Improving DNA 6mA Site Prediction via Integrating Bidirectional Long Short-Term Memory, Convolutional Neural Network, and Self-Attention Mechanism
Journal of chemical information and modeling(2023)
摘要
Identifying DNA N6-methyladenine (6mA) sites is significantlyimportantto understanding the function of DNA. Many deep learning-based methodshave been developed to improve the performance of 6mA site prediction.In this study, to further improve the performance of 6mA site prediction,we propose a new meta method, called Co6mA, to integrate bidirectionallong short-term memory (BiLSTM), convolutional neural networks (CNNs),and self-attention mechanisms (SAM) via assembling two different deeplearning-based models. The first model developed in this study iscalled CBi6mA, which is composed of CNN, BiLSTM, and fully connectedmodules. The second model is borrowed from LA6mA, which is an existing6mA prediction method based on BiLSTM and SAM modules. Experimentalresults on two independent testing sets of different model organisms,i.e., Arabidopsis thaliana and Drosophilamelanogaster, demonstrate that Co6mA can achieve an averageaccuracy of 91.8%, covering 89% of all 6mA samples while achievingan average Matthews correlation coefficient value (0.839), which ishigher than the second-best method DeepM6A.
更多查看译文
关键词
dna,memory,site prediction,short-term,self-attention
AI 理解论文
溯源树
样例
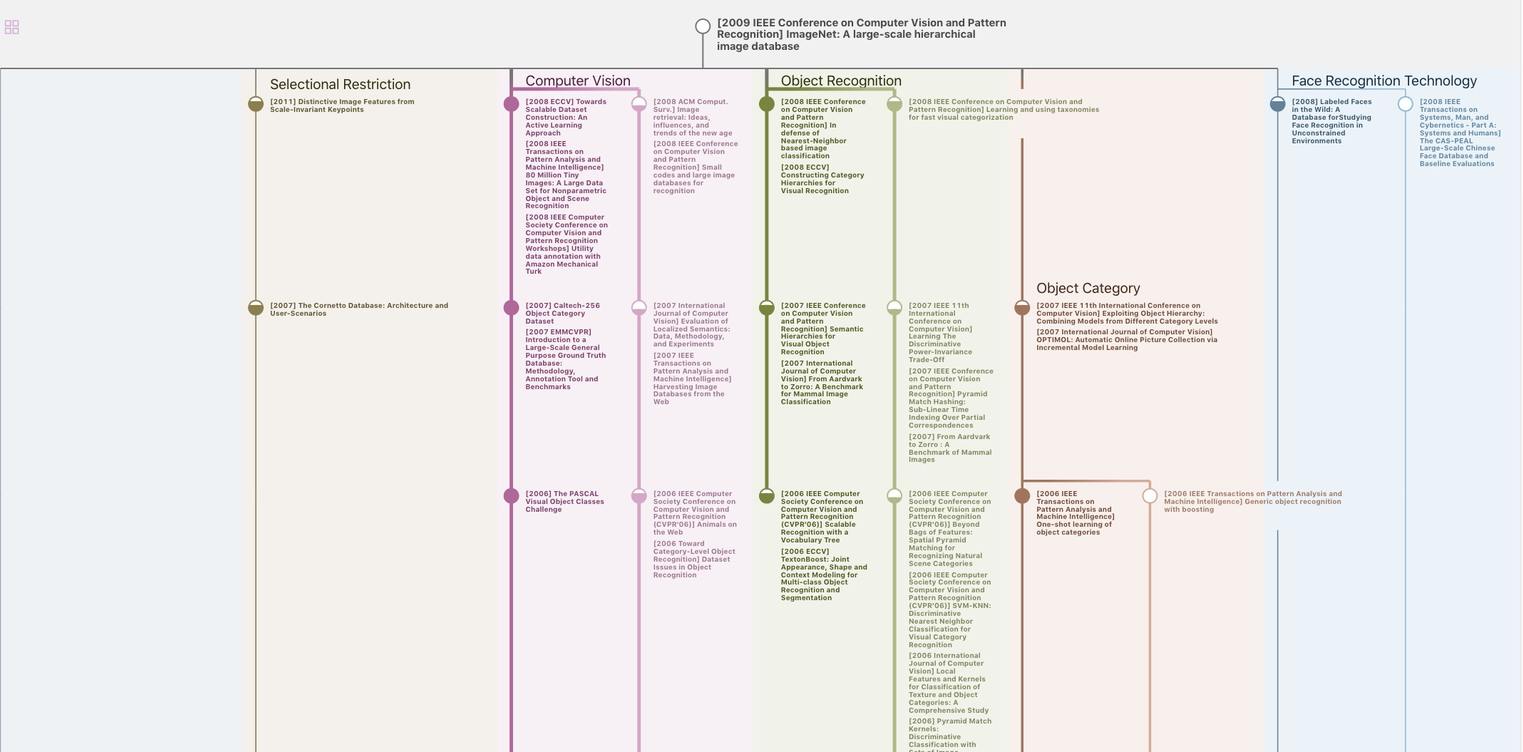
生成溯源树,研究论文发展脉络
Chat Paper
正在生成论文摘要