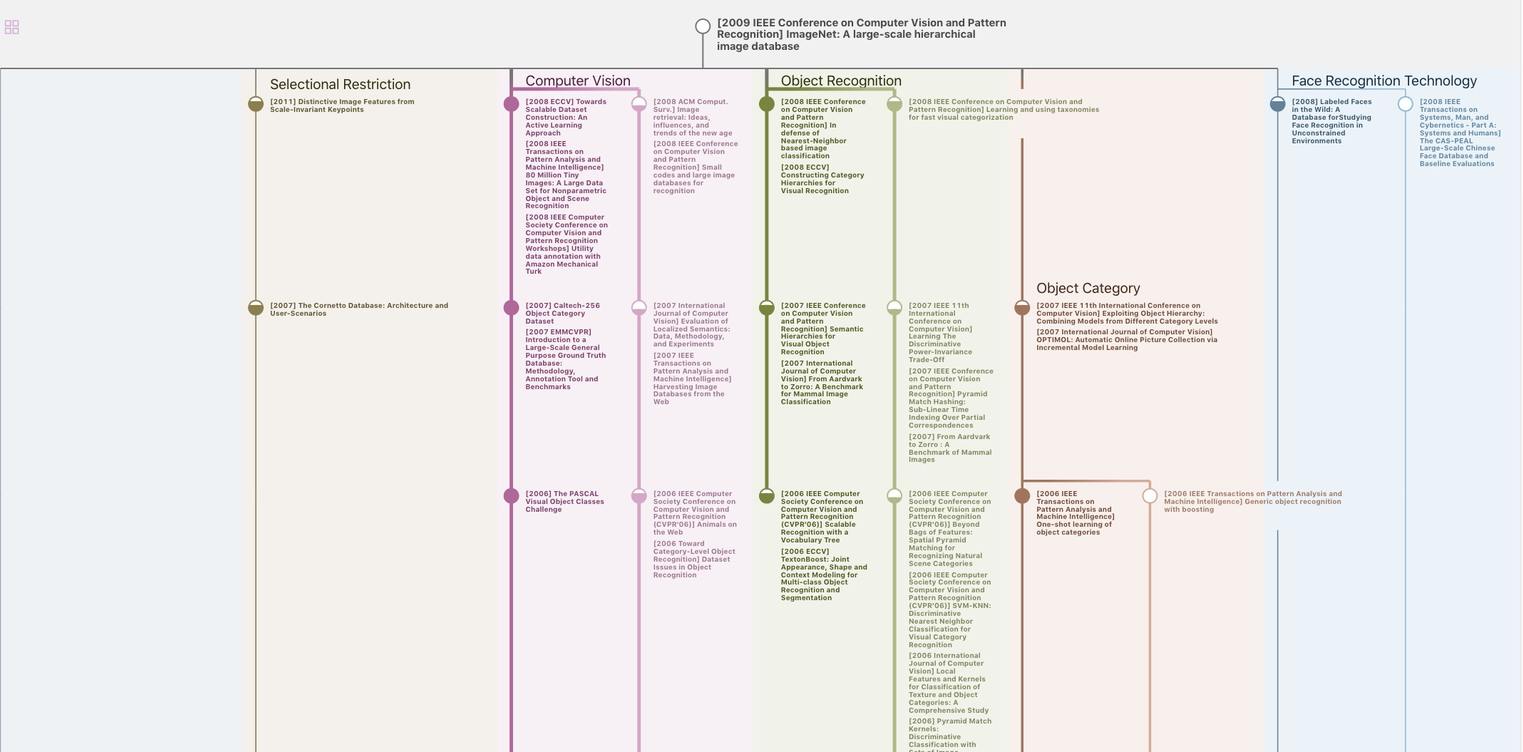
生成溯源树,研究论文发展脉络
Chat Paper
正在生成论文摘要