Delving into Motion-Aware Matching for Monocular 3D Object Tracking
Proceedings of the IEEE/CVF International Conference on Computer Vision (ICCV)(2023)
摘要
Recent advances of monocular 3D object detection facilitate the 3D multi-object tracking task based on low-cost camera sensors. In this paper, we find that the motion cue of objects along different time frames is critical in 3D multi-object tracking, which is less explored in existing monocular-based approaches. In this paper, we propose a motion-aware framework for monocular 3D MOT. To this end, we propose MoMA-M3T, a framework that mainly consists of three motion-aware components. First, we represent the possible movement of an object related to all object tracklets in the feature space as its motion features. Then, we further model the historical object tracklet along the time frame in a spatial-temporal perspective via a motion transformer. Finally, we propose a motion-aware matching module to associate historical object tracklets and current observations as final tracking results. We conduct extensive experiments on the nuScenes and KITTI datasets to demonstrate that our MoMA-M3T achieves competitive performance against state-of-the-art methods. Moreover, the proposed tracker is flexible and can be easily plugged into existing image-based 3D object detectors without re-training. Code and models are available at https://github.com/kuanchihhuang/MoMA-M3T.
更多查看译文
关键词
monocular 3d object,motion-aware
AI 理解论文
溯源树
样例
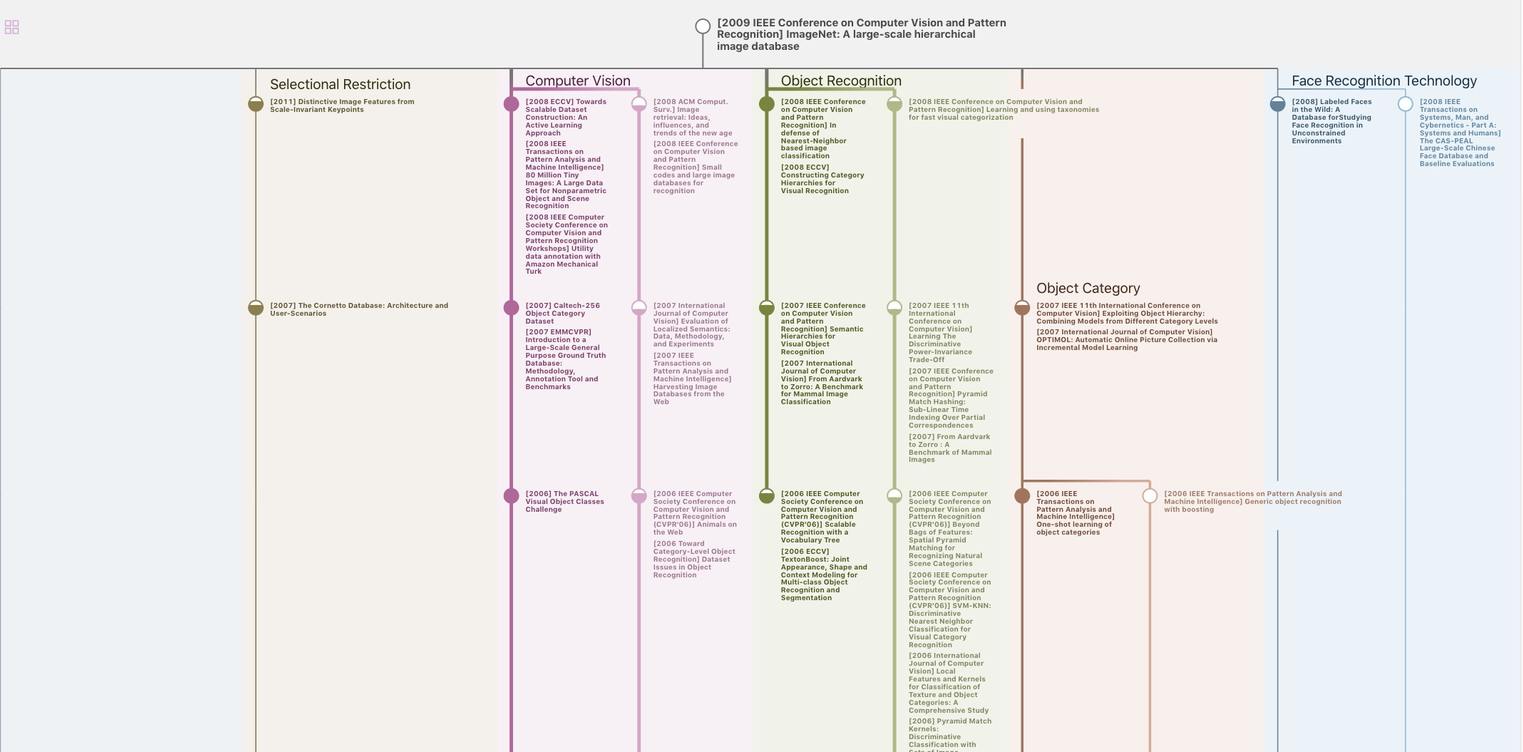
生成溯源树,研究论文发展脉络
Chat Paper
正在生成论文摘要